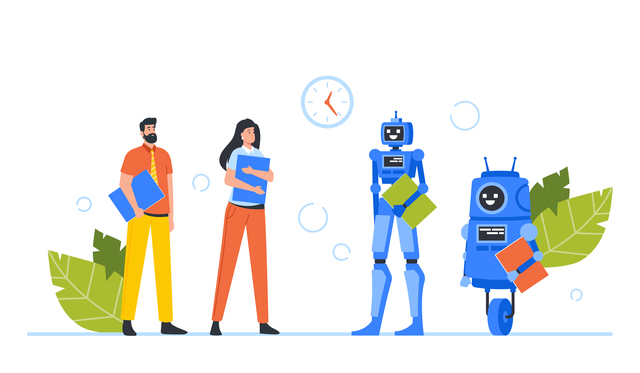
Machine translation has come a long way in the decades since it was first researched. With every great development in computing and technology, machine translation has grown in its scope and capabilities, producing ever more accurate translations for an ever increasing list of language pairs. But every generation of new tech has is limits, and what do we do when machine translation has no more room to grow?
A Short History of Machine Translation
In its early stages, with experiments starting as early as the 1950s, machine translation was extremely promising in concept but lacking in execution, as the technology of the times simply couldn’t account for all of the discrepancies, rule exceptions, and contextual variance that exist between languages. For decades, machine translation was essentially a word-for-word replacement from one language into another, according to its encoded dictionaries and grammar rulesets. While helpful at providing a simple understanding of the original text in the target language, it often resulted in stilted and nonsensical sentences that failed to address context or natural word progression, and it inevitably required a human translator to translate the text in full.
The lack of utility, despite the costly investment, initially dissuaded further research and development of these systems, save for a short list of language pairs and restricted inputs. But with improvements to computer processing speeds and mainframe technology, as well a surge of commercial demand to meet the growth of globalization, the use of machine translation began to spread through the 70s and 80s, and new methods were researched, leading to the development of statistical and example-based machine translations. The basis of these methods was manipulating the text corpora, instead of trying to code entire sets of linguistic rules and dictionaries into the system, resulting in translations that are more natural and less memory intensive than their purely rules-based MT counterparts.
By the 1990s, thanks in large part to the development of both less expensive and more powerful computers, machine translation became not only a corporate and government tool, but a service available to the public through PC software and Internet websites. However, the quality of these services was still far removed from that of a human translator, and the resulting text had nowhere near the accuracy or legibility to reliably use in professional releases without extensive edits.
Finally, around 2015, another breakthrough in MT technology occurred with the development and application of neural machine learning. Thanks to the incorporation of artificial intelligence, inspired by the application of deep learning to the closely related field of speech recognition technology, the fluency of machine translations improved drastically, despite using only a fraction of the memory needed for statistical MT, which had become the leading MT method up to that point. Neural MT engines developed by major tech companies like Google, Microsoft, and others became capable of identifying context and arranging words into natural patterns based on enormous amounts of machine-led information analysis, and the accuracy and usability of MT results spiked.
Modern Implementations of Neural Machine Translation
In the past few years, an increasing number of tech companies—including many not originally associated with language services, like Amazon, Facebook, and Apple—have identified the many benefits and applications of neural machine translation and have developed their own NMT engines, freely available to the public in some capacity. And the quality of these engines has progressed at an alarming rate for an increasing breadth of language pairs.
The dedicated translation engine for Facebook was developed for multilingual translation of the many user-created posts that appear on their social networking service. The Translate app by Apple was developed for use on their iOS and iPadOS devices. Such services exist to extend the reach and appeal of the companies’ primary enterprises. Google Translate and Microsoft Translator were similarly developed in support of the Internet browsers and search engines that are central pillars of these companies, but the translation engines themselves have become independent services utilized by individuals and corporate entities, alike.
Based on the available options listed by many of the largest language service providers (LSPs) who offer machine translation as a service, Google Translate, Microsoft Translator, and the independently developed DeepL Translator, are the three most reliable NMT engines on the current market. In a very short amount of time, ever since the application of deep learning to MT, these engines have improved drastically across hundreds of language pairs. LSPs including Human Science have put a lot of time and resources into measuring the relative strengths of each engine and ranking their utility as both a tool and an independent service. But, in contrast to the steep rise in growth that we witnessed for the first five years of utilization, these NMT engines have reached a sort of equilibrium.
According to the observations of MT researchers at Lionbridge, another language service provider based in the USA, the improvements being made to generic neural machine translation engines across many platforms have slowed to a trickle. And for many of the most common languages, the quality of the translations has more-or-less equalized across all the top engines. Certain engines may still demonstrate unique quirks or particular strengths in certain languages, but for the most part, it seems that no matter which translation engine you select, the quality of the results will be about the same. And by their calculations, that quality is unlikely to improve much further without another drastic breakthrough in computing.
But what does that mean for companies hoping to utilize machine translation in their future endeavors? How can they hope to build beyond this plateau and ensure the efficacy of their investment in NMT?
The Benefits of Customization
Thankfully, even if the generic MT engines that are publicly available have approached their limits, a great deal of improvement can still be gained through customization.
Already, when considering the introduction of machine translation to your business from an information security perspective, there is a clear benefit to using an isolated machine translation platform, so that your translation data is not co-opted to train the AI of a generic NMT engine, which may be considered an information security leak. As an added benefit, having an isolated platform also allows for personalized machine teaching and other customizations of the MT engine, which result in more accurate and consistent MT outputs that further improve according to the corrections that you register within your system.
To be more specific, the first aspect of MT customization that comes with an isolated MT platform is a personalized pool of materials from which the translation engine is able to learn. Instead of referencing and drawing correlations from the full breadth of knowledge that gets fed into generic translation engines and is impossible to control, by isolating the MT engine that your company uses, the engine receives a curated assortment of translation materials that are tailored to the business that your company conducts and the style of writing that is most appropriate for your documents. Whether this information is fed into the core system in advance through annotation or built up over time through the AI teaching that occurs in conjunction with the post editing process to correct the results of newly translated documents, the MT engine learns to correlate the appropriate words and phrases that appear consistently within your documents and provide more suitable results as time goes on.
The second way that MT customization can be applied is syntax-related rule setting. While neural machine translation and its predecessor, statistical machine translation, do not technically rely on linguistic rulesets to function, there are tools and add-ons that can automatically correct some stylistic issues and inconsistencies that appear in the results of generic ME engines. MTrans, an MT customization tool developed by Human Science, for example, has an automatic style editing function that can ensure stylistic consistency in ways that are easy for a human editor to overlook. The types of automatic corrections that it handles include spacing issues around certain symbols, inconsistency in the shape or width of quotation marks and other characters, the improper utilization of contractions and other words or phrases that may be too casual for the type of document being translated, and so on. The style rules must be registered manually at the start, but as with all MT training, once the rules are in place, they are automatically applied to the translation results, ensuring higher stylistic consistency in the MT results.
The third type of MT customization that is available is the incorporation of a glossary. The benefits and limitations of creating glossaries for translation work were explained in depth in a previous blog post, What Terms to Include in a Translation Glossary, but in short, the purpose of such a glossary is to promote appropriate and consistent terminology in a body of translated works. Translation glossaries are especially useful when handling documents that contain UI (user interface) terms or proper nouns. And when using a custom MT engine, unlike the generic MT engines available for free, individualized translation glossaries may be applicable. For machine translation in particular, these glossaries are most effective when they don’t contain any verbs or other terms that may require conjugation in the target language. (Recall that modern NMT engines do not actually function according to grammar rules and are also incapable of inferring context across multiple sentences.) But for the accurate translation of things like product names, organizations, titles of existing documents, on-screen labels and messages, and other static language that must not vary, the use of a translation glossary is extremely valuable.
By utilizing these three aspects of MT customization—individualized machine training, rule setting, and glossary setting—NMT can be an increasingly economical source of reliable translations, despite the slowing pace at which the engines, themselves, are improving. This is not to say that human involvement could become unnecessary to the translation process. In the same way that an editor is necessary to the human translation process, machine translations still require a post-editor to confirm the accuracy and stylistic quality of the translated results, and preparatory steps like training the AI and managing the custom rules and glossaries also require manual updates by human administrators.
The difference in using an MT engine over relying on a human translator is that with just a bit of routine maintenance (some of which might be necessary regardless, in the case of translation glossaries), a machine translation engine is able to handle the translation process much faster than a human translator, and for a much larger volume of materials. And based on the time and money in the budget, determinations can be made on whether to sacrifice quality for speed and affordability. Therefore, by applying customization to the machine translation engine that is used, the quality of even the baseline MT results for all documents is raised compared to the results of a general engine. So even as the pace of improvement of NMT in general slows, the rates of accuracy and conformity of the MT translations in your library of documents can continue to grow.
There is no need to wait for the next technological breakthrough to establish worthwhile MT.
関連サービス
AI自動翻訳ソフトMTrans Team
執筆者情報
-
Rachel TackettMultilingual Translation Group
JP→EN Translation Reviewer- ・Before joining Human Science, Rachel worked for two years as an English teaching assistant, and then another two years as a writer and translator of Asian culture and entertainment news.
- ・She has since amassed over six years of experience in technical translation and the production of technical manuals.
- ・She is currently engaged in Japanese to English translation and quality control for technical documents such as product manuals, help pages, and work manuals for SaaS and other big industries.
- ・She is also in charge of quality assessment and verification for Japanese to English translations by machine translation engines.