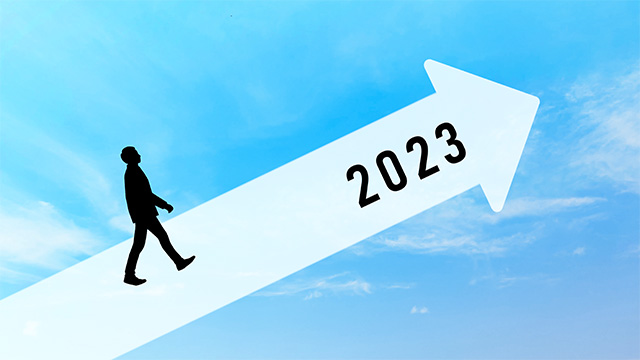
In September 2020, on our blog "What is the translation accuracy of DeepL in medical translation? Verification results of CIOMS, ICF, IB, etc. (https://www.science.co.jp/nmt/blog/21613/)", we conducted machine translation using DeepL and Google for various medical documents and evaluated and verified them through automatic evaluation BLEU scores and human assessment.
We will again describe the evaluation methods used in the previous verification.
Language Pair: English → Japanese
Target documents: six types including white papers, manuals (medical devices), CIOMS, ICF (informed consent documents), IB (investigational drug brochures), and papers
Evaluation volume: Approximately 1,000 words for each type (around 50 sentences for each type)
Evaluation Criteria: Automatic Evaluation BLEU Score and Human Evaluation
Now, two and a half years later (March 2023), we conducted a translation of the exact same text using DeepL and Google Translate to examine what changes have occurred. This article presents the results.
- Table of Contents
Some improvements at the technical terminology level
In medical documents, there are unique specialized terms. When these are translated into common terms, it results in typical (and potentially fatal) mistranslations. Additionally, in my opinion, not limited to the medical field, there may also be a tendency for machine translation to convert common terms into IT-related terminology.
In this translation, improvements were observed in those areas.
Improvements at the level of technical terminology were relatively often seen in CIOMS's Google Translate.
Case correction
"Correction of uppercase and lowercase letters" becomes "Case correction"
Outcome is Unknown
"The result is unknown" becomes "Outcome is unknown"
Attend
"Participate" becomes "Attended"
By the way, these were correctly translated by DeepL as of 2020.
Examples of improvements at the term level in Google Translate for documents other than CIOMS are as follows.
White Paper
highlighted within the analysis
In 2020, Google referred to it as "highlighted," but in 2023 it is referred to as "emphasized."
ICF
Monotherapy
In 2020, it was "monotherapy" at Google, but in 2023 it is "monotherapy"
The above white papers and ICF terminology were accurately translated by DeepL as of 2020. The overall higher evaluation of DeepL in the human assessment of 2020 may have been influenced by the relatively accurate translation of such specialized terms.
However, there are also areas that have not been improved
Let's take a look at the CIOMS translation as well.
Seriousness: serious regarding severity was incorrectly translated as "seriousness: serious" by Google in 2020 and as "severity: serious" by DeepL.
Even in 2023, Google translated it as "Severity: Severe" and DeepL as "Significance: Major", neither of which were correct.
The term "Narrative," which can be translated as case description or case progression, was translated as "story" by Google in both 2020 and 2023 (while DeepL translated it as "narrative" in both years). Additionally, "Listedness: unlisted" was translated by Google as "listed: unlisted" in both 2020 and 2023 (while DeepL translated it as "publishability: unpublished" in 2020 and "Listedness: unlisted" in 2023).
In this way, while improvements in the translation of technical terms can be confirmed to some extent, it is considered to be limited in nature.
By the way, when comparing the documents from 2020 and 2023, there are quite a number of changes. Taking the translation of the white paper by DeepL as an example, there were 182 changes in a document of over 1600 characters (based on the number of changes calculated by the Word document comparison feature).
However, the main changes seemed to be mostly related to the order of the segments and other aspects that do not significantly affect the overall meaning, as shown below.
"Investigated" → "Considered"
"And" → "And"
"Induced" → "Guided"
"Regarding the use of" → "Regarding the usage of",
These changes may not matter as long as the meaning is understood, especially in documents intended for internal readers. For example, whether "investigated" or "considered" is more appropriate, or if there is another suitable translation, depends on the context and background. In the future, it is unclear whether improvements to this level can be achieved with advancements in machine translation, and it can be said that this is precisely an area that should be properly handled in the post-editing process.
As a result, the differences between 2020 and 2023 are primarily as mentioned above. While there have been improvements at the level of technical terminology, they have not reached the point of surpassing the previous translation evaluation scores, and it can be said that a rigorous improvement process through post-editing is still necessary.
From here on, I would like to discuss the details for each specific point.
Regarding style and terminology consistency
"Desu-masu style" and "dearu style":
In CIOMS, IB, and papers, "dearu style" is appropriate, while "desu-masu style" is suitable for ICF, white papers, and manuals. So, how did the machine translation results turn out?
In Google Translate, both in 2020 and 2023, the predominant style for all documents was the "desu-masu" form. There were only a few instances of the "dearu" form mixed in, which can be considered as errors or instances where the terminology in the text was coincidentally judged to be appropriate for the "dearu" form.
In DeepL, from 2020 to 2023, CIOMS used the "dearu" style (appropriate), IB mixed both "dearu" and "desu-masu" styles, ICF used the "desu-masu" style (appropriate), white papers used the "desu-masu" style (appropriate, although some mixed with "dearu"), manuals used the "desu-masu" style (appropriate), and papers used the "dearu" style (appropriate, although some mixed with "desu-masu").
Naturally, the unification to either the "dearu style" or the "desu-masu style" will be done during the post-editing process. By the way, in the human evaluation of 2020, the "dearu style" and "desu-masu style" were excluded from the evaluation materials for verification.
Half-width spaces and half-width brackets:
It is unclear whether it is a coincidence or a decision made by the developers, but in Google Translate in 2023, half-width spaces were added before and after alphanumeric characters, and parentheses and colons were also in half-width. However, there were a few instances where there were no half-width spaces, which may be an error.
Terminology Consistency: A Comparison Between 2020 and 2023, Is It Unified Within the Document?
Typical terms that we want to standardize in medical translation include "cancer" and "malignant tumor".
Upon checking the IB where the term "Cancer" was mentioned six times in the original text, the following results were found.
Google Translate 2020 "cancer" 2 cases, "cancer" 4 cases → 2023 "cancer" 4 cases, "cancer" 2 cases
DeepL 2020 "cancer" 5 cases, "cancer" 0 cases → 2023 "cancer" 5 cases, "cancer" 0 cases (Note: The remaining one instance was in the organization name as cancer, so it was left as is)
Additionally, the term "signature (molecular signature, translated as [molecular signature] or [molecular signature])" appeared three times in the white paper, so I also investigated whether it was translated as "signature," "signature," or "signature."
Google Translate 2020 "Signature" 0 results, "Sign" 1 result, "Signature" 2 results → 2023 "Signature" 0 results, "Sign" 2 results, "Signature" 1 result
DeepL 2020 "Signature" 1 result, "Sign" 2 results → 2023 "Signature" 2 results, "Sign" 0 results, "Signature" 1 result
The unification of terminology and notation is one of the most important items that should originally be addressed in post-editing. Even though this verification was limited in scope, there was no unification of terminology and notation except for the unification of "cancer" in DeepL.
What are sentences that are not valid?
Although there were only a few, there were some translations where the text was not coherent.
Common characteristics include a high word count (generally exceeding 40 words in a single sentence) and the presence of multiple parentheses or slashes within the text. An example of such a sentence is as follows.
The optimized molecular HRD signature from Study AAA (BBB) was prospectively applied to the primary analysis of Study CCC (DDD), an ongoing, randomized, double-blind, Phase 3 study of eee versus placebo as switch maintenance treatment in patients with platinum-sensitive, relapsed, high-grade ovarian cancer (n = fff enrolled patients).
Note: AAA (BBB), CCC (DDD) are test names. eee is the drug name. fff is the value (number of patients)
Such sentences are the type that always appear in clinical trial-related documents and papers. They can also be said to be sentences that are not easily familiar with "pre-editing," which involves editing the original text in advance to facilitate machine translation.
Google Translate was unable to produce a coherent translation for the text in both 2020 and 2023.
While DeepL was able to produce coherent sentences, in cases like this, whether through post-editing or human translation, it is necessary for a person to analyze the original text and consider the context and background.
Additionally, if there are similar existing translations that can be reused, using translation memory with translation support tools may be a strong option rather than creating new translations each time.
Summary
When comparing the same text in 2020 and 2023 using machine translation (Google and DeepL), there were some changes. Specifically, there were several instances where critical mistranslations at the level of technical terms were improved, but overall, it was not as remarkable as expected.
It goes without saying that the technology related to AI, including machine translation, is in a situation where further significant advancements could happen at any time, as exemplified by Chat GPT. However, from the recent re-evaluation of machine translation, I got the impression that post-editing improvements are still essential at this stage. Additionally, for documents that are highly specialized and complex, such as those in the medical field, but still have a certain degree of regularity, utilizing translation support tools that leverage translation memory is also a strong option. Furthermore, there still seem to be many situations where completely human translation is effective.
We offer machine translation solutions and post-editing services that can unify the use of formal and informal language. If you have any concerns or interests, please feel free to contact us.
Machine Translation Solutions (MTrans for Phrase TMS/MTrans for Trados)
https://www.science.co.jp/nmt/service/memsource.html
https://www.science.co.jp/nmt/service/nmt.html
Post-editing Service
https://www.science.co.jp/nmt/service/postedit.html
Medical Translation Services
https://www.science.co.jp/localization/industry/medical/index.html