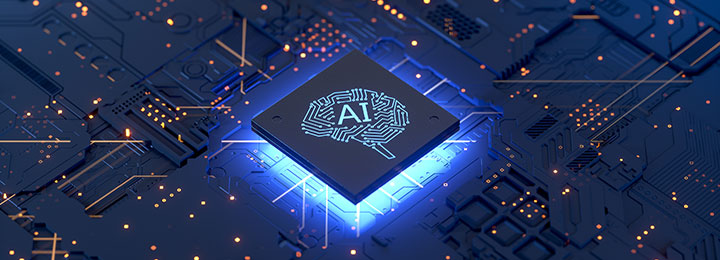
- Table of Contents
1. The Evolution of AI Translation
AI translation (machine translation by computers) first appeared in the 1950s. The technology used initially was rule-based, but statistical machine translation emerged in the late 1980s, followed by neural machine translation in the 2010s.
In rule-based machine translation, translation rules are manually created based on dictionaries and grammar. It was necessary to create a vast number of rules, which made development and updates for new terms labor-intensive. The accuracy was not high, and it could mainly translate only formulaic sentences.
In statistical machine translation, computers learn the rules instead of humans. They read a large number of pairs of original texts and their translations (such as one million sentences) and learn to statistically associate words and phrases from the original text and the translation based on that data (called a corpus). If additional original texts and translations are collected, it is relatively easy to accommodate new phrases. However, translation between languages with significantly different grammar, such as English and Japanese, is difficult, and the translation accuracy was not yet practical.
In addition, there are technologies such as hybrid machine translation that combines statistical machine translation and rule-based methods, as well as example-based machine translation that extracts similar parts from existing pairs of source texts and translations for use in translation. These machine translation methods have improved translation accuracy compared to rule-based and statistical machine translation.
Neural machine translation, like statistical machine translation, learns by feeding a large number of pairs of original texts and their translations to a computer. However, by using neural networks and deep learning, which are types of machine learning, it extracts and utilizes far more information for translation than statistical machine learning. Compared to traditional machine translation, the accuracy of translations has significantly improved. The translations are characterized by their fluency, producing natural translations that resemble human translations. With the advent of neural machine translation, machine translation has gained attention and is now widely used in everyday life and business.
2. Differences Between Traditional Machine Translation Technology and Latest Machine Translation Technology
The main difference between traditional automatic translation technologies, such as rule-based machine translation and statistical machine translation, and the latest automatic translation technology, neural machine translation, is the fluency of the translated text. Traditional machine translation often results in unnatural translations that are easily recognizable as machine-generated. Additionally, because translations are done sentence by sentence, the connections between sentences can often be awkward. In contrast, the translations produced by the latest neural machine translation are natural. When translating at the paragraph level, as with DeepL, the connections between sentences in the translated text are natural, making it difficult to distinguish whether the text was translated by a human or by machine translation when reading through the paragraph.
On the other hand, there are issues that have become prominent with neural machine translation. These include translation duplication and omissions. In traditional machine translation, since phrases from the original text are translated one by one into the output, duplication and omissions rarely occur. However, in neural machine translation, there can be cases of duplicated translations or omissions. Particularly because the translations produced by neural machine translation are fluent, it can be difficult to identify omissions when reading only the translated text. To find omissions, it is necessary to compare the original text with the translated text.
3. Features of Popular Machine Translation Worldwide
Representative machine translation services include DeepL, Google, and Microsoft, all of which utilize neural machine translation technology. Among these, DeepL is particularly noteworthy. The characteristic of DeepL is its fluency. This fluency is achieved through paragraph translation. By translating text at the paragraph level, it accurately captures context and domain, resulting in not only the appropriate terminology being used compared to sentence-level translation, but also a more natural connection between sentences. As a result, the translated text becomes more fluent.
For the latest trends in machine translation and comparison results of DeepL, Google, Microsoft, and Amazon, please see the blog article below.
Latest Trends in Machine Translation and Comparison of DeepL and Google Translate
The Future of AI Translation Technology Evolution
The evolution of AI translation technology has accelerated with the advent of large language models (LLMs) such as OpenAI's ChatGPT. LLMs learn from vast amounts of text data, enabling natural language understanding and generation similar to that of humans. This allows for translations that go beyond simple language conversion, understanding context and nuances, and reflecting cultural backgrounds for natural translations. LLMs can flexibly adapt to the translation style and tone requested by users, providing appropriate translations across a wide range of contexts—from casual conversations to formal business documents, and even emotionally expressive content. For more details on the translation accuracy of LLMs, please refer to the blog article below.
What Is the Translation Accuracy of OpenAI's New Model GPT-4.1? A Comparison with DeepL!
However, AI translation technology also has an issue known as "hallucination." This phenomenon occurs when AI generates information that does not actually exist or outputs incorrect content as the translation result. It often happens especially when the context is unclear or when AI makes excessive guesses, so human review is still necessary for translations produced by AI. Post-editing (human correction) continues to play an important role in translations involving specialized content or subtle nuances.
Improving real-time capabilities is also one of the important advancements in AI translation. It has become possible to generate subtitles for foreign language audio in real time and translate them simultaneously. Many people may have experienced this in online meetings or seminars. Additionally, quantum computers hold the potential to dramatically enhance the processing power of AI translation. Compared to conventional computers, quantum computers can process vast amounts of data in parallel, accelerating AI machine learning and large-scale data analysis. As a result, AI will be able to perform translation tasks with even greater accuracy and efficiency.
5. Summary
AI translation began in the 1950s with rule-based methods, evolved to statistical translation in the late 1980s, and advanced to neural translation in the 2010s. Currently, DeepL, Google, and Microsoft are the leading providers, with DeepL especially offering natural translations at the paragraph level. With the advent of large language models (LLMs), translations that understand context and nuance have become possible; however, the issue of "hallucination" remains, and human review is still necessary. In the future, real-time capabilities and quantum computing may further improve accuracy.
Our company, Human Science, offers the automatic translation software "MTrans for Office," which utilizes translation engines such as DeepL, Google, Microsoft, and OpenAI. OpenAI not only handles translation but can also generate, rewrite, and proofread text depending on the prompts, supporting work efficiency and multilingual capabilities. MTrans for Office is also available with a 14-day free trial. Please feel free to contact us.
Features of MTrans for Office
① Unlimited number of translatable files and glossaries with a flat-rate plan
② Translate with one click from Office products!
③ Secure API connection for peace of mind
・For customers who want enhanced security, we also offer SSO and IP restrictions
④ Support in Japanese by Japanese Companies
・Response to security check sheets is also available
・Payment by bank transfer is available
MTrans for Office is an easy-to-use translation software for Office.