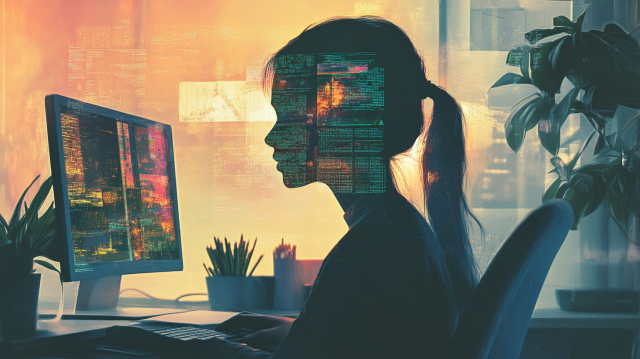
In its terms of service, ChatGPT forbids its use for a few select reasons. This list of prohibited actions starts with those that have obvious legal implications, like the furtherance of illegal acts, but also includes things like the unauthorized practice of law, unaided financial advice, and medical diagnosis or treatment. The reason why ChatGPT is not appropriate for such applications is because the system is not actually capable of making informed judgements based on situational variables, only of generating natural-sounding sentences on the topics of the prompts. It does not think and reason the way that humans do, but strings together words based on the probability of them appearing together. In fact, because the accuracy of the information presented is not actually a factor in the answers generated, such systems are known to “hallucinate” information that, if treated as fact and acted upon, could lead to severe harm.
Receiving bad information in the three fields listed above can have enormous impacts on people’s lives and livelihoods. So, to even insinuate that the automatically generated results of an AI system can be treated as actual advice in one of these fields without verification by a human specialist would be disingenuous and morally corrupt, in addition to being a violation of OpenAI’s ToS and in some cases illegal.
1. False Information and Hallucinations
Even without considering the specific fields of law, finance, and healthcare, one particularly harmful misconception about ChatGPT is the idea that it can be used like a detailed search engine to generate long-form factual responses to question prompts. Throughout the usage policies and terms of service presented by OpenAI, the creators of ChatGPT, they reiterate the fact that automatically generated output may not be accurate and should be independently verified.
ChatGPT and other automatic text generators do not actually have a fact checking function. Rather, they use predictive text patterns to create sentences that are statistically likely to occur around the topics at question, based on linguistic word associations that were trained into the systems from vast amounts of digital data. If the answer to your question is heavily documented and thus many examples of factual word associations have been imprinted on the database, then the text generated by the system may incidentally be true, but the accuracy of the information is in no way verified before output. And if the question you’re asking is very niche or requires expert knowledge, logic, or intuition, then the validity of the answer is even more unlikely, no matter how confidently the answer is worded.
Take the following exchange, based on a crossword clue by the New York Times:
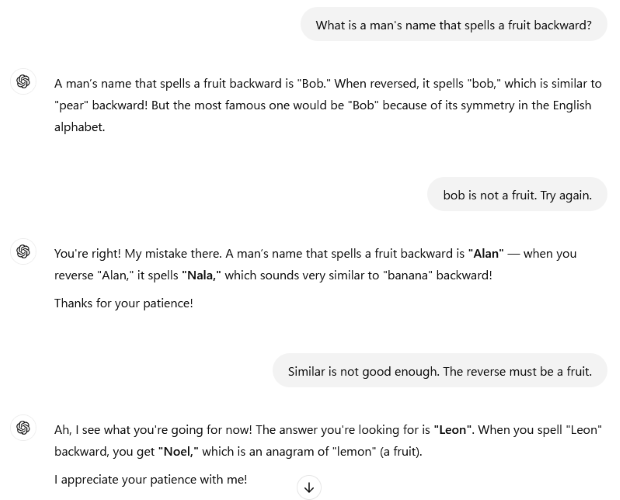
Clearly, ChatGPT cannot actually understand the intersection of these concepts and deduce the proper answer but will formulate responses in a format that replicates statement of fact. While the answers above contain a number of mistakes that are obvious to any person with a basic understanding of the English language, if a person has zero context for the answers that they seek and no inclination to independently verify the results generated by the system, they may easily become misled.
Even professionals asking questions in their field have been tricked by the confidence that ChatGPT mimics while presenting misinformation. In June of 2023, a pair of New York lawyers were sanctioned and made to pay a $5,000 fine for submitting a brief to the court that was written with the assistance of ChatGPT and contained multiple references to nonexistent cases that were hallucinated by the text generator.
When a 2024 study on the rate of hallucinations by ChatGPT asked the system to retrieve a randomized list of health-related studies, the results showed notable improvement from GPT-3.5 to GPT-4, but the hallucination rate still exceeded one in four articles, at 28.6%.
In some ways, the creation of text that is fact adjacent is actually encouraged by the inner workings of the system, as ChatGPT and other LLM text generators are not supposed to exactly replicate any of their training data. Afterall, to do so would likely be copyright infringement, as was discussed in part one of this blog series. Unfortunately, as AI formulates alternative phrasing to answer your prompts, preservation of the facts contained in the data it was trained upon is not a factor in the algorithm.
2. Fluctuations
Another pitfall of ChatGPT and other such AI text generators on public release is the fact that maintenance and quality control are largely out of the users’ hands and can fluctuate over time, without any clear reason as to how these shifts in accuracy, tone, or other quality aspects came about.
When talking about fluctuations in accuracy, many online articles reference a 2023 study by researchers at Stanford and UC Berkeley in which GPT-4’s ability to identify prime numbers dropped from 84% to 51% over a period of just three months. On the whole, accuracy does appear to be trending upwards with each new version that is released, but the ways that these models are adjusted and the cascading effects that this can have on the results and their accuracy is not public knowledge, if such changes can be comprehensibly mapped at all.
Furthermore, even within a single session, challenging the results that are provided or requesting clarification on a topic can cause the system to change its stance and provide conflicting results. This indicates a lack of precision on the part of the system, aka the ability to produce the same results more than once. This is especially prevalent in instances where the system is already hallucinating its response. Without both accuracy and precision, users must be cautious when utilizing results generated automatically by an LLM.
3. Training Restrictions
Another point worth making about the accuracy of the responses that are output from ChatGPT and other LLMs is that they are limited by the scope of the training data that was input into them. One of the biggest limiters is the “knowledge cutoff date,” which marks the latest date on which the information used to train the model was gathered. This varies by LLM but effectively marks the date after which no new data is added to the training set. The result is that LLMs contain no information or context for questions regarding the most recent world developments, technological advancements, social trends, news, and so on. Many systems, including recent iterations of ChatGPT, have found ways to mitigate this shortcoming by integrating internet search capabilities into their systems, but the risk of receiving outdated information or hallucinations is nevertheless increased beyond the cutoff date.
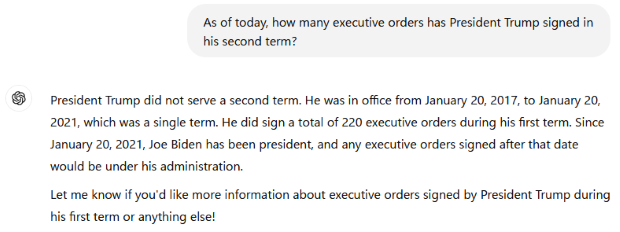
(This prompt was given to ChatGPT 4o mini on January 27, 2025, approximately one week after Trump was inaugurated for his second term.)
Another restriction is simply the volume of content on which to train. This may seem odd, considering GPT-3.5 was trained on 570GB of text data amounting to approximately 300 billion words, but the fact remains that some topics receive much more publicity than others, and looking to ChatGPT for detailed answers regarding niche topics is less likely to yield satisfactory results. As we’ve mentioned above, LLMs like ChatGPT work by creating sentences based on common word associations. This means that popular topics and generalized descriptions, which are more likely to appear in the training data, are also more heavily associated by the systems and can be generated with fewer issues, though even popular topics cannot be explored in much depth and lack intuition or insight. Similarly, if a topic has little documentation or is particularly complex and open-ended, AI systems have a much harder time creating reliable output for it at all.
And finally, the language of prompts and responses can be a limiting factor in generating good and accurate text by an LLM. Models like ChatGPT, Copilot, and Meta AI are all operated by American companies and were trained on a larger volume of English text than any other language. As a result, English is the most accurate language when it comes to understanding prompts and generating answers. As of January 2025, ChatGPT has shown some level of proficiency in nearly 100 spoken languages, not to mention the handful of coding languages on which it has been trained. However, the fluency and the scope of knowledge can vary greatly, depending on the language.
4. Solutions to Improve Accuracy
Ask straightforward questions.Do not rely on an LLM for opinions, insights, or advice on complex topics, moral quandaries, or open-ended questions.
Write precise prompts.Thoroughly explain the parameters in your prompt, including the format of the desired output. Provide examples and include other reference materials when relevant.
Avoid niche topics.Note that topics requiring expert analysis and/or extensive research are not suitable for LLM generation and are likely to result in hallucinations.
Use English. Even when writing a prompt for analyzation or output in another language, prompts written in English are generally processed more quickly and have a higher accuracy rate.
Of course, regardless of the measures taken, accuracy from an LLM cannot currently be guaranteed, so the greatest solution is human oversight. Try to find uses for AI that will streamline tedious or time-consuming tasks, especially those that would benefit from simple language analysis or the generation of a framework to be further developed by human experts.
There’s still a long way to go before AI programs can truly think for themselves, so if accuracy and relevance are important to your business, perhaps reconsider your reliance on an LLM as the last-step solution to your work tasks.
Sources:
Adjust Your AI Startup Dreams About Using ChatGPT Because OpenAI Says There Are These Starkly Prohibited Uses, Per AI Ethics And AI Law
New York lawyers sanctioned for using fake ChatGPT cases in legal brief
Hallucination Rates and Reference Accuracy of ChatGPT and Bard for Systematic Reviews: Comparative Analysis
How Is ChatGPT’s Behavior Changing over Time?
Knowledge Cutoff Dates for ChatGPT, Meta Ai, Copilot, Gemini, Claude
ChatGPT: Everything you need to know about OpenAI’s GPT-4 tool
ChatGPT Languages: How Many Does It Speak? (Full List)