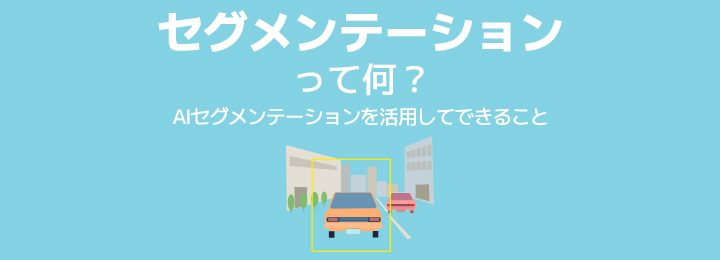
The use of AI products and services utilizing image recognition technology has been rapidly increasing in recent years. There are various types of AI image recognition technology, including image classification and object detection, as well as region extraction. In previous blog posts, we have explained object detection, but this time, we would like to explain region extraction.
- Table of Contents
-
- 1. What is Segmentation?
~Semantic, Instance, and Panoptic Segmentation~- 1-1. Semantic Segmentation
- 1-2. Instance Segmentation
- 1-3. Panoptic Segmentation
- 2. Segmentation Case Studies
- 1. Case 1: Inspection of Infrastructure Facilities (Detection of Rust and Other Areas)
- 2. Case 2: Medical Diagnosis (Detection of Lesion Area)
- 3. Case 3: Autonomous Driving (Detection of Surrounding Objects and Drivable Area)
- 3. Summary (HS Case Studies Using Segmentation)
- 4. For inquiries about utilizing AI, please contact Human Science Co., Ltd.
- 4-1. Utilize the latest data annotation tools
- 4-2. 48 million records of teacher data creation
- 4-3. Resource Management without Using Crowdsourcing
- 4-4. Equipped with a security room within the company
- 1. What is Segmentation?
1. What is segmentation?
Segmentation refers to the process of identifying the subject captured in an image and determining which class it belongs to at the pixel level, with the meaning of "division/division".
As shown in the figure above, semantic segmentation allows for a more visual and detailed understanding of the shape of the object compared to image classification and object detection. Additionally, since labeling is done at the pixel level, it is more suitable for AI models that require higher density and more detailed predictions than image classification and object detection.
However, unlike the rectangles (bounding boxes) used for object detection training data, annotation work for segmentation is generally more difficult and time-consuming.
There are three types of segmentation methods: semantic segmentation, instance segmentation, and panoptic segmentation.
1-1. Semantic Segmentation
Semantic segmentation classifies objects in an image at the pixel level. For example, it defines classes such as "road", "car", "person", "sky", and "building", and classifies them at the pixel level. Even if multiple "cars" are captured, they are all classified as "car" and not differentiated as "car1", "car2", etc. Semantic segmentation is suitable for recognizing spatial elements such as "sky" and "floor" as well, as it classifies all pixels in an image.
*A class is the name given when classifying each target that you want to detect with AI.
1-2. Instance Segmentation
Instance segmentation is a method that classifies subjects of the same class in an image, in addition to the method of semantic segmentation. In annotation, if there are multiple objects of the same class in an image, they are classified separately for each object. For example, if there are multiple cars in an image, each car is given an ID and identified by AI as "car 1" or "car 2". Usually, instance segmentation only classifies specific objects that need to be identified. Unnecessary areas such as "sky" or "floor" in the background are not classified.
1-3. Panoptic Segmentation
Panoptic segmentation is a method that combines semantic segmentation and instance segmentation. It classifies all pixels in an image by class (semantic) and also classifies all classes individually (instance). In other words, with panoptic segmentation, it is possible to classify objects of the same class individually and classify the entire image, including the background, at the pixel level. The figure below compares the differences between these three types of segmentation.
(a) Original image photo
(b) Image with semantic segmentation applied. The entire image is classified into "vehicle group", "road surface", "sidewalk", "building", and "background".
(c) Image with instance segmentation applied. Vehicles are classified individually.
(d) Panoptic segmentation. All subjects are classified, including individual entities.
2. Segmentation Case Studies
As mentioned in the previous section, segmentation performs pixel-level labeling of objects within an image. Therefore, it is commonly used for AI models that require more accurate and detailed detection compared to image classification or object detection. In other words, while object detection can detect the target object, it cannot accurately capture its shape or contour. This is why segmentation is used for AI products and services that require contour and shape recognition.
Here, we would like to introduce a case study of utilizing AI models using deep learning for segmentation services.
● Case 1: Infrastructure Inspection
- This is an example of how the electric infrastructure and telecommunications industry can be utilized. From images taken by drones of facilities such as transmission towers, AI can extract areas where paint has peeled off and rust, and even identify the progress of rust. This allows for safer implementation of tasks that previously required humans to physically climb the towers and visually inspect them, reducing labor and enabling efficient maintenance inspections.
>> Drone and AI Inspection of Transmission Towers ● Case 2: Medical Support (Lesion Detection)
- AI is being used to streamline diagnostic operations. Using images such as X-rays, ultrasounds, CT scans, and MRIs, AI is able to detect areas that may indicate lesions or tumors. This technology now supports diagnostic processes that previously relied solely on a physician's experience, reducing the burden on doctors, as well as the time and potential errors involved in diagnosis. It is currently being applied to detect various human organs and lesions, enabling highly accurate real-time diagnosis.
>> Artificial intelligence (AI) supporting image diagnosis to alleviate physician workload ● Case 3: Autonomous Driving
- In autonomous driving, it is necessary for AI to accurately identify elements related to driving, such as traffic signals, oncoming vehicles, pedestrians, and lanes, from the surrounding objects. Segmentation allows for the division of surrounding images into pixels, enabling precise processing. For this purpose, annotation of objects and road signs around the car must be carried out according to appropriate rules. As a result, the recognition accuracy of AI will improve, allowing for safe driving.
>>Unmanned autonomous driving "Level 4" runs through the city
3. Summary
This time, we have discussed segmentation, its types, and use cases. These segmentations are currently being applied to a wide range of AI fields, and in the future, the fields in which they are applied will continue to expand and become even more ingrained in human life. As if to prove this, the development of AI for companies that request our annotation services is truly diverse.
This is just one example, but in addition to the previous use case, we would like to introduce our AI image recognition technology that we provide through our segmentation annotation service.
https://www.science.co.jp/annotation/experience/index.html
<Industry Examples>
● Medical Industry: Surgical Support, Diagnostic Support (Object Detection)
https://www.science.co.jp/annotation/industry/medical.html
● Automotive Industry: Autonomous Driving Project 2D/3D (Object Detection)
https://www.science.co.jp/annotation/industry/automobile.html
● IT Industry: Automatic Recognition of Invoices (Character Recognition)
https://www.science.co.jp/annotation/industry/it.html
As mentioned earlier, while various segmentation methods are ideal for AI models that require high accuracy and detailed predictions, the difficulty of annotation work is also high and it generally takes a considerable amount of time, resulting in high costs. If you are unable to provide personnel internally or want to reduce costs, considering outsourcing or delegating the annotation work to those familiar with segmentation annotation can be an effective solution. We offer a wide range of services, from consultation on annotation to support for creating annotation specifications, developing annotation tools, so please feel free to contact us.
For inquiries about utilizing AI, please contact Human Science Co., Ltd.
4-1. Utilize the latest data annotation tools
One of the annotation tools introduced by Human Science, Annofab, allows customers to check progress and provide feedback on the cloud even during project execution. By not allowing work data to be saved on local machines, we also consider security.
4-2. 48 million records of teacher data creation
"I want to introduce AI, but I don't know where to start."
"I don't know what to ask for when outsourcing."
Please consult Human Science Co., Ltd. in such cases.
At Human Science, we are involved in AI development projects in various industries such as natural language processing, medical support, automotive, IT, manufacturing, and construction. Through direct transactions with many companies including GAFAM, we have provided over 48 million high-quality training data. We can handle various annotation projects regardless of industry, from small-scale projects to large-scale projects with 150 annotators.
>>Human Science's Annotation Services
4-3. Resource Management without Using Crowdsourcing
At Human Science, we do not use crowdsourcing and instead directly contract with workers to progress projects. We carefully assess each member's practical experience and evaluations from previous projects to form a team that can perform to the best of their abilities.
4-4. Equipped with a security room within the company
At Human Science, we have a security room that meets the ISMS standards in our Shinjuku office. This allows us to provide on-site support for highly confidential projects and ensure security. We consider confidentiality to be extremely important for all projects at our company. We continuously provide security education to our staff and pay close attention to the handling of information and data, even for remote projects.