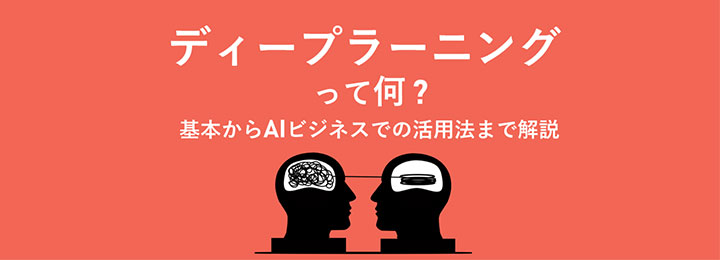
One of the terms that almost always comes up when discussing AI is deep learning. Here, for those who are wondering, "What exactly is deep learning?", we will discuss its basic mechanisms, the differences from traditional machine learning, and key points for implementing deep learning in business.
- Table of Contents
-
- 1. The Mechanism of AI Development and Deep Learning
- 1-1. What is Deep Learning
- 1-2. What is Machine Learning
- 1-3. Types of Machine Learning
- 1-4. Neurons and Neural Networks
- 1-5. What is being done in deep learning
- 1-6. Key Points for Implementing Deep Learning
- 1-7. Background of Deep Learning Development
- 1-8. Differences Between Deep Learning and Machine Learning
- 1-9. Distinguishing Between Deep Learning and Machine Learning
- 2. Case Studies Utilizing Deep Learning
- 2-1. Medical Support
- 2-2. Development of Autonomous Driving Technology
- 2-3. Improving the Accuracy of Natural Language Processing
- 2-4. Ensuring Safety at Production and Construction Sites
- 3. Recommended Books for Learning About Deep Learning
- 4. Human Science Annotation Agency Services
- 4-1. Extensive track record of creating 48 million teacher data entries
- 4-2. Resource Management Without Using Crowdsourcing
- 4-3. Utilizing the latest data annotation tools
- 4-4. Fully equipped security room within the company
1. The Mechanism of AI Development and Deep Learning
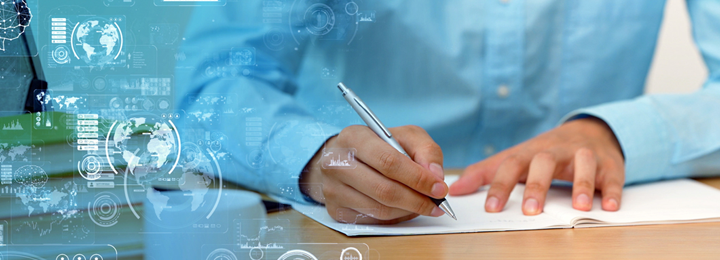
1-1. What is Deep Learning
Deep learning is one of the methods of machine learning, which is a process of AI development. Although its adoption has been expanding in recent years, its fundamental mechanisms have existed for some time. To understand the differences from traditional machine learning, I will explain from the perspective of AI development.
The positioning of machine learning in AI development is as follows.
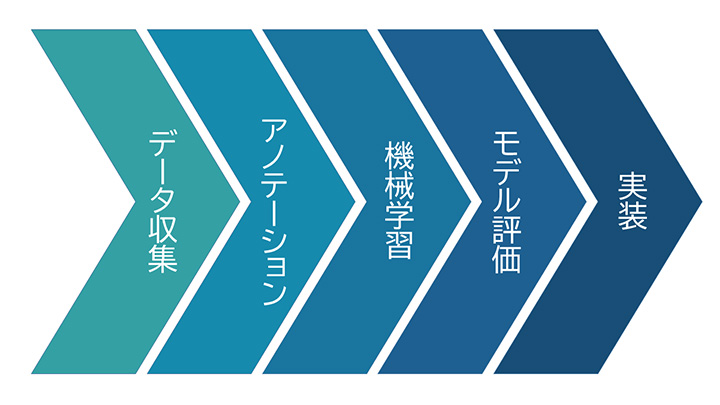
For the meaning of annotation, click here
>>What is annotation? An explanation from its meaning to its relationship with AI and machine learning.
Click here for articles about training data used in machine learning
>> What is training data? An explanation from its relationship with AI, machine learning, and annotation to how to create it.
1-2. What is Machine Learning
Machine learning is a field of AI. It is a technology that allows machines to learn by providing them with data and patterns in advance, enabling them to classify and identify new data. It is utilized in various fields such as data analysis, image analysis, and natural language processing. Before the advent of machine learning, it was necessary to manually design rules and algorithms. These methods are referred to as rule-based systems. In rule-based systems, predictions and analyses can be performed with high accuracy for data that fits specific rules, but they cannot flexibly respond to data that falls outside those rules.
1-3. Types of Machine Learning
There are three main types of machine learning.
1. Supervised Learning Uses labeled training data with correct answers to teach the machine. Classification and regression algorithms are utilized. Linear regression and random forests are representative algorithms. 2. Unsupervised Learning Does not prepare training data with clear answers or correct responses; instead, the machine automatically discovers patterns such as regularities and similarities in the data. Algorithms such as clustering and association analysis are used. 3. Reinforcement Learning Is characterized by not preparing data in advance. The machine learns through trial and error. Humans specify scores (rewards/goals), and the machine autonomously makes judgments and iterates through trial and error to maximize these scores. Algorithms that search for the actions that yield the highest scores are used, such as Q-learning and SARSA.
These learning methods are types of machine learning, but with the emergence of a new technique called neural networks, machine learning has evolved significantly.
1-4. Neurons and Neural Networks
The human brain is composed of nerve cells called neurons. It is believed that complex connections between many neurons enable information processing within the brain. The idea behind AI development started from the notion that if we mimic the structure of the human brain, we might be able to replicate human intelligence. This model, which simulates the workings of neurons, is called a neural network.
1-5. What is being done in deep learning
When input is provided to the AI, the neural network performs calculations where each unit interacts with one another to adjust their respective biases. In machine learning through deep learning, by structuring the intermediate layers in a multi-layered format, calculations and determinations are made with an enormous number of neurons compared to traditional simple-layer neural networks.
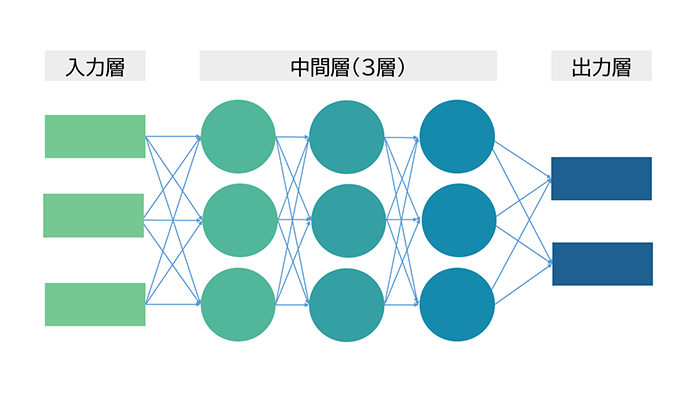
This is an illustrative diagram of a deep learning neural network. The circles represent each unit corresponding to neurons, and the arrows connecting them indicate the decision pathways of AI. It can be seen that the more layers are added to the intermediate layer, the greater the variety of pathways from the input layer to the output layer. The increase in pathways contributes to the ability to handle complex computations. In this diagram, there are three layers in the intermediate layer, but in actual deep learning, more layers may be used.
1-6. Key Points for Implementing Deep Learning
To effectively realize AI learning with deep learning, a larger amount of data is required compared to traditional methods. High-performance hardware capable of processing vast amounts of data is also necessary. In cases with limited data, other methods may yield better results. It is important not to assume that deep learning is suitable for all AI development projects, but rather to comprehensively assess whether to adopt deep learning by considering the amount of data available, annotation tools, and cost performance.
1-7. Background of Deep Learning Development
The theory of deep learning first emerged in the 1980s. The recent advancements are attributed to improvements in computer performance and the availability of data. Deep learning requires a vast amount of labeled data. However, preparing the materials before labeling has not been easy due to the difficulty in obtaining large quantities of data. With the recent enhancements in computer processing power and the trend of digitizing all types of data, especially images and videos, it can be said that theories that have existed for a long time have become practical.
1-8. Differences Between Deep Learning and Machine Learning
Deep learning is positioned as one of the various techniques in machine learning. It can be considered an advanced form of traditional machine learning. The most significant feature of deep learning, which is not present in conventional machine learning, is that machines can automatically learn without human intervention. Human intervention refers to the act of providing "features" to the machine. In machine learning, humans provide the "features" for the machine to derive the correct answers, whereas in deep learning, the machine itself discovers the "features" and progresses in its learning.
*Features are elements that influence predictions, or in other words, they refer to the variables used to derive predictions. For example, in sales forecasting, elements such as 'price', 'color', 'brand', 'shelf position', and 'weather' fall into this category. By appropriately selecting these elements, high-accuracy predictions can be achieved. When human intervention is involved, it is necessary to find the appropriate features, and if this is done incorrectly, high-accuracy predictions cannot be obtained. In deep learning, machines can reach optimal solutions by discovering features on their own without human intervention.
1-9. Distinguishing Between Deep Learning and Machine Learning
The distinction between deep learning and machine learning varies depending on the type and amount of data, as well as the challenges and objectives to be addressed.
First, regarding the types and amounts of data, in deep learning, as mentioned above, machines generally require a vast amount of data to discover features on their own, while machine learning can achieve high accuracy even with relatively small amounts of data by using appropriate algorithms.
Next, the usage varies depending on the issues or objectives you want to address. Deep learning excels at complex pattern recognition and prediction problems. Examples include image recognition, speech recognition, and natural language processing. On the other hand, machine learning is suited for relatively simple problems such as regression analysis, clustering, and classification, and can generally achieve high accuracy even with less data.
Furthermore, deep learning generally requires a lot of computational resources, necessitating fast computing devices such as GPUs. On the other hand, machine learning can be executed with relatively lightweight computations, making it feasible on standard CPUs.
As mentioned above, the distinction between deep learning and machine learning varies depending on the type and amount of data, as well as the challenges and objectives to be addressed. By selecting the appropriate method, we can provide efficient and accurate solutions.
2. Case Studies Utilizing Deep Learning
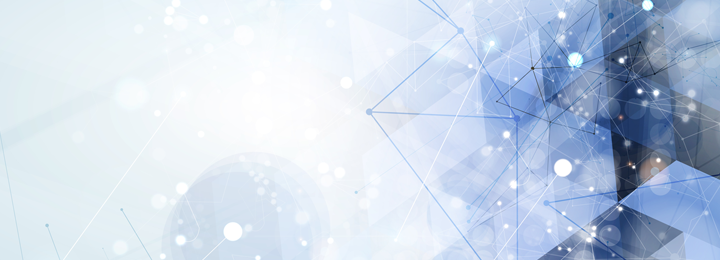
Most of the current AI is developed using deep learning. By introducing deep learning techniques in these fields where AI has traditionally been implemented, the potential and accuracy that AI aims for have dramatically improved.
2-1. Medical Support
Using endoscopic images and X-ray images as materials, we identify features and potential areas of concern with AI. This helps prevent oversight of tumors and lesions and improves diagnostic accuracy.
2-2. Development of Autonomous Driving Technology
Utilizing images and video materials from dashcams capturing accidents and near misses, we enable AI to accurately recognize all elements such as traffic signs, pedestrians, and oncoming vehicles.
2-3. Improving the Accuracy of Natural Language Processing
It helps improve the accuracy of voice recognition and intent extraction represented by "Hey Siri" and "OK, Google" used in smartphones and AI speakers.
2-4. Ensuring Safety at Production and Construction Sites
Captures the movements of workers engaged around manufacturing equipment and heavy machinery at production and construction sites. By automatically detecting when workers enter hazardous areas, AI contributes to ensuring their safety.
3. Recommended Books for Learning About Deep Learning
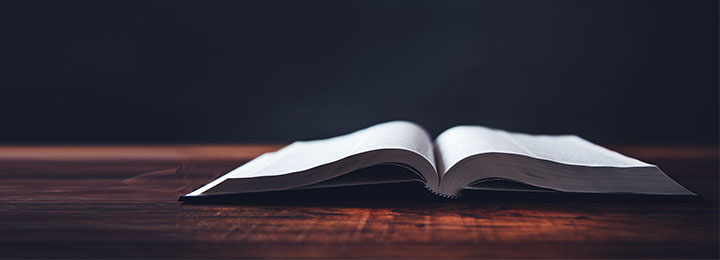
So far, we have discussed an overview of deep learning. To gain a deeper understanding of its mechanisms, deepening your knowledge through books is the shortcut. Here, we will introduce selected books on AI and machine learning, including deep learning.
This explains business growth know-how using machine learning in a way that is understandable even without knowledge of IT or mathematics. You can learn what managers should know, from the mechanisms of AI and deep learning to project management.
What AI Can and Cannot Do - Four Skills for Thriving in the Business World
This is a book written with the concept of understanding what AI can and cannot do in order to leverage AI effectively! It is very well organized, covering the reality of AI, essential knowledge about deep learning, and introducing actual case studies in a very readable manner.
How to Implement AI in Business
This book focuses on the know-how for companies launching projects that actually implement AI. It introduces real examples of companies that have adopted AI, showcasing their before & after across a wide range of industries. You can strategically learn how to leverage AI and deep learning for business.
Deep Learning Textbook Deep Learning G Certification (Generalist) Official Text
This is the official textbook for the G Test (Generalist Test), which is a certification exam for foundational knowledge of deep learning and business application skills. It consolidates everything from the basics of deep learning to the latest developments and practice problems in one book. Even if you do not plan to take the certification exam, it is ideal for systematically learning the knowledge.
4. Human Science Annotation Agency Services
4-1. Extensive track record of creating 48 million teacher data entries
At Human Science, we participate in AI development projects across various industries, including natural language processing, medical support, automotive, IT, manufacturing, and construction. To date, we have provided over 48 million high-quality training data through direct transactions with many companies, including GAFAM. We handle a wide range of annotation projects, from small-scale projects to long-term large-scale projects with 150 annotators, regardless of the industry. If your company wants to implement AI but doesn't know where to start, please feel free to consult with us.
4-2. Resource Management Without Using Crowdsourcing
At Human Science, we do not use crowdsourcing. Instead, projects are handled by personnel who are contracted with us directly. Based on a solid understanding of each member's practical experience and their evaluations from previous projects, we form teams that can deliver maximum performance.
4-3. Utilizing the latest data annotation tools
One of the annotation tools introduced by Human Science, AnnoFab, allows customers to receive progress checks and feedback from the cloud even during the project. By ensuring that work data cannot be saved on local machines, we also take security into consideration.
4-4. Fully equipped security room within the company
Human Science has a security room that meets ISMS standards within our Shinjuku office. We can handle even highly confidential projects on-site. We consider the assurance of confidentiality to be extremely important for any project. Our staff undergoes continuous security training, and we exercise the utmost care in handling information and data, even for remote projects.