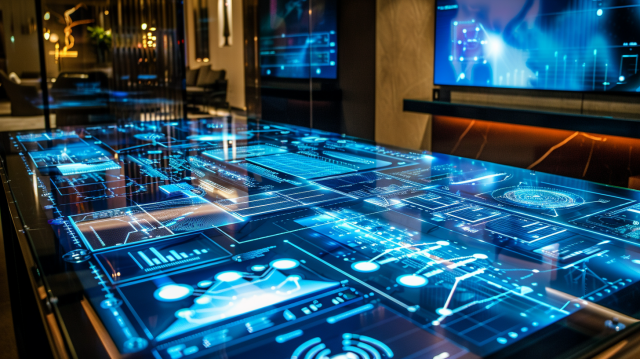
Spinoff Blog Project
――Annotations Supporting AI in the DX Era: The Reality of the Analog Field
What is the Unexpected Difficulty of Annotations?
~Tips for Selecting Annotation Outsourcing Service Companies Based on Difficulty~
Until now, our company has been publishing various blogs related to annotation and AI. In those, we have focused on conveying general knowledge and know-how. While the task of annotation may seem simple when expressed in words, it is a task that inevitably involves a lot of human interaction due to its inherent "ambiguity" and the fact that it is a "task that cannot be avoided by humans." Therefore, it often becomes quite messy and cannot be resolved with the neat theories that are commonly found. In order to ensure quality and productivity, a variety of experiences and know-how are actually required.
Therefore, understanding the specific problems that occur in the actual annotation field and how to address them can serve as helpful hints for leading to successful annotation.
What actually happens in our field and what specific responses and measures we take. Unlike a regular blog, we would like to share the realities of our field, including our unique features and commitments, under the spin-off blog project titled: "Annotations Supporting AI in the DX Era. The Reality of the Analog Field."
>>Past Published Blogs (Partial)
How to Outsource Annotation Work? 7 Tips
Dealing with Edge Cases Not Covered in Specifications
- Table of Contents
1. What is the unexpected difficulty of annotations?
This time, I would like to discuss the unavoidable difficulty of annotation that must be considered when outsourcing annotation or selecting a service provider.
Those of you reading this blog can easily imagine "high-difficulty annotation" when domain expertise or domain knowledge is required for annotation and labeling in specific fields. For example, special appearance defects in fields such as healthcare or manufacturing often cannot be judged or labeled without familiarity with that field, and customers considering outsourcing or delegation of annotation may often have concerns in this area.
However, there are surprisingly high-difficulty tasks, and when outsourcing or delegating annotation, it is important to be cautious as there may be a decline in quality or costs may be higher than expected. Therefore, this time, excluding those that require expertise or specific domain knowledge, I would like to discuss what high-difficulty annotations are from the perspective unique to the field of annotation.
There are many types of labels and classes
Before starting the annotation work, the worker should familiarize themselves with the types of labels and specifications to some extent. It is somewhat intuitive, but a human can typically remember about 10 types of labels while working. This does not apply if the labeling involves objects that one frequently encounters in daily life. However, when the number of label types exceeds 15 to 20, the worker will need to refer to the specifications and work procedures each time, which can lead to decreased productivity and work efficiency, resulting in increased costs and extended work periods. Additionally, as the number of labels increases, there will naturally be more similar labels, leading not only to confusion in judgment but also a higher likelihood of labeling errors. While this issue tends to resolve as one becomes more proficient, in cases of low-volume, short-term annotation, the work may end just as one is becoming accustomed to the task.
Many exceptions and edge cases
It is particularly common in language-related text annotations, but when there are many exceptions and edge cases not specified in the specifications, work often comes to a halt. In such cases, one must check the Q&A sheet where methods for handling specifications and edge cases are accumulated, and if it is still unclear, the judgment will be sought from a PM or reviewer/QA personnel who is familiar with the specifications. However, there are often cases where even the PM cannot make a judgment, so in such cases, the PM will ask the customer questions and discuss to determine the policy.
To ensure quality, the PM needs to compile these Q&A examples and share them with all workers, creating an environment where they can easily view and confirm the information. In language-related annotations, it is somewhat inevitable that such exceptions and edge cases will increase. However, as the number of exceptions and edge cases grows, there is a risk that information may not reach the workers, or that workers may focus solely on their tasks and often overlook details. This can lead to an increased probability of errors. Therefore, the PM must determine whether the updates to the Q&A sheet affect the entire workforce or if it is sufficient to communicate the response methods to the specific workers involved. Additionally, since workers cannot retain too much detailed information, it is important to abstract and clearly communicate policies and directions, hold meetings with workers to provide clear verbal explanations, and implement various management strategies to ensure quality.
High ambiguity - there is no absolute correct answer
This is also often seen in text and dialogue annotations, for example, labeling that classifies types of human emotions in dialogue text. Annotations in areas where judgments can vary greatly among individuals tend to be more difficult overall. For expressions of emotion, if the worker feels a certain way, in a sense, there is only one correct answer, and they can only assign the label that reflects how they felt.
To ensure the quality of annotations that are influenced by human perception, it is essential to carefully select and assign workers, along with managing the suitability of the personnel involved. Additionally, during the work process, at the beginning, workers tend to proceed cautiously by checking specifications and procedures, which aligns the labeling tendencies with the annotation specifications. However, as the work continues, the workers' sensitivity may dull, leading to a gradual misalignment of direction and the boundaries of different labels, resulting in annotation outcomes that unintentionally deviate from the specifications.
In a sense, there is no absolute correct answer for annotation, which is often done through a process called "consensus check," where multiple people annotate the same material and derive correct examples through majority vote or agreement rate. In such annotations, it is common not to conduct third-party rechecks or reviews, and even when third-party checks are performed, they often have little effect. Therefore, it is important for the PM to regularly check the labeling tendencies of the workers to ensure quality and provide instructions to correct the direction as needed.
2. Tips for Outsourcing Annotations Based on Difficulty
Do not increase the number of labels or classes unnecessarily
There are purposes and goals for AI development, so I think there are some things that are unavoidable to a certain extent. However, if we think, for example, 'just in case, let's label this too,' I believe the number of labels and classes will continue to increase. This relates to the balance and relationship with the development purposes and goals, but it is important to clearly set the goals of AI development and the annotation specifications, and to avoid unnecessarily increasing the number of labels and classes.
Choose an annotation vendor skilled in handling exceptions, edge cases, and information management
Exceptions and edge cases are inherent to annotation, and a significant amount of time spent on managing annotation work is dedicated to addressing these exceptions and edge cases. From the perspective of those on the ground in annotation, it is not an exaggeration to say that management in annotation work "begins and ends with edge cases," in addition to the setup and preparation of annotation projects.
If information management and communication regarding edge cases are not thoroughly conducted, it can lead not only to operational mistakes and errors but also to a situation where reviewers and checkers responsible for QA do not understand how to handle edge cases and exceptions, making their checks meaningless. Therefore, it is essential for the PM to have the know-how to manage information appropriately and ensure that everyone involved in the annotation work is well-informed. Particularly, such information is often difficult for workers to grasp through text alone, and sometimes it is necessary to hold meetings to convey nuances verbally and to ascertain whether the other party understands. In this sense, rather than relying on vendors that focus on repeatedly checking and correcting errors through sheer effort, it is more beneficial to engage vendors that prioritize preventing errors from occurring in the first place, which ultimately helps to keep costs low and ensure stable quality.
Choose a vendor with extensive experience in high-ambiguity annotation work
As mentioned earlier, there are specific know-how and management methods for consensus checks and annotations decided by majority vote. Even if there are no checks on annotations determined by the majority of workers, simply leaving the work unfinished will not ensure the expected quality. In particular, text annotations such as emotion labeling often rely heavily on human sensibility and perception, making it essential to understand the characteristics of personnel in advance to assign the right individuals. In this sense, effective personnel suitability management, meticulous management, and engaging vendors with experience in such annotations and checking methods will lead to better results.
3. Summary
What has been stated so far may not strictly mean that "the difficulty of annotation is high" in a pure sense. However, if these factors are underestimated, whether performing annotation in-house or outsourcing to an annotation service, it can significantly impact the desired quality, cost, and delivery time, leading to results that do not meet expectations. In that sense, it can be said that the difficulty is high. Ultimately, highly specialized annotation is difficult because it cannot yield correct answers, which means it is challenging to ensure the expected quality. In this regard, the same factors discussed so far apply to highly specialized annotation as well.
For those designing annotation specifications, who are deeply engaged in the specifications and have conducted extensive discussions, the factors that increase the difficulty of annotations, as mentioned so far, may not seem particularly challenging. However, for annotation workers who only have general knowledge or are seeing a specification document for the first time, it is quite natural that the barriers to work can be high. It would be unfortunate if you found that the estimate from an annotation outsourcing company was unexpectedly high, or if you discovered that the delivered data contained many errors. I hope this serves as a reference to avoid situations where you are asked to review costs after the work is done.
Author:
Kazuhiro Sugimoto
Annotation Department Group Manager
・At my previous job at a Tier 1 automotive parts manufacturer, I focused on quality design and quality improvement guidance for the manufacturing line, and I have experience as a project manager for model line construction and in cross-departmental projects such as business efficiency improvement (lean improvement) consulting.
・In my current position, I have been involved in the establishment and expansion of the annotation business, the construction and improvement of the management system for annotation projects, following my work on management systems such as ISO and promoting knowledge management. QC Level 1, Member of the Japan Society for Quality Control