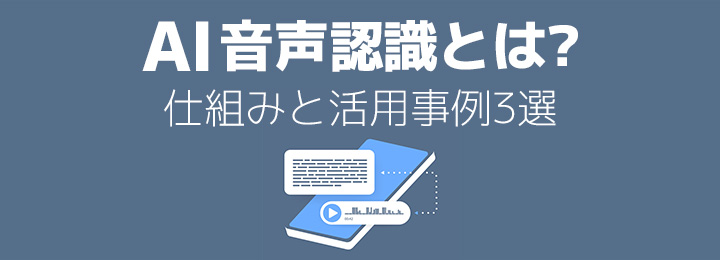
With voice assistants such as Siri and Alexa installed on smartphones, voice input to machines has become more familiar. The advantage of voice input is that it allows for more intuitive input without the need for input interfaces such as keyboards. However, since voice is data that machines cannot understand as is, "speech recognition" technology is used to convert it into text so that machines can understand it. In recent years, the accuracy of this speech recognition has rapidly improved, supported by technological innovations by AI.
Technology for Converting Voice to Text
When AI uses voice recognition technology to convert speech to text, it uses a technology called pattern recognition, which can identify patterns based on the characteristics of the subject.
By teaching AI about the genre or topic it wants to investigate, it becomes possible for AI to convert voice data into text.
Not only for voice, but also for faces and buildings, which are difficult to process logically, using pattern recognition can greatly expand the range of information that AI can process.
The Importance of Algorithms
By the way, in order to operate a computer, you need an "algorithm". All computers and websites operate according to a certain algorithm.
An algorithm is like a process or calculation method that leads to the correct answer for a given problem or task. By following the provided questions or patterns, the computer can present the correct information that the user is looking for.
Of course, even AI pattern recognition requires algorithms to learn information such as sound and images in order to function correctly.
In this article, we will introduce the basic mechanism of voice recognition using AI, and also explain actual use cases.
- Table of Contents
-
- 1. Voice Recognition Mechanism
- 1-1. Benefits of Utilizing Voice Recognition
- 1-2. Acoustic Analysis
- 1-3. Acoustic Model
- 1-4. Pronunciation Dictionary
- 1-5. Language Model
- 2. Examples of Utilizing AI Voice Recognition
- 2-1. Example of Use 1: Introduction of Multilingual AI Robot at Station Information Center
- 2-2. Case Study 2: Business Improvement and Efficiency in Call Centers
- 2-3. Example of Use 3: Automation of Meeting Minutes
- 3. Summary
- 4. Consult with Human Science for Voice Data Annotation
- 4-1. Number of teacher data created: 48 million records
- 4-2.Resource Management without Using Crowdsourcing
- 4-3. Utilizing the Latest Data Annotation Tools
- 4-4. Equipped with a security room within the company
1. Mechanism of Speech Recognition (Four Steps from Voice to Text)
Speech data input by recording equipment such as microphones is in a state where various waveforms are mixed. It is easy for our ears to identify human voices from this, but it is not an easy task for machines. Even if the waveform corresponding to the words can be identified, the machine cannot recognize it correctly and give the correct instructions without converting it into text data. In addition, it is necessary to correctly select homophones and other words during text conversion. To solve these problems, we will go through four main steps to convert speech into text. The technologies used in each step are acoustic analysis, acoustic model, language model, and pronunciation dictionary, which will be introduced next.
1-1. Benefits of Utilizing Voice Recognition
Here are three benefits of introducing voice recognition.
①Efficiency in business
By eliminating the need for transcription work in meetings and negotiations, efficiency in business can be achieved. For example, in the case of creating minutes, it used to be necessary to listen to recorded audio data and type it out. However, with voice recognition technology, it is possible to create them semi-automatically, leading to a reduction in work time.
②Reduction of input errors
Human typing errors cannot be completely eliminated as long as humans are performing the work. On the other hand, voice recognition has the advantage of being able to prevent mishearing and input errors. Of course, there are some points to note regarding noise and conversation volume, but by making corrections on the human side, it is possible to achieve a higher level of transcription.
③Hands-free input is possible
With voice recognition, transcription can be done hands-free. This allows for the possibility of text conversion while performing other tasks. It can also lead to increased efficiency for those who are not good at typing or spend a lot of time on transcription.
1-2. Acoustic Analysis
Audio analysis is the process of analyzing the characteristics of input audio (such as frequency and volume) and extracting and converting it into data that can be easily handled by AI. AI cannot recognize speech from raw audio data like humans can. For example, in a noisy city, humans can easily recognize a specific person's voice. However, before audio analysis, AI recognizes various sounds as mixed together. Therefore, it is necessary to digitize and remove noise such as background noise so that AI can recognize human speech. This process is called audio analysis. Based on the extracted human speech data, AI continues to advance speech recognition.
1-3. Acoustic Model
An acoustic model is the process of extracting phonemes by comparing data extracted through acoustic analysis with data previously learned by AI. Phonemes are the smallest units of meaning when speech is divided, and in Japanese, they are considered to be vowels, consonants, and glottal stops. It can be thought of as the individual characters in "o-mo-te-na-shi". The training data for phoneme extraction consists of processing the voices of thousands of people over thousands of hours. This allows AI to extract the necessary information for converting speech to text.
1-4. Pronunciation Dictionary
Once the phoneme, which is the smallest unit of speech, is determined by the acoustic model, it is necessary to reconstruct that phoneme into the correct word. This is where a pronunciation dictionary is used. It serves as a database for combining the phonemes extracted by the acoustic model to form words. By using this pronunciation dictionary to combine phonemes, it is possible to construct a word acoustic model that corresponds to the word for the first time. In the previous acoustic model, the phonemes "o-mo-te-na-shi" were combined to create the word "omotenashi". Once words are formed, the next step is to move on to the language model.
1-5. Language Model
A language model is a model that represents the "words" that humans speak or write based on the probability of word occurrences. For example, the phrase "hospitality for customers" could also be "hospitality for clients", but it would have a lower probability. Recently, language models using neural networks have been widely used. The GPT-3, which was introduced in 2020 as a large-scale language model, uses 175 billion parameters.
With these technologies, our conversations will be recognized by AI, converted to text, and can be utilized for device operation.
2. Examples of Utilizing AI Voice Recognition
By utilizing AI voice recognition, it is possible to expand services and improve business efficiency. We will introduce three examples of how to use it.
2-1. Case Study 1: Introduction of Multilingual AI Robot at Station Information Center
By installing a conversational robot equipped with multilingual voice recognition AI at the information center of the station, it is expected to improve the efficiency of inquiries and counter services for travelers and others.
In addition, by understanding the needs of users from dialogue logs and reflecting them in services, we will be able to provide services that are more in demand by users. Analyzing dialogue logs also allows us to collect and analyze customer feedback and satisfaction, which is a strength of voice recognition AI. In addition to just serving as a voice guide, there are also benefits that can lead to improved customer satisfaction and expanded services.
>>Challenges pointed out by the Tokyo Metropolitan Bureau of Transportation's service consultant for dialogue robots installed near station ticket gates
2-2. Case Study 2: Business Improvement and Streamlining in Call Centers
The field where voice recognition technology is most commonly used is call centers. In the past, when converting call data into text in call centers, it was necessary for a person to listen to the voice data and transcribe it. With the advancement of voice recognition technology, the accuracy of transcription through AI automation has greatly improved.
In addition, call center electronic manuals can reach thousands of pages when converted to paper media, making it quite time-consuming for operators to search for FAQs. By introducing voice recognition AI to address these challenges, business improvement and improved customer satisfaction can be expected.
>>Significant efficiency improvements in call center operations with real-time voice recognition
2-3. Usage Example 3: Automation of Meeting Minutes
Until now, the minutes of meetings had to be recorded in real time by the person in charge, or transcribed while listening to recorded audio data. There is a possibility of missing or mishearing information in real time. In addition, transcription is a redundant task and can take a significant amount of time depending on the content. In the midst of a demand for faster work, transcription work can also impede other tasks and decrease the productivity of the person in charge as a time-consuming routine. By introducing speech recognition AI to address these challenges, business improvement can be expected.
>>Efficiently transcribe a wide range of materials, from meeting minutes to interviews
Combined with operational improvements, recognition accuracy has increased to over 90%
3. Summary
This time, we have introduced three examples of utilizing AI for voice recognition.
Recently, the use of AI voice recognition has been expanding. As a result, the need for creating training data for AI learning has also increased.
If you want to reduce the cost of data annotation for creating teacher data, one effective method is to consider outsourcing the annotation work. We offer a wide range of services, from consultation on annotation tools to support for creating annotation specifications, creating specifications, and outsourcing annotation work. Please feel free to contact us.
4. Consult with Human Science for Voice Data Annotation
4-1. Number of teacher data created: 48 million records
"I want to introduce AI, but I don't know where to start."
"I don't know what to ask for when outsourcing."
If you need help with that, please consult Human Science. At Human Science, we participate in AI development projects in various industries such as natural language processing, medical support, automotive, IT, manufacturing, and construction. Through direct transactions with many companies including GAFAM, we have provided over 48 million high-quality training data. We can handle various annotation projects regardless of industry, from small projects with a few people to large-scale projects with 150 annotators.
>>Human Science's Annotation Services
4-2.Resource Management without Using Crowdsourcing
At Human Science, we do not use crowdsourcing and instead directly contract with workers to manage projects. We carefully assess each member's practical experience and evaluations from previous projects to form a team that can perform to the best of their abilities.
4-3. Utilizing the Latest Data Annotation Tools
One of the annotation tools introduced by Human Science, AnnoFab, allows customers to check progress and provide feedback on the cloud even during project execution. By not allowing work data to be saved on local machines, we also consider security.
4-4. Equipped with a security room within the company
At Human Science, we have a security room that meets the ISMS standards in our Shinjuku office. We can handle highly confidential projects on-site. We consider ensuring confidentiality to be extremely important for all projects. We continuously provide security education to our staff and pay close attention to handling information and data, even for remote projects.