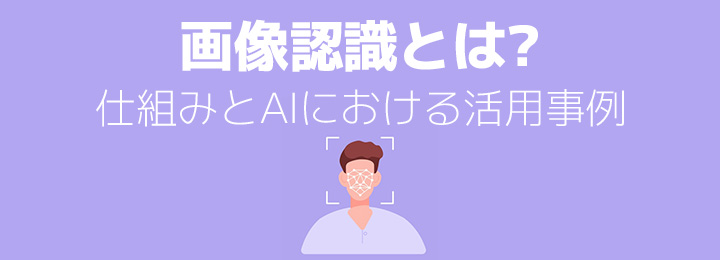
In recent years, AI image recognition technology has been active in various fields. Although image recognition technology itself has been researched and put into practical use for a long time, the development of AI technology using deep learning has been remarkable in recent years, and products and services using this technology have rapidly spread to familiar places. As the author myself, I often encounter situations where I am surprised and think, "Is this also using AI image recognition technology?" This time, I would like to explain what image recognition is, its mechanism, and how it is being utilized, using examples.
- Table of Contents
-
- 1. What is Image Recognition? Mechanism of Image Recognition
- 1-1. What is Image Recognition?
- 1-2. Mechanism of Image Recognition (Machine Learning, Introduction of Deep Learning)
- 2. Types of Image Recognition
- 2-1. Image Classification
- 2-2. Object Detection
- 2-3. Segmentation (Region Detection)
- 2-4. Character Recognition
- 3. Examples of Utilizing Image Recognition
- 4. Summary ~Image Recognition AI Developed by Our Company~
- 5. Human Science's Data Annotation and LLM RAG Data Structuring Agency Service
- 5-1. Utilizing the latest data annotation tools
- 5-2. Number of teacher data created: 48 million records
- 5-3. Resource Management without Using Crowdsourcing
- 5-4. Equipped with a security room within the company
1. What is Image Recognition? Mechanism of Image Recognition
1-1. What is Image Recognition?
Image recognition is a technology that recognizes people and objects in images, in short. Image recognition is a type of pattern recognition, and as mentioned earlier, it has been widely applied in various fields in recent years thanks to the technology of deep learning (Deep Learning).
The history of image recognition is quite old, with research having been conducted for 40-50 years. One of the earliest and most familiar forms of image recognition is barcode recognition.
In recognizing the subject in an image and determining what it is, humans use their experience (such as the difference between dogs and cats) to unconsciously identify various characteristics of the subject. However, this is not the case for computers. Computers can only recognize images as a collection of pixels or at the pixel level. As a result, various research and efforts have been made. Template matching is one example, where the image to be recognized and detected is compared to a template to extract information such as "where in the image is the object appearing" and "how many objects are appearing".
However, even with this method, it is difficult to put into practical use without carefully managing various conditions such as differences in image capture conditions and a decrease in recognition rate due to significant differences with the template image.
1-2. Mechanism of Image Recognition (Machine Learning, Introduction of Deep Learning)
Image recognition used to be a difficult technology to put into practical use, but with the emergence of machine learning and deep learning, the situation has completely changed. Machine learning has also been a long-standing technology, but with technological advancements such as improved processing speed of computers, it has become a practical and familiar technology.
(For details on the mechanism of deep learning, we will omit detailed explanations here, but please refer to the following blog on our website for more information.)
https://www.science.co.jp/annotation_blog/30343/
Deep learning is an algorithm that uses neural networks, which mimic the neural networks of human neurons, as you all know, and is now often talked about as a representative technology that supports AI. This falls under the category of pattern recognition, and by training with data labeled by humans, called training data (for example, data labeled as dog or cat for images of dogs and cats), AI can read the characteristics of dogs and cats and learn to identify images of dogs and cats.
As humans gain more experience, they become better at identifying confusing things. Similarly, the more training data there is, the more accurate AI becomes. In other words, having more data has the same effect as humans gaining more experience.
The crucial point here is preparing a large amount of training data. To prepare a large amount of training data, a large amount of labeling is necessary. (This labeling process is called data annotation.) Although automation has advanced considerably recently, the fact remains that the majority of data still requires human intervention in order to identify ambiguous elements that computers cannot recognize based on rules. Therefore, it is inevitable that a large number of people are needed and it comes with a certain cost.
As is well known, the quality of teacher data = the quality of data annotation greatly affects the identification accuracy of AI. Also, since neural networks mimic the structure of the human brain, areas where humans are prone to making mistakes will also be prone to mistakes for AI. However, when humans recognize something, their judgment can be dulled by the situation they are in, their physical condition, and their emotions, which can greatly affect the identification accuracy. This is not the case for AI. In addition, the speed of identification is incomparable to that of humans, as it can identify and process in an instant. Therefore, tasks that were previously difficult for machines to identify due to ambiguity or lack of regularity can now be automated with the introduction of AI, leading to significant effects in products and services. As a result, the application of AI has been accelerating in recent years.
2. Types of Image Recognition
So far, we have discussed image recognition and its mechanisms, but now we will introduce the representative types of image recognition: image classification, object detection, segmentation, and character recognition.
2-1. Image Classification
Image classification is a technology that classifies objects within an image. It recognizes whether or not predefined objects are present in the image. For example, image classification is the task of classifying whether the objects in the image are dogs or cats, which have been defined as objects to be recognized. Unlike object detection, which will be discussed later, image classification does not detect the position of objects.
Example 1. Scene Recognition
In scene recognition, instead of recognizing specific objects within an image, we recognize the overall characteristics of the image. If image classification is the task of recognizing whether a specific tree exists in a forest image, then scene recognition is the task of recognizing whether the image is a forest or not.
Application Example 2. Anomaly Detection
In industries such as manufacturing and construction, there is a method to detect anomalies from images as an alternative to visually detecting abnormalities in objects. Since anomalies often occur infrequently, a large number of images are usually loaded and the normal values are learned, and then images with values that deviate from them (anomalies) are detected.
Application Example 3. Face Recognition
Face recognition is a technology that extracts and recognizes prominent features from human face images, as the name suggests. It can be used for face identification and grouping. With this technology, it is now possible to use face recognition for security management, as well as identifying the age range of transportation users and store customers.
2-2. Object Detection
Object detection is a technology used to detect the position of a specific object in an image. It is often confused with object recognition, but they are strictly different. Object recognition is a technology used to verify whether the same object as the target exists in the image, and does not specifically detect its position. When these AI image recognition technologies are used in products and services, they are often used in combination.
Object detection and recognition technology is used in a surprisingly wide range of fields, but some alternative examples include its use in automatic driving for identifying signs, pedestrians, and forward vehicles.
Application Example: Image Caption Generation
Image caption generation is a technology that adds captions to situations within images. It is similar to scene recognition mentioned in the image classification section, but it also requires object detection and recognition of their positions. Therefore, object detection technology is also necessary. In addition, it is necessary to output the relationship and situation of objects in natural language, so natural language processing technology is also used. It is expected to be utilized as a spatial awareness aid for visually impaired individuals.
2-3. Segmentation (Region Detection)
In object detection, it is possible to detect the position of objects in an image, but not their shape or contour. In segmentation, learning is performed to detect the contours of specific objects, making it useful in industries such as healthcare that require more accurate object detection, such as shape recognition.
What is segmentation? What can be done using AI segmentation?
2-4. Character Recognition (OCR)
Optical Character Recognition (OCR) is a technology that recognizes characters and symbols written on paper or in images. Due to the fact that characters and symbols have a certain level of regularity, this technology has been used practically for a long time. Recently, the accuracy of recognizing handwritten characters has also improved. By combining this technology with machine translation, there are now apps that can translate restaurant menus scanned with a smartphone camera, and automatically add receipts to household budgets by scanning them with a smartphone camera. Products and services that use this technology have spread not only in business scenes, but also in everyday life.
AI OCR - The Difference and 3 Use Cases Compared to Traditional OCR
3. Examples of Utilizing Image Recognition
Efficiency Improvement of Construction Photo Management
In construction work, a large amount of construction photos are taken to understand the construction status and progress, and various management tasks using images and drawings, such as attaching these images to documents and drawings, are generated. In order to address the 2024 problem, it is urgent to improve business efficiency and productivity through construction DX, and the use of AI image recognition technology in the management of these construction photos can be expected to improve efficiency.
Sorting of Recyclable Waste
Industrial waste must be accurately sorted, including various types of recyclable waste such as PET bottles, steel cans, aluminum cans, and bottles. In the past, this was done manually, but due to the labor-intensive and complex nature of the task, automation is now an urgent issue. While sorting of PET bottles and steel cans has been automated, sorting of bottles by color has not been possible. However, with the advancement of AI image recognition technology, color identification is now possible, making it possible to address the issue of labor shortage.
Expansion of One-Man Train Operation Routes
In order to promote labor-saving and business efficiency, one-man operation is required in railway companies as well. JR Tokai has adopted one-man operation on some routes with a maximum of two cars. By introducing a safety confirmation device incorporating image recognition AI, the safety of one-man operation can be confirmed even on routes operated with four cars, making it possible to expand the routes for one-man operation.
JR Tokai to expand one-man operation from fiscal year 2025, utilizing AI image recognition
4. Summary ~Image Recognition AI Developed by Our Company~
This time, we mainly discussed the mechanism of image recognition and the types of image recognition. These AI technologies for image recognition are currently used in a wide range of fields, and in the future, they will continue to expand and become even more ingrained in people's lives. As if to prove this, the AI development of the companies that request our data annotation services is truly diverse.
Here is just one example, but Human Science would like to introduce the AI image recognition technology that we provide as a data annotation service.
https://www.science.co.jp/annotation/experience/index.html
<Industry Examples>
● Medical Industry: Surgical Support, Diagnostic Support (Object Detection)
https://www.science.co.jp/annotation/industry/medical.html
● Automotive Industry: Autonomous Driving Project 2D/3D (Object Detection)
https://www.science.co.jp/annotation/industry/automobile.html
● IT Industry: Automatic Recognition of Invoices (Character Recognition)
https://www.science.co.jp/annotation/industry/it.html
Not only image recognition, but also a large amount of training data is required for AI machine learning. As mentioned earlier, this means that there is a considerable cost for annotation. However, if you want to reduce the cost of annotation, one effective method is to consider outsourcing the annotation work. At our company, we provide a wide range of services from consultation on annotation to support for creating annotation specifications, creating specifications, and proposing annotation tools. Please feel free to contact us.
5. Human Science's Data Annotation and LLM RAG Data Structuring Agency Service
5-1. Human Science's Data Annotation, LLM RAG Data Structuring Agency Service
At Human Science, we are involved in AI model development projects in various industries such as natural language processing, medical support, automotive, IT, manufacturing, and construction. We have provided over 48 million high-quality training data through direct transactions with many companies, including GAFAM. We can handle various types of annotation, data labeling, and data structuring, from small-scale projects to large-scale projects with a team of 150 data annotators, regardless of industry.
5-2. Resource Management without Using Crowdsourcing
At Human Science, we do not use crowdsourcing and instead directly contract with workers to manage projects. We carefully assess each member's practical experience and evaluations from previous projects to form a team that can perform to the best of their abilities.
5-3. Not only data annotation, but also supports creation and structuring of AI LLM datasets
In addition to data annotation for labeling and identification system AI for data organization, we also support the structuring of document data for the construction of generative AI and LLM RAG. Since our founding, we have been providing manual creation as our main business and service, utilizing our unique know-how of being well-versed in the structure of various documents to offer the best solution.
5-4. Equipped with a security room within the company
At Human Science Co., Ltd., we have a security room that meets the ISMS standards in our Shinjuku office. This allows us to ensure security even for projects that handle highly confidential data. We consider maintaining confidentiality to be extremely important for all of our projects. Even for remote projects, we not only take measures on the hardware side, but also continue to provide security education to our workers. As a result, our information security management system has received high praise from our clients.