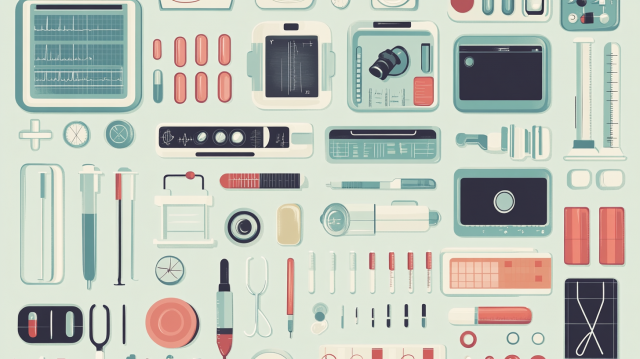
Introduction: The Birth and Potential of Medical AI
In recent years, the evolution of AI technology has led to the development of medical AI in the healthcare field. This is driven by various challenges faced in medical settings. For example, improving diagnostic accuracy, enhancing efficiency in healthcare environments, and addressing the serious shortage of personnel are some of the issues. These challenges require urgent solutions to maintain patient safety and the quality of medical services.
AI holds great potential in addressing these challenges. AI can analyze vast amounts of medical data and detect subtle changes that may be overlooked by the human eye. Additionally, it is expected to alleviate the burden on doctors and nurses through diagnostic support and operational efficiency, contributing to the provision of higher quality medical services. Furthermore, AI is believed to contribute to the realization of personalized medicine and preventive healthcare.
This time, we will explain the history and evolution of AI so far.
- Table of Contents
-
- 1. The Dawn of Medical AI (1950s to 1990s) — The Challenge of Rule-Based Systems
- 1-1. The Encounter of AI and Healthcare
- 1-2. The Emergence of Rule-Based Medical AI Systems
- 2. Growth Period of Medical AI (2000s to 2010s) — The Rise of Machine Learning
- 2-1. The Emergence of Data-Driven AI
- 2-2. Development of Image Diagnosis AI
- 2-3. Utilization of Electronic Medical Records and Natural Language Processing (NLP)
- 3. The Transformation Period of Medical AI (Late 2010s - Present) — The Deep Learning Revolution
- 3-1. Breakthroughs in Image Diagnosis AI
- 3-2. The Evolution of Natural Language Processing and Medical AI
- 3-3. Personalized Medicine
- 3-4. Evolution of Drug Discovery AI
- 4. Summary: The Evolution Story of Medical AI
- 5. Medical Annotation Services in Human Sciences
1. The Dawn of Medical AI (1950s to 1990s) — The Challenge of Rule-Based Systems
1-1. The Encounter of AI and Medicine
The history of AI research began in the 1950s when Alan Turing posed the question, "Can machines think?" The Turing Test remains an important concept today as a benchmark for measuring machine intelligence. Subsequently, in the 1960s and 70s, efforts began to explore the application of AI in the medical field. Early attempts focused on expert systems that described expert knowledge as rules.
However, at that time, AI technology was still in its developmental stages and had not yet been put to practical use in the medical field. Challenges included the limited performance of computers and the difficulty of expressing complex medical knowledge as rules. Additionally, the lack of cooperation from the medical field and distrust towards AI also hindered its practical application.
1-2. The Emergence of Rule-Based Medical AI Systems
In the early days, rule-based medical AI systems were developed. These systems describe expert knowledge as rules and perform diagnosis and treatment based on those rules. Notable examples include the expert system "MYCIN" for diagnosing infectious diseases, developed in the 1970s, and the AI "Internist-1" for internal medicine diagnosis. While these systems demonstrated high accuracy in specific areas, they faced challenges in terms of versatility and flexibility.
However, rule-based medical AI also has its limitations. Challenges include the need to create a vast number of rules and the difficulty of modifying or adding rules. Additionally, there was the issue of a narrow scope, which made it unable to handle complex cases. Furthermore, rule-based systems lack the ability to learn from data, making them unable to adapt to new knowledge or changes.
2. Growth Period of Medical AI (2000s to 2010s) — The Rise of Machine Learning
2-1. The Emergence of Data-Driven AI
From the late 1990s to the 2000s, AI technology known as machine learning began to be applied in the medical field. Machine learning is a technology that allows AI to learn patterns from large amounts of data and make predictions and judgments. Unlike rule-based AI, machine learning enables AI to autonomously learn based on data, allowing for more flexible and advanced processing. As a result, the application range of medical AI has expanded, and it is now expected to contribute to solving various challenges.
The transition from rule-based AI has greatly expanded the possibilities of medical AI. Machine learning can analyze large amounts of data and recognize complex relationships and patterns. This is expected to help detect subtle changes and anomalies that are difficult for humans to identify, leading to more accurate diagnoses and treatment plans. Additionally, machine learning can continuously self-learn and improve its accuracy by incorporating new data.
2-2. Development of Image Diagnosis AI
The advent of machine learning has rapidly advanced image diagnostic AI. From the late 1990s to the 2000s, computer-aided diagnosis (CAD) systems were put into practical use and began to be utilized for the analysis of mammograms and chest X-ray images. These systems assist radiologists in their diagnoses and contribute to the early detection of diseases.
2-3. Utilization of Electronic Medical Records and Natural Language Processing (NLP)
With the spread of electronic medical records, it has become possible to analyze patient data and utilize natural language processing (NLP) for automated patient interviews and diagnostic support. NLP is a technology that understands and processes the language used by humans, and in the medical field, it is expected to be utilized for analyzing medical records and supporting communication with patients. This is believed to reduce the administrative burden on doctors and increase the time they can spend with patients.
The vast amount of data accumulated in electronic medical records serves as valuable training data for medical AI. It is expected that AI will contribute to early disease detection, prediction of treatment effects, and the realization of personalized medicine by analyzing this data. Additionally, AI chatbots utilizing NLP are believed to enhance the efficiency of medical settings by automatically answering patient inquiries and understanding symptoms.
3. The Transformation Period of Medical AI (Late 2010s - Present) — The Deep Learning Revolution
3-1. Breakthroughs in Image Diagnosis AI
In the late 2010s, AI technology known as deep learning gained attention. Deep learning is a type of machine learning that uses multi-layered neural networks to learn more complex patterns. This has achieved higher accuracy in fields such as image recognition and natural language processing, surpassing previous AI technologies. The emergence of deep learning has greatly expanded the possibilities of medical AI and brought about innovative changes in various fields.
The introduction of deep learning has dramatically evolved image diagnostic AI. A technology called CNN (Convolutional Neural Network) has been developed, achieving high accuracy in fields such as skin cancer diagnosis and CT/MRI analysis. CNN can automatically extract and learn the features of images, eliminating the need for humans to manually extract features. This has enabled more efficient and highly accurate image diagnostics.
In 2016, it was reported that Google's AI could diagnose diabetic retinopathy from retinal images, which became a major topic of discussion. This research demonstrated that AI has diagnostic capabilities comparable to those of human specialists, rekindling awareness of the potential of medical AI.
Reference Blog: 3 Examples of Image Diagnosis Using Medical AI
3-2. The Evolution of Natural Language Processing and Medical AI
Natural language processing has also evolved, and with the advent of GPT models, the sophistication of medical record and paper analysis is advancing. GPT models can learn from large amounts of text data and generate natural sentences that resemble human writing, as well as answer questions. As a result, it is expected that in the medical field, tasks such as summarizing and translating medical records, as well as searching and analyzing papers, will become more efficient.
An AI-assisted diagnostic support system has also been developed, enabling interactive diagnostic support with physicians. These systems support physicians' diagnoses by having the AI ask questions and provide advice based on the patient's symptoms and medical record information. This is expected to lead to improved diagnostic accuracy and reduced burden on physicians.
Reference Blog: The Cutting Edge of Medical × LLM: 6 Use Cases
3-3. Personalized Medicine
Personalized medicine utilizing genetic data is also beginning. AI can analyze patients' genetic information and suggest the most suitable treatments and medications. This makes it possible to provide more effective treatments with fewer side effects. Personalized medicine aims to provide optimal healthcare tailored to each patient's constitution and condition.
Furthermore, not only through genetic analysis but also by using wearable devices to collect data in real-time and having AI analyze it, we aim to assist in detecting abnormal behavior patterns and early disease detection. AI is expected to play a role in dynamically adjusting treatment plans while monitoring the changes in individual patients' lifestyles and health conditions by combining these diverse data.
3-4. Evolution of Drug Discovery AI
Efforts to utilize AI in the field of drug discovery are also beginning. AI-driven drug discovery, such as protein structure prediction by AlphaFold, is accelerating. AlphaFold is an AI model that can predict protein structures with high accuracy, and it is expected to significantly enhance the speed of new drug development.
The era has arrived where AI can discover new drug candidates in a short period of time. AI can analyze large amounts of compound data and biological data to predict efficacy and side effects. This has made it possible to significantly shorten the duration of new drug development, which traditionally took several years.
4. Summary: The Evolution Story of Medical AI
Medical AI has evolved from the rule-based dawn to machine learning and then to deep learning. With its application advancing in various fields such as image diagnosis, natural language processing, robot-assisted surgery, and drug discovery, it holds the potential to change the future of healthcare.
With the advancement of AI technology, ethical and legal challenges are also emerging. However, research and development are being pursued with the aim of a future where AI and doctors coexist to provide higher quality medical care. Medical AI is still an evolving field, and it is believed that its potential will further expand through future technological innovations and social discussions.
Reference Blog: Current Status and Future Prospects of Medical AI
At Human Science, we have extensive experience in medical annotations that require high expertise, such as endoscopic surgery images, chest X-ray images, and abdominal CT images. Our strengths include the creation of reliable annotation data under the supervision of physicians, the accumulation of know-how through trials, and close collaboration with our clients. If you would like to discuss the selection and implementation strategies for optimal AI systems tailored to the scale and characteristics of medical institutions, or if you are looking for specialized support, please feel free to contact us.
5. Medical Annotation Services in Human Sciences
●Extensive annotation experience in medical imaging
Our company has extensive experience in medical image annotation that requires skill transfer, such as surgical images and MRI images, which are of high difficulty and specialization. In addition to experienced project managers in medical image annotation projects, we also have many skilled workers, ensuring high-quality annotation even for projects that are complex, specialized, and require skill transfer.
●Support for physician supervision and annotations by physicians
It can be concerning to have only general workers perform all tasks. In such cases, we receive requests for supervision by a physician for certain check tasks. To meet these requests, we have further strengthened our physician supervision system, allowing us to handle more complex annotations. Additionally, even if you request annotations by physicians instead of general workers, our project managers will provide comprehensive management services, ensuring resource allocation, quality, and progress management.
Resource management without crowdsourcing
At Human Science, we do not use crowdsourcing. Instead, projects are handled by personnel who are contracted with us directly. Based on a solid understanding of each member's practical experience and their evaluations from previous projects, we form teams that can deliver maximum performance.
Secure room available on-site
Within our Shinjuku office at Human Science, we have secure rooms that meet ISMS standards. Therefore, we can guarantee security, even for projects that include highly confidential data. We consider the preservation of confidentiality to be extremely important for all projects. When working remotely as well, our information security management system has received high praise from clients, because not only do we implement hardware measures, we continuously provide security training to our personnel.
Support for not just annotation, but the creation and structuring of generative AI LLM datasets
In addition to labeling for data organization and annotation for identification-based AI systems, Human Science also supports the structuring of document data for generative AI and LLM RAG construction. Since our founding, our primary business has been in manual production, and we can leverage our deep knowledge of various document structures to provide you with optimal solutions.