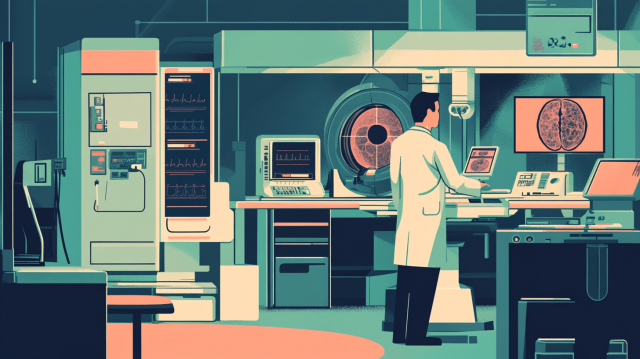
In Japan's medical field, the shortage of healthcare workers is becoming serious amid a declining birthrate and an aging population, and the disparity in medical care between regions is also widening. As solutions to these structural problems are sought, the development and implementation of AI can be considered an important solution expected to contribute to the efficiency of medical practices, the improvement of the quality of care, and the enhancement of medical access in remote areas.
In recent years, AI in the medical field has made remarkable advancements. Demonstration experiments are being conducted on initiatives such as patient interviews and predicting disease risks, as well as medical AI tools that streamline administrative tasks, with great expectations for reducing the burden on the field and improving the quality of medical services.
Among them, image recognition AI (AI that supports image diagnosis) can be said to be a technology that symbolizes the transformation in the medical field. AI that has learned specialized judgment criteria is expected to improve the efficiency and accuracy of image diagnosis. This will pave the way for solving a wide range of issues, including the streamlining of operations in medical settings, standardization of diagnostic accuracy, and even the elimination of regional disparities in healthcare. On the other hand, such AI requires advanced specialized knowledge, so active involvement from physicians is necessary.
This time, we will explain the importance of physician checks and their role in improving the quality of training data in image recognition AI in the medical field. (In this article, we will refer to AI systems equipped with image recognition technology used in the medical field as "medical AI.")
- Table of Contents
-
- 1. The Reliability of Training Data Essential for AI Development
- 2. Reliability Required for Medical AI
- 3. The Role of Physicians and Challenges of Efficiency in Medical AI Development
- 4. Improving Accuracy and Efficient Creation of Training Data through Physician Checks
- 5. Key Points for Implementing Physician Checks to Enhance the Quality of Medical AI
- 6. Summary
- 7. Medical Annotation Services in Human Sciences
1. The Reliability of Training Data Essential for AI Development
Generally, the performance of image recognition AI is greatly influenced by the quality of the vast amount of training data that supports it. No matter how advanced the algorithms are, if the reliability of the training data is low, it is difficult to achieve the expected performance.
The reliability of training data refers to the correctness and consistency of the reasoning behind the labels assigned to the data. In particularly specialized fields, the involvement of experts in that field is required for the creation of training data. By ensuring high reliability of the training data, the learning efficiency of AI models is enhanced, directly leading to improved accuracy.
The reliability of such training data becomes particularly evident in the field of AI in healthcare.
2. Reliability Required for Medical AI
In the development of medical AI, a higher level of reliability is required than in other fields. This is because AI's decisions can directly impact patient diagnoses and treatment plans, and may sometimes involve life-and-death decisions.
Creating training data for medical AI often requires specialized knowledge, making it more challenging to prepare appropriate data compared to other fields. Additionally, if there are incorrect labels or biased data, it can directly impact the diagnostic accuracy of the AI, so it is essential to maintain accuracy throughout the entire data creation process.
Furthermore, there are security challenges in the development of medical AI. If patient personal information is included in the medical images used as training data, careful attention is required in handling it, such as applying anonymization processes. Additionally, considerations regarding data protection, the establishment of legal frameworks, and ethical perspectives are important. By taking these factors into account, it leads to the realization of medical AI systems that possess high safety and reliability.
3. The Role of Physicians and Efficiency in Medical AI Development
Creating training data for medical images often requires specialized knowledge and experience, making it difficult for general workers to perform appropriate labeling. For example, when identifying the positions of various organs and instruments in chest X-ray images, it is necessary to understand the basic interpretation techniques of chest X-rays and the instruments involved. Furthermore, in development cases where accurate identification of lesion signs and precise labeling of their location and extent is required, the knowledge of a specialist is essential.
So, can we say that having doctors themselves create the training data is optimal for such specialized tasks? Certainly, if doctors can create the training data themselves, we can ensure high-quality training data. However, on the other hand, it is often difficult for them to allocate sufficient time for this work, as they can only do it outside of their regular working hours or by dedicating a portion of their working hours. This would require an enormous number of days to create the necessary amount of training data. Therefore, the delivery time would also be longer compared to general timelines. This becomes a bottleneck in AI development, which demands speed. Additionally, there are challenges such as higher costs compared to general workers. In typical AI development, it is possible to efficiently mobilize general workers by utilizing outsourcing services like annotation vendors to create large amounts of training data in a short period. However, this is not the case in medical AI development. Nevertheless, direct involvement of doctors is essential to enhance the reliability of training data in medical AI development.
One method to solve such challenges is the system of "physician check," where doctors are involved specifically in the checking process rather than creating the training data. This allows for quality assurance while reducing the burden on physicians.
4. Improving Accuracy and Efficient Creation of Training Data through Physician Checks
To briefly describe the process of creating training data that incorporates physician checks, first, general workers perform the entire labeling task, and then physicians review all of them and provide correction instructions as needed. This process helps to solve the following issues.
Creation of Training Data with Short Turnaround
Since the creation of training data is performed by general workers, it can be completed in a significantly shorter time compared to when a physician creates it during their practical work.
●Improvement of Work Efficiency
Compared to when a doctor creates training data from scratch, significant cost reductions can be expected by having general workers take on the tasks. Additionally, by specializing the doctor's work in the review process, the workflow becomes more efficient.
●Ensuring AI Reliability
After labeling by general workers, doctors will review the data and provide correction instructions, ensuring the reliability of the training data. As a result, the validity as a medical AI is supported, and the acceptance and reliability from clinical settings are enhanced.
The process of creating training data with physician checks is an effective approach to improve the accuracy and reliability of AI suitable for the medical industry while also reducing the burden on physicians. However, there is a possibility that the number of correction instructions from physicians may increase due to a lack of quality understanding by general workers, which presents new challenges. Therefore, it is necessary to prepare and establish an appropriate system.
5. Key Points for Implementing Physician Checks to Enhance the Quality of Medical AI
When introducing physician checks in the creation of training data for medical AI, several important points should be considered. By properly preparing and operating these elements, an effective physician check system and process can be established.
●Matching AI Development with Medical Specialties:
It is important to secure physicians from the appropriate specialties according to the data targeted for development and the objectives of medical AI.
●Team Structure for Doctor Check:
It is necessary to secure an appropriate number of doctors and adjust the working schedule considering the doctors' working hours and their primary duties, in order to establish a system that allows for smooth checking operations within the work period.
●Lecture on Medical Knowledge for General Workers:
It is important to educate general workers on the minimum medical knowledge required for their tasks. Additionally, regular information sharing and the maintenance of manuals help to reduce variations in work quality. If the quality of work performed by general workers is poor, the amount of feedback and corrections from doctors will increase, leading to a loss of many benefits of doctor checks. Therefore, it is essential to organize the key points of the lectures to align with the requirements of the development side and to utilize workers who have extensive experience in creating training data in the medical field.
●Communication platform between doctors and the development team:
When there is a discrepancy between the medical judgment of the physician and the judgment of the development side, effective communication to resolve that discrepancy is key. To facilitate smooth communication, a dedicated PM who acts as a bridge between both parties is necessary. The PM prevents discrepancies in judgment by sharing information such as regular meetings with both sides and comments from the physician's review.
●Selection of Annotation Tools:
We select tools that doctors can intuitively operate and easily record necessary information. It is important that doctors can proceed with their work without burden and that the tools facilitate the easy collection of doctors' check records.
●Policy for responding when there is a difference in judgment among physicians:
We establish methods and criteria for resolution in case of differing judgments in advance, ensuring consistency in development policies and data.
●Security measures for training data:
We establish strict rules regarding the protection of personal information, prevention of data leaks, and handling of anonymized processed information, and implement appropriate security measures such as data encryption and access restrictions.
6. Summary
So far, we have explained the importance of physician checks in the creation of training data for medical AI and the key points for its implementation. However, it is often unrealistic for companies developing AI to handle the creation of training data and physician checks in-house, as it goes beyond the scope of their core development activities.
In such cases, if you can request external vendors who have extensive experience in creating training data for medical AI and can also secure resources for physician checks, it will be possible to focus on the original AI development tasks.
4. Medical Annotation Services in Human Sciences
●Extensive annotation experience in medical imaging
Our company has extensive experience in medical image annotation that requires skill transfer, such as surgical images and MRI images, which are of high difficulty and specialization. In addition to experienced project managers in medical image annotation projects, we also have many skilled workers, ensuring high-quality annotation even for projects that are complex, specialized, and require skill transfer.
●Support for physician supervision and annotations by physicians
It can be concerning to have only general workers perform all tasks. In such cases, we receive requests for supervision by a physician for certain check tasks. To meet these requests, we have further strengthened our physician supervision system, allowing us to handle more complex annotations. Additionally, even if you request annotations by physicians instead of general workers, our project managers will provide comprehensive management services, ensuring resource allocation, quality, and progress management.
●Resource management without using crowdsourcing
At Human Science, we do not use crowdsourcing; instead, we advance projects with personnel directly contracted by our company. We form teams that can deliver maximum performance based on a solid understanding of each member's practical experience and their evaluations from previous projects.
●Equipped with a security room in-house
At Human Science, we have a security room that meets ISMS standards within our Shinjuku office. Therefore, we can ensure security even for projects that handle highly confidential data. We consider the protection of confidentiality to be extremely important for all projects. Even for remote projects, our information security management system has received high praise from our clients, as we not only implement hardware measures but also continuously provide security training to our personnel.
Supports not only annotation but also the creation and structuring of generative AI LLM datasets.
In addition to labeling and annotation for identification systems for data organization, we also support the structuring of document data for the construction of generative AI and LLM RAG. Since our founding, we have been engaged in manual production as a primary business and service, leveraging our unique know-how gained from a deep understanding of various document structures to provide optimal solutions.