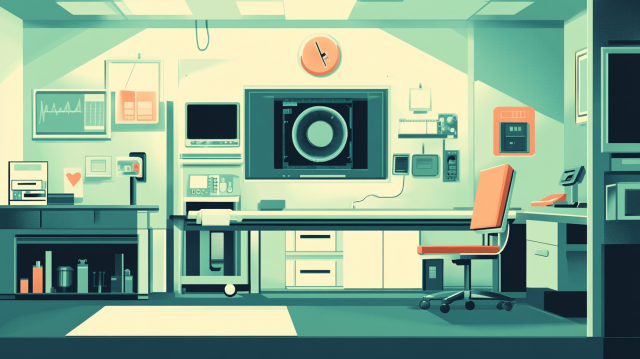
The use of AI is advancing in the medical industry. AI has the ability to analyze vast amounts of data quickly and accurately, and is being recognized as a new means to improve the quality of healthcare. As a result, there are expectations for rapid responses to patients, improved treatment accuracy, and a reduction in the burden on healthcare professionals. Among these, the field of medical imaging is particularly noted as an area benefiting from AI, and research and development are actively progressing.
Medical AI in imaging diagnosis analyzes medical images such as X-rays, CT scans, and MRIs to support early detection and diagnosis of diseases. For example, it is beginning to be utilized in various areas such as tumor detection, fracture confirmation, and risk assessment for pneumonia and heart disease. By streamlining tasks that specialists traditionally took time to perform and reducing the chances of oversight, it is expected to provide significant benefits not only to healthcare professionals but also to patients. In this article, we will clearly explain how medical AI is being utilized in the field of imaging diagnosis, highlighting specific case studies.
- Table of Contents
1. The Penetration of Medical AI in Imaging Diagnosis
The advancement of medical AI is attracting significant attention in the field of imaging diagnostics, and efforts are underway to implement it in various medical settings, such as radiology and endoscopic examinations. Imaging diagnostic AI refers to the technology that analyzes medical images like X-rays, CT scans, and MRIs to assist in the early detection of lesions and improve diagnostic accuracy. The evolution of this technology is not only expected to reduce the burden on healthcare professionals and enhance the speed of diagnosis but also to provide solutions to challenges such as labor shortages and regional disparities that have plagued traditional healthcare systems.
One of the strengths of AI lies in its exceptional data processing capabilities. AI, which can analyze vast amounts of medical data in a short time and extract patterns and features with high precision, supports diagnoses that may be overlooked by the human eye. In particular, AI using deep learning plays a crucial role in quickly and accurately recognizing patterns of lesions and abnormalities, serving as an important aid in diagnosis. For example, AI that automatically detects lesions from chest X-rays and breast mammography images analyzes large volumes of image data rapidly and with high accuracy, leading to earlier detection of lesions and improved diagnostic accuracy. This is expected to contribute to better patient prognosis and improved quality of healthcare.
2. Three Examples of Utilizing Image Diagnosis with Medical AI
Estimation of Lung Function by Chest X-ray Images / Osaka Metropolitan University
According to research announced by Osaka Metropolitan University in July 2024, a model has been successfully developed to estimate lung function with high accuracy from chest X-ray images using AI.
Data Collection and Learning:
•Collected 141,734 chest X-rays from 5 domestic facilities between 2003 and 2021
•Conducted training and validation of AI models at 3 facilities
•Conducted external testing at 2 facilities
Achieving High-Precision Estimation:
• Estimating key indicators of pulmonary function tests, such as Forced Vital Capacity*1 and Forced Expiratory Volume in 1 second*2
• Comparing the AI model's estimates with actual measurements from pulmonary function tests, achieving a very high rate of agreement
Expected Applications:
•Application for patients who have difficulty undergoing standard pulmonary function tests, such as dementia patients and children.
•An alternative method when standard tests cannot be conducted during infectious disease outbreaks like COVID-19.
•Enables estimation of pulmonary function using only chest X-rays, contributing to the efficiency of testing.
*1 "Vital Capacity": The amount of air that can be inhaled to the maximum and then forcefully exhaled. It is an indicator that reflects the size and elasticity of the lungs.
*2 "Forced Expiratory Volume in 1 Second": The amount of air that can be exhaled in one second after taking a maximum inhalation. It is an indicator that reflects the width of the airways and the force of exhalation.
Reference Link: AI Estimates Lung Function! Development of a High-Precision Model Using Chest X-Ray Images
Breast cancer detection using mammography images / Cancer Research Institute, Ariake Hospital and Google
In a joint research project between the National Cancer Center Hospital East and Google, the effectiveness of AI for the automatic detection of breast cancer from mammography images was validated in Japanese women.
Data Collection and Analysis:
•Used mammography images of approximately 20,000 Japanese women taken between 2007 and 2020
•Implemented appropriate anonymization processes to protect personal information
How to Operate AI:
• Utilize the AI model as a "second reader"
• A second reader will verify only if the AI's opinion does not match that of the first interpreting physician
Performance of AI Models:
• Accuracy of breast cancer detection improved by 7.6% compared to traditional methods (double reading method by different radiologists)
• Improved consistency of readings between different doctors (the kappa coefficient, a statistical measure of agreement among multiple evaluators, improved from an average kappa of 0.65 to 0.74)
Detection of Kidney Abnormalities by Abdominal CT Images / NTT Data and Miyazaki University Medical School Hospital
NTT Data and Miyazaki University Medical School Hospital developed and validated an AI that automatically detects abnormalities in the kidneys from abdominal CT images.
AI Training Data:
•Trained on abdominal CT images of approximately 5,000 American patients (about 110,000 images)
Features:
• Capable of detecting not only specific diseases (such as kidney cancer, kidney stones, hydronephrosis) but also various organ abnormalities (such as cysts, tumors)
• Independent of CT imaging conditions such as CT manufacturers and the presence of contrast agents
Results of the Demonstration Experiment:
•Validated with data from 700 patients at Miyazaki University Hospital
•Accuracy of kidney cancer diagnosis: Correct rate 89.00%, Sensitivity 82.00% *1, Specificity 95.00% *2, Precision 94.60%
*1 "Sensitivity": Defined as "the probability of correctly identifying something that should be classified as positive," indicating that a higher value signifies fewer missed cases.
*2 "Specificity": Defined as "the probability of correctly identifying something that should be classified as negative," meaning that a higher value indicates fewer false positives (incorrectly classifying a healthy person as sick), and it is an important indicator in diagnoses with low prevalence.
3. Summary
The role of medical AI in image diagnosis is becoming increasingly important. As demonstrated by the cases introduced in this article, AI has the potential to assist in the early detection of diseases and improve diagnostic accuracy through the analysis of medical images, thereby reducing the burden on healthcare professionals. This is expected to lead not only to prompt responses for patients and improved prognoses but also to an overall enhancement in the quality of healthcare.
On the other hand, in order to maximize the performance of AI, it is important to create high-quality training data (annotation data). However, it is a challenge for medical device manufacturers and medical institutions to create such training data in-house due to resource and cost constraints. Careful preparation is required, such as selecting external vendors who understand the specialized requirements unique to the medical field and conducting a Proof of Concept (PoC) before scaling.
At Human Science, we have extensive experience in medical annotations that require high expertise, such as endoscopic surgery images, chest X-ray images, and abdominal CT images. Our strengths include the creation of reliable annotation data under the supervision of physicians, the accumulation of know-how through trials, and close collaboration with our clients. If you would like to discuss the development and implementation strategies of optimal AI systems tailored to the scale and characteristics of medical institutions, or if you are looking for specialized support, please feel free to contact us.
4. Medical Annotation Services in Human Sciences
●Extensive annotation experience in medical imaging
Our company has extensive experience in medical image annotation that requires skill transfer, such as surgical images and MRI images, which are of high difficulty and specialization. In addition to experienced project managers in medical image annotation projects, we also have many skilled workers, ensuring high-quality annotation even for projects that are complex, specialized, and require skill transfer.
●Support for physician supervision and annotations by physicians
It can be concerning to have only general workers perform all tasks. In such cases, we receive requests for supervision by a physician for certain check tasks. To meet these requests, we have further strengthened our physician supervision system, allowing us to handle more complex annotations. Additionally, even if you request annotations by physicians instead of general workers, our project managers will provide comprehensive management services, ensuring resource allocation, quality, and progress management.
Resource management without crowdsourcing
At Human Science, we do not use crowdsourcing. Instead, projects are handled by personnel who are contracted with us directly. Based on a solid understanding of each member's practical experience and their evaluations from previous projects, we form teams that can deliver maximum performance.
Secure room available on-site
Within our Shinjuku office at Human Science, we have secure rooms that meet ISMS standards. Therefore, we can guarantee security, even for projects that include highly confidential data. We consider the preservation of confidentiality to be extremely important for all projects. When working remotely as well, our information security management system has received high praise from clients, because not only do we implement hardware measures, we continuously provide security training to our personnel.
Support for not just annotation, but the creation and structuring of generative AI LLM datasets
In addition to labeling for data organization and annotation for identification-based AI systems, Human Science also supports the structuring of document data for generative AI and LLM RAG construction. Since our founding, our primary business has been in manual production, and we can leverage our deep knowledge of various document structures to provide you with optimal solutions.