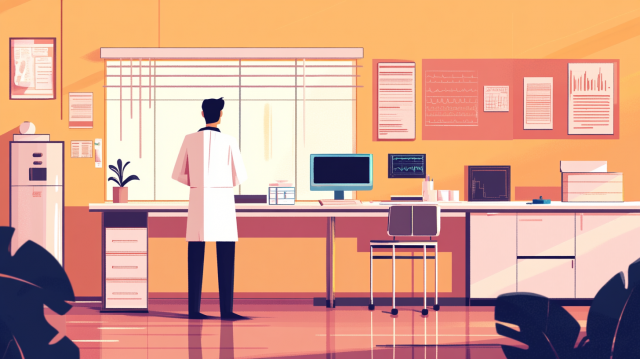
The use of AI in the medical field has moved beyond the experimental stage and is now producing concrete results. However, there are legitimate concerns about whether it can truly improve operations in medical settings and whether the benefits will justify the implementation costs.
In fact, the introduction of AI in healthcare requires careful consideration and meticulous preparation. Particularly important are the quality of medical data and the expertise in annotation.
This article provides a detailed explanation of the specific benefits of utilizing AI in the medical field, the challenges of implementation, and practical steps for introduction, along with real-life examples. We hope this serves as a concrete guideline for those considering the introduction of medical AI.
- Table of Contents
-
- 1. What can AI do in the medical field?
- 1-1. Image Diagnosis Support AI
- 1-2. Triage Support AI in Emergency Medical Care
- 1-3. Automatic Voice Input and Medical Record Generation
- 1-4. Optimization of Drug Administration Plans
- 3 Benefits of AI Utilization in Healthcare
- 2-1. Improvement of Diagnostic Accuracy and Speed
- 2-2. Business Efficiency Improvement
- 2-3. Standardization of Medical Care and Regional Medical Support
- 3. Three Challenges of AI Utilization in Healthcare
- 3-1. Introduction Cost Issues
- 3-2. Technical Limitations
- 3-3. Ethical and Psychological Resistance
- 4. Steps for Implementing AI Systems in Healthcare
- 4-1. Current Situation Analysis and Requirements Definition Before Implementation
- 4-2. Data Collection and Annotation
- 4-3. Execution of PoC
- 4-4. Appropriate Vendor Selection
- 5. Summary
1. What can AI do in the medical field?
AI in the medical field is becoming established as a tool to support physicians' judgments and enhance the quality of healthcare. Particularly in the areas of image analysis and clinical support, practical results have already emerged.
>>Related Articles: Challenges and Solutions in Medical AI Annotation - Utilizing Outsourcing Vendors with Expertise
1-1. Image Diagnosis Support AI
Image diagnostic support AI functions as a "second eye" for physicians, particularly in the field of radiology. In the recognition and analysis of medical images, AI provides specific support as follows.
●Automatic detection of lesion sites and localization
●Measurement of tumor size changes over time
●Detection of deviations from normal ranges
For example, in the interpretation of chest CT images, image recognition AI can automatically measure the location and size of pulmonary nodules and quantitatively show temporal changes.
By utilizing image recognition AI, doctors are now able to spend more time observing detailed cases. A specific example of its application is in breast cancer screening. In mammography interpretation, AI pre-detects subtle calcifications and potential tumors, highlighting areas that require the attention of specialists.
This not only contributes to preventing oversight but also helps reduce reading time.
What is particularly noteworthy is that AI can analyze multiple inspection data by combining them. For example, by referencing CT and MRI images simultaneously, it has become possible to identify lesions more accurately.
In addition, AI can process large amounts of pathology image data in a short time. For example, in the search for lymph node metastasis, AI performs preliminary screening, allowing pathologists to focus their observations on areas that require more detailed examination.
If you are considering AI development, we recommend utilizing AI Market, which provides support for selecting AI development companies. They will introduce companies that are strong in specific industries such as healthcare and specific technologies like image recognition.
Reference Blog: 3 Examples of Image Diagnosis Using Medical AI
1-2. Triage Support AI in Emergency Medical Care
In emergency medical settings, assessing the severity of patients and determining the appropriate treatment priorities is extremely important. Triage AI comprehensively analyzes the symptoms, vital signs, and test data of patients transported to the emergency department to assist in determining the urgency of care.
A specific example of application is the initial evaluation of suspected stroke patients. AI analyzes the patient's symptom data and imaging results, contributing to a reduction in the time to start treatment.
In particular, its usefulness has been confirmed as a support tool for initial judgment in situations where specialists cannot respond immediately, such as during nights and holidays.
1-3. Automatic Voice Input and Medical Record Generation
Record creation in medical settings occupies a significant portion of the working hours of doctors and nurses. Traditionally, creating medical records required doctors to input data into electronic medical records after examinations.
The current AI voice input system can transcribe the speech of doctors in real-time during examinations and record it in electronic medical records in the appropriate format. Notably, it can accurately recognize medical-specific terminology and abbreviations and record them in a structured format.
A specific example of utilization is its application in emergency outpatient care. Doctors can verbally record findings and treatment details while examining patients. This improves the efficiency of medical care and the accuracy of records.
Reference Blog: The Cutting Edge of Medical × LLM: 6 Use Cases
1-4. Optimization of Drug Administration Plans
Optimizing drug administration plans is an extremely important area for balancing patient safety and treatment effectiveness. AI-driven optimization of administration plans achieves more precise medication management while considering patient individuality.
Optimization of dosing plans in chemotherapy is a representative application. AI algorithms comprehensively analyze elements such as the following to propose the optimal dosage and schedule for each individual patient.
● Patient's physiological parameters
● Genetic information
● Past treatment responsiveness
For example, in the administration of antibiotics to dialysis patients, research is being conducted on the optimal timing of administration considering the dialysis schedule and pharmacokinetics.
3 Benefits of AI Utilization in Healthcare
The use of AI in the medical field has become possible to provide solutions to the structural challenges faced by medical settings due to advancements in deep learning technology and big data analysis.
2-1. Improvement of Diagnostic Accuracy and Speed
Image diagnostic support systems utilizing deep learning have shown remarkable results, particularly in the field of radiology. Improvements have been reported compared to traditional reading processes, including the following:
●Optimization of reading time: AI pre-screening reduces the reading time per case for radiologists
●Improvement in diagnostic accuracy: Deep learning models can detect early-stage lesions that are often overlooked in traditional visual diagnoses with high precision
●Standardization of diagnosis: Reduces variability in diagnoses based on the years of experience and expertise of physicians
A distinctive feature of medical AI is its ability to learn continuously. The system can improve its accuracy by learning from daily clinical data.
2-2. Business Efficiency Improvement
By utilizing natural language processing (NLP) technology for voice input systems and optimizing business processes through machine learning, it has become possible to significantly reduce the workload of healthcare professionals.
For example, AI voice input integrated with electronic medical record systems brings about specific improvements compared to traditional manual input as follows:
●Efficiency in creating medical records: Time previously spent on manual record input can now be dedicated to actual patient care.
●Improvement in data quality: Real-time checks for omissions in symptom descriptions and inappropriate terminology usage during input.
It is noteworthy that these AI systems are not just labor-saving tools, but also contribute to the qualitative improvement of healthcare. They can also support the development of optimal treatment plans by integratively analyzing individual patient medical data.
2-3. Standardization of Medical Care and Regional Medical Support
The concentration of specialists in urban areas has resulted in limited access to advanced specialized medical care in rural areas. AI-powered telemedicine support systems provide solutions to this uneven distribution of medical resources.
The introduction of Clinical Decision Support Systems (CDSS) enables general practitioners in the region to receive diagnostic support at the level of specialists.
For example, in the field of dermatology, even in medical institutions without specialists, the use of AI-based image analysis systems has made it possible to detect important lesions such as melanoma at an early stage.
Reference Blog: Advantages and Disadvantages of Medical AI
3. Three Challenges of AI Utilization in Healthcare
The introduction of AI in the medical field presents complex challenges from technical, economic, and ethical perspectives. Properly understanding and addressing these challenges is essential for the implementation of sustainable AI systems.
3-1. The Issue of Implementation Costs
The implementation of medical AI systems incurs not only initial investment but also ongoing operational and maintenance costs. An important investment item that must be considered is the preparation of high-quality training data.
For example, annotating medical data necessary for building an AI system that performs image recognition requires personnel with specialized medical knowledge, and compared to general data labeling, costs can reach 5 to 10 times higher.
In terms of operations, in addition to the operational costs of medical AI, additional investments may also be required for integration with existing electronic medical record systems and security measures.
From the perspective of ROI (Return on Investment), it is important to evaluate the indirect benefits of long-term improvements in healthcare quality and operational efficiency rather than focusing solely on short-term revenue enhancement.
3-2. Technical Limitations
The technical limitations of medical AI are primarily related to the quality and quantity of data, as well as the interpretability of algorithms. A particularly serious challenge is the imbalance of training data caused by the specificity of medical data.
Data on rare diseases and emergency cases is inevitably limited, which tends to introduce bias in the performance of AI models.
Furthermore, in the development of medical AI, the explainability of the algorithm's decision-making basis is particularly important. It is important to note that current deep learning models, while demonstrating high accuracy, tend to have a characteristic of becoming black boxes in their decision-making processes.
3-3. Ethical and Psychological Resistance
The ethical challenges in the implementation of medical AI range from protecting patient privacy, determining responsibility for AI diagnoses, to maintaining the humanity of healthcare.
What is particularly important is the response to concerns that AI-driven decisions may threaten the expertise of healthcare professionals.
Psychological resistance on the part of patients is also an issue that cannot be overlooked. Healthcare is fundamentally a service based on trust between individuals, and there is concern that the involvement of AI may undermine this trust.
To achieve ethical AI, it is essential to establish clear guidelines regarding the handling of medical data.
4. Steps for Implementing AI Systems in Healthcare
The introduction of AI systems to medical institutions requires careful planning and a phased approach. In particular, the integration with existing medical systems and the implementation process that takes into account the specificity of medical data are crucial.
4-1. Current Situation Analysis and Requirements Definition Before Implementation
When introducing AI systems, the most important thing is to accurately understand the current situation and challenges of medical institutions. The integration of existing systems and AI in medical institutions requires careful consideration from a technical perspective.
As a specific analysis method, we will visualize the processing flow of the current system and simulate the business processes after the introduction of the AI system. This will allow us to identify potential issues in advance and take measures accordingly.
The success of implementing AI systems requires the formation of a team with diverse expertise. We will establish a communication system within the team and manage progress and solve issues through regular meetings.
4-2. Data Collection and Annotation
The performance of image recognition AI directly depends on the quality of the training data. Especially in the medical field, the accuracy of the data and professional interpretation are essential, and the quality of annotation (accurate labeling of data) greatly influences the reliability of the AI system.
Medical data annotation requires specialized judgment that goes beyond mere labeling. Particularly important are the training of the staff performing the annotation and quality control. To ensure data quality, the following system improvements are recommended.
● Establishment of a mutual check system by multiple staff members (including specialists)
● Regular alignment of evaluation criteria
● Standardization of evaluation criteria through case review meetings
● Regular audits of annotation results
Investing sufficient time and resources in the data collection and annotation stages leads to the success of the project.
Reference Blogs:
Enhancing the Reliability of Medical AI: Quality and Security Management of Training Data
The Key to Improving Medical AI Reliability: Training Data and Physician Review
4-3. Execution of PoC
Before the full-scale implementation of the AI system, it is essential to conduct a Proof of Concept (PoC) within a limited scope. The main objectives of this phase are to verify the effectiveness of the system and to identify challenges in actual operations at an early stage.
In the implementation of the PoC, for example, we select specific medical departments or testing divisions and begin trial operations in an actual clinical environment.
In the evaluation of operational aspects, we will analyze in detail the impact on the workflow of healthcare professionals. Particularly noteworthy are the changes in work efficiency due to the introduction of AI systems and their impact on the decision-making processes of healthcare professionals.
For example, in addition to quantitative indicators such as the reduction of reading time and improvement of diagnostic accuracy, we also collect detailed feedback from the field regarding the usability and operability of the system.
4-4. Appropriate Vendor Selection
Selecting a medical AI vendor is a critical decision that can determine the success or failure of a project. Especially in the medical field, a deep understanding of medical-specific requirements and challenges is essential, in addition to mere technical capabilities.
In terms of technical evaluation, the explainability of AI models is particularly important. In the medical field, it is essential for healthcare professionals to understand and verify the rationale behind AI decisions.
Additionally, a proven track record of smooth integration with existing medical systems is also an important consideration. In evaluating data management systems, we will thoroughly check the understanding and compliance with relevant regulations such as the Personal Information Protection Act and the Next-Generation Medical Infrastructure Act.
Reference Blog: Challenges and Solutions in Medical AI Annotation - Utilizing Outsourcing Vendors with Expertise
5. Summary
The use of AI in the medical field is demonstrating concrete results across a wide range of areas, from improving diagnostic accuracy to enhancing operational efficiency.
However, the implementation of AI systems requires proper planning and a phased approach. Particularly important are the quality management of medical data and the expertise in annotation. It is essential to understand the specific requirements of the medical field and to conduct thorough preparations, from selecting the appropriate vendors to implementing the Proof of Concept (PoC).
We recommend taking concrete steps through consultations with experts regarding the selection of the optimal AI system and the development of implementation strategies tailored to the scale and characteristics of medical institutions.
Human Science has extensive experience in medical annotations that require high levels of difficulty and expertise, such as surgical images and MRI images. Even in fields with high levels of difficulty and specialization, our project managers have closely collaborated with clients, repeatedly conducting trials to acquire know-how. If you are interested in the medical field or are looking for specialized support in AI development, please feel free to contact us.
>>Human Science Medical Industry Annotation Outsourcing Service - Overview Here