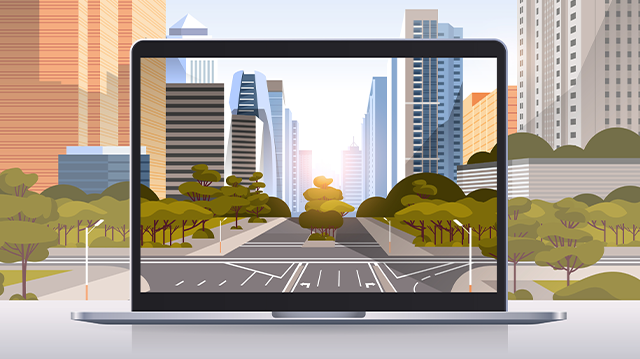
It has been over 10 years since AI utilizing deep learning gained attention by winning an image recognition competition in 2012. AI, known as "discriminative systems," which are specialized for specific tasks, has reached maturity and is still being developed and implemented in various fields, such as image recognition in the medical sector.
While discriminative systems are certainly important, recent developments in generative AI, such as ChatGPT, also often require a large amount of "training data" to teach the AI. The process of creating this training data is called annotation. In this article, we will thoroughly explain the methods of annotation by introducing articles from our company's blog that we have published so far.
- Table of Contents
1. What is Annotation
In order for AI to perform tasks, learning is necessary. In addition to the previously mentioned "supervised learning," there are also "unsupervised learning" and "semi-supervised learning" methods. Supervised learning is adopted in many identification-based AIs. The role of the teacher here is taken on by the training data. For example, in car identification, the task of enclosing the car in the image with a rectangle (bounding box) and tagging it with the label "car" is what constitutes the training data. This task is called annotation (or data labeling). By training the AI with the created training data, the AI learns the characteristics of cars and can identify them in new images that have not been annotated.
Here is the blog about annotations and training data
>What is Annotation
>What is the difference between AI training data and learning data? A clear explanation!
Annotation originally means "to add notes" and refers to the act of marking relevant parts of a text with underlines or marks to provide additional information or comments. The process of creating training data involves similar tasks, which is why the term "annotation work = creating training data" has come to be used. Additionally, labeling, which refers to attaching price tags to products, is a similar task in that it involves assigning metadata, such as price, to the product. Therefore, data labeling has also come to mean the creation of training data. In Japan, annotation is common, while in the United States, it is often referred to as data labeling.
2. Types of Annotations
As mentioned earlier, for image data, we mark the target to be identified using bounding boxes and other methods. In addition to bounding boxes, there are annotations such as segmentation and key points. For simple object detection like cars and people, bounding boxes are sufficient, while for more complex shapes, such as lesions in body tissues, segmentation is necessary to accurately identify them. The content that can be learned by AI also varies depending on the annotation method chosen. It is important to select the optimal annotation method to achieve the desired task with AI.
Click here for a blog about the types of annotations
>What is Bounding Box Annotation
>What is Keypoint Annotation
>What is Segmentation
3. Annotation Tools
While it is possible to use image editing software such as Photoshop for image annotation, in many cases, dedicated annotation tools are used.
Let's consider the bounding box as an example. The annotation data required includes the width and height of the rectangle that surrounds the object in the image, its coordinates, and the class name of that rectangle (for example, car, person, etc.). This information is not written directly onto the image but is instead recorded in a separate file created as annotation data. There are various formats for such annotation data, including COCO-compliant JSON format and others. Most general image editing software does not support these data formats. Therefore, specialized tools are necessary. Some tools may not support the desired output format, but by creating a converter to change the data format, the necessary data can be obtained.
Additionally, since annotations are performed on a large number of images, it is important to have features that allow for efficient work to enhance productivity. For example, features such as a rich set of shortcuts, the ability to smoothly transition between images, and the ability to check and make corrections, as well as management features that allow for tracking the assignment and progress of workers. Most image editing software does not have these features. Of course, there are various annotation tools available, ranging from free open-source options to paid ones, and some may not be sufficient in terms of functionality. However, we recommend considering the ease of work from the perspective of productivity and quality when selecting an annotation tool.
Here is the blog about annotation tools
>What is the Annotation Tool Annofab?
>Comparison of 5 Recommended Annotation Tools - What are the 3 Points to Choose a Tool?
>Comparison of 6 Recommended Text Annotation Tools - What are the 3 Points to Choose a Tool?
4. Tips for Annotation Methods
To proceed with the annotation work, we first gather data to train the AI. If we have data within our company, we can utilize that, but if such data is not available, we can either collect new data or use publicly available datasets for AI research and development.
For the blog about the dataset, click here
>Benefits and Drawbacks of Using Annotated Open Datasets
Once the data is ready, we will begin the annotation work. First, we will create work instructions and specifications to ensure that the workers perform their tasks correctly. We will clearly state the annotation criteria and use not only text but also reference images to prevent any discrepancies in the workers' understanding. Additionally, there will inevitably be edge cases that may cause confusion in judgment, so it is advisable to include such examples as well.
Once the instruction manual is ready and the setup for tools and data is complete, we will conduct a lecture for the workers and finally begin the annotation work. After starting, various management tasks will be necessary, including addressing questions that arise during daily work, managing progress and quality, and responding to unexpected troubles. Sometimes, it may be necessary to align quality among workers in meetings, and there may also be interactions with the AI development personnel regarding questions and other matters. Relying entirely on the workers for these tasks will increase their burden beyond the annotation work. By having the PM follow up, the annotation project can proceed smoothly.
There are tips that can be gained by doing a lot of annotations regarding this method of annotation. If you are trying annotation for the first time or if it doesn't go well even when you try it in-house, it is recommended to refer to these tips.
Here is a blog about tips for annotation
>Essential knowledge and tips for annotation work
>7 tips to lead annotation to success
>How to ensure and improve the quality of training data? Practical methods explained!
5. Summary
So far, we have explained the method of annotation by introducing the blogs that our company has published. In addition to the blogs introduced here, we have released many explanatory articles on annotation and AI. Furthermore, as a PM who understands the field while actually progressing with annotation work, we regularly share insights on the difficulties of annotation, candid opinions, and exclusive stories that are not often made public as spin-off blogs. If you are interested, we would be grateful if you could read our other blogs as well. And when considering outsourcing annotation, please feel free to consult with our company.
For a list of our company blogs, click here
>Annotation Services Blog
6. Human Science Annotation, LLM RAG Data Structuring Agency Service
A rich track record of creating 48 million pieces of training data
At Human Science, we are involved in AI model development projects across various industries, starting with natural language processing, including medical support, automotive, IT, manufacturing, and construction. Through direct transactions with many companies, including GAFAM, we have provided over 48 million high-quality training data. We accommodate various types of annotation, data labeling, and data structuring, from small-scale projects to long-term large projects with a team of 150 annotators, regardless of the industry.
Resource management without using crowdsourcing
At Human Science, we do not use crowdsourcing; instead, we advance projects with personnel directly contracted by our company. We form teams that can deliver maximum performance based on a solid understanding of each member's practical experience and their evaluations from previous projects.
Supports not only annotation but also the creation and structuring of generative AI LLM datasets
In addition to labeling and annotation for identification systems for data organization, we also support the structuring of document data for the construction of generative AI and LLM RAG. Since our founding, we have been engaged in manual production as a primary business and service, leveraging our unique know-how gained from a deep understanding of various document structures to provide optimal solutions.
Equipped with a security room in-house
At Human Science, we have a security room that meets ISMS standards within our Shinjuku office. Therefore, we can ensure security even for projects that handle highly confidential data. We consider the protection of confidentiality to be extremely important for all projects. Even for remote projects, our information security management system has received high praise from our clients, as we not only implement hardware measures but also continuously provide security training to our personnel.