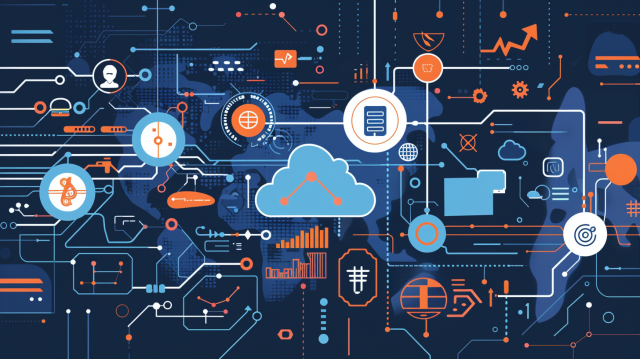
LLMs (Large Language Models) like the widely recognizable ChatGPT have significantly improved in performance since their inital release. They can perform various tasks, such as text and program code generation, information analysis, and information gathering, with the help of prompts (instructions to AI) that are given to the system in plain language (natural language). In contrast to "traditional" AI models, which had to be developed from scratch for specific tasks, the barriers to utilizing LLMs have been greatly lowered, leading to their application in various fields, including business, research and development, and entertainment. For the business sector in particular, improving operational efficiency through digital transformation (DX) has become an essential task, and efforts to utilize LLMs are becoming more active.
Many LLMs are being developed under the leadership of American companies such as Meta and Microsoft. These models are based on training in English, and are then adapted for other languages through additional training. For general use in Japanese, their performance can be considered sufficient, though the accuracy is a bit questionable when it comes to more specialized fields. This is also true when applied to business use. If there were LLMs specialized in Japanese that reflect Japan's unique culture and business practices, this would help promote digital transformation in domestic companies. As a result, an increasing number of companies and research institutions have begun developing LLMs specialized in Japanese. Here, we would like to introduce you to a few.
- Table of Contents
1. Why Use a Japanese-Specific LLM?
The capabilities of LLMs are are largely thanks to having trained on the vast amount of data available on the internet. As of January 2024, content in English accounts for over 50% of all web content, while Spanish holds a distant second place at 6%. Japanese makes up approximately 4% of web content (Statista: Languages most frequently used for web content as of January 2024, by share of websites; February 2024 article). As the name suggests, LLMs enhance their performance by learning from massive datasets. Increasing the data volume allows them to learn more broadly, thereby improving the versatility, generative ability, and accuracy. Currently, many of the companies that develop LLMs are based in English-speaking regions, so it stands to reason that the language LLMs excel in the most is English.
Of course, many LLMs do support Japanese. ChatGPT is but one example, with excellent Japanese capabilities. However, because the amount of data available on the internet in Japanese is significantly less than that in English, depending on the content of your questions, these general LLMs may not be able to generate accurate responses. To make matters even more difficult, writing in Japanese involves the complex interplay of multiple scripts (kanji, hiragana, and katakana), and the boundaries between words are not always clear. Other unique challenges include the need to understand sentences in the context of the lines that come before and after, which is less of a requirement in English, where every sentence includes its own subject and verb. Furthermore, the ability to generate responses that naturally capture the nuance of honorifics and other Japanese-specific expressions is an area in which many existing LLMs could stand to improve. Next, let's look at some LLMs that are currently being developed with a focus on Japanese.
2. Top 3 Recommended Japanese-Specific LLMs
● CyberAgentLM3
This is a Japanese-specific LLM developed by CyberAgent, Inc. As of July 2024, this model has 22.5 billion parameters, and according to the Japanese language ability evaluation metric "Nejumi LLM Leaderboard 3," it has recorded performance equivalent to Meta's LLM, "Meta-Llama-3-70B-Instruct." It is one of the top-class models for Japanese LLMs.
Reference URL: CyberAgentLM3 Demo
● ao-Karasu
Lightblue Technology is a startup that offers services to add AI assistant features to chat tools like Slack. It released its Japanese LLM "Qarasu" in December 2023, promising top-class performance within Japan. After the release of this model, further development has continued, with the ao-Karasu model announced in March 2024, just four months later. This LLM contains 72 billion parameters, boasting performance that surpasses GPT-3.5.
Reference URL: ao-Karasu: Cutting-edge 72B Japanese LLM Development
● ELYZA
This Japanese language-focused LLM is operated by ELYZA, a startup that was born from the Matsuo Laboratory at the University of Tokyo. Based on Meta's "Llama 3," this model has been trained using a unique Japanese dataset, and the commercially available "Llama-3-ELYZA-JP-8B" boasts 8 billion parameters, remaining lightweight. Its performance has been deemed comparable to "GPT-3.5 Turbo" and "Gemini 1.0 Pro" in automatic evaluations using two benchmarks for measuring Japanese performance (ELYZA Tasks 100 and Japanese MT-Bench).
As of June 2024, a model with 70 billion parameters is also in development, which claims to surpass GPT-4 in terms of Japanese performance. You can catch a glimpse of its capabilities in the demo version.
Reference URL: ELYZA LLM for JP, Demo Version
3. Summary
Above, we have introduced just three of the Japanese language-specific LLMs being developed by companies and research institutions, of which there are many more. Among these LLMs, there are models that rival the performance of ChatGPT, and it is believed that the utilization of these LLMs will flourish in professional business. If we can leverage Japanese language-specific LLMs, perhaps we can accelerate the promotion of DX through means like using internal data in conjunction with RAG (Retrieval-Augmented Generation) to improve operational efficiency and knowledge management. Though on the other hand, internal office data often consists of unstructured information, such as emails, documents containing charts and images, meeting minutes, and business reports, which are difficult to use as training data in their original form. When implementing LLMs, certain preparations, such as structuring this data, are necessary. And since it may be difficult to carry out such tasks internally, we recommend that you consider using an external vendor who specializes in data structuring as you proceed with the implementation.
4. Human Science Annotation, LLM RAG Data Outsourcing Service
Over 48 million pieces of training data created
At Human Science, we are involved in AI model development projects across various industries, starting with natural language processing and extending to medical support, automotive, IT, manufacturing, and construction, just to name a few. Through direct business with many companies, including GAFAM, we have provided over 48 million pieces of high-quality training data. No matter the industry, our team of 150 annotators is prepared to accommodate various types of annotation, data labeling, and data structuring, from small-scale projects to big long-term projects.
Resource management without crowdsourcing
At Human Science, we do not use crowdsourcing. Instead, projects are handled by personnel who are contracted with us directly. Based on a solid understanding of each member's practical experience and their evaluations from previous projects, we form teams that can deliver maximum performance.
Support for not just annotation, but the creation and structuring of generative AI LLM datasets
In addition to labeling for data organization and annotation for identification-based AI systems, Human Science also supports the structuring of document data for generative AI and LLM RAG construction. Since our founding, our primary business has been in manual production, and we can leverage our deep knowledge of various document structures to provide you with optimal solutions.
Secure room available on-site
Within our Shinjuku office at Human Science, we have secure rooms that meet ISMS standards. Therefore, we can guarantee security, even for projects that include highly confidential data. We consider the preservation of confidentiality to be extremely important for all projects. When working remotely as well, our information security management system has received high praise from clients, because not only do we implement hardware measures, we continuously provide security training to our personnel.