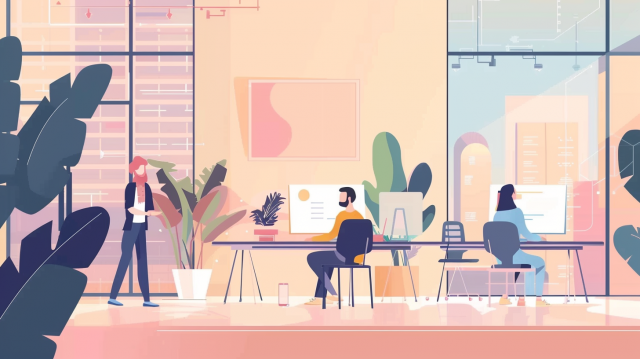
LLMs (Large Language Models) like ChatGPT and Gemini excel at performing a wide range of tasks such as document generation, programming, and information retrieval with high accuracy in response to user prompts that resemble natural speech. However, it is important to note that the generated content is not always guaranteed to be correct. The data that LLMs learn from consists of text data available on the internet, but they do not always learn the most up-to-date information. Additionally, they cannot learn information that is not available online. Due to their nature, LLMs can produce responses that sound plausible even when they are based on incorrect information, a phenomenon known as "hallucination."
In order to promote DX in business, we want to utilize LLMs to improve efficiency and productivity in various operations. However, due to the challenges mentioned above, it is difficult to use existing LLMs as they are. That said, developing an LLM from scratch incurs enormous development costs, making it unrealistic. Therefore, various technologies are being developed to customize existing LLMs and enhance their accuracy. Among these, RAG and fine-tuning are attracting attention.
This time, we will explain the differences between RAG and fine-tuning for customizing LLMs.
- Table of Contents
1. What is RAG?
As we have explained in our company blog, RAG stands for Retrieval-Augmented Generation. The mechanism of RAG can be simply described as the LLM searching for information from untrained data (external data) based on the user's input prompt, adding the search results to the prompt, and passing it to the LLM. The LLM generates responses based on this information. A key feature of RAG is that there is no need for additional training of the LLM itself.
Reference Blog
>LLM and RAG: An Explanation of the Utilization of Generative AI in Business
●Benefits
・It is possible to generate responses based on the latest information
RAG searches the information from a dedicated database that stores external data. If the information in this database is updated with the latest information, the LLM's responses can always be generated to cover the most current information.
●Disadvantages
・The accuracy of the response results depends on external data
If there are errors in the database information, that information will be passed to the LLM, resulting in incorrect answers.
・Data organization is necessary
To perform accurate searches with RAG, attention must be paid to the state of the data stored in the database. For example, parts of PDF documents that contain embedded images cannot be searched with RAG. It is important to use text-based Word files before they are converted to images, if available. Additionally, it is essential to structure documents as much as possible and to ensure that the latest version is used when there are multiple versions of a document, as proper data organization is crucial for accurate searches with RAG.
2. What is Fine-Tuning?
Fine-tuning is a method of customizing existing LLMs by providing additional training datasets tailored to specific purposes. Fine-tuning itself has been used in deep learning, which mimics neural networks, even before the advent of LLMs. The mechanism involves adding a new layer to the output layer of the neural network and adjusting the parameters across the entire LLM through training with the dataset.
The format of the training dataset varies by LLM. For example, for ChatGPT, the dataset is described in a format such as JSONL with <prompt (question), completion (answer)>.
●Benefits
・Improvement of accuracy for specific domains and tasks
Fine-tuning allows for specialized learning in specific domains due to the way LLMs learn. For example, by focusing on learning specific to the medical field, it becomes possible to operate LLMs with higher accuracy.
●Disadvantages
・Creation of large datasets is necessary
Datasets need to be in a format that can be learned by LLMs. Therefore, it is necessary to create datasets for this purpose, and while the amount cannot be generalized, in some cases, it may require preparing thousands or tens of thousands of data points manually, which takes a tremendous amount of labor.
3. The Difference Between RAG and Fine-Tuning
Both methods share the commonality of customizing the LLM using external data. The major difference is whether the LLM itself undergoes additional training. In RAG, external data is searched and passed to the existing LLM, so there is no need for additional training. On the other hand, in fine-tuning, the LLM undergoes additional training with external data, resulting in the LLM itself being customized.
The characteristics mentioned so far are summarized below.
RAG | Fine-tuning | |
---|---|---|
LLM Learning | None | Available |
Training Data | ・Documents stored in the database, etc. | ・Formats that LLM can process |
Response Generation Method | ・Search the database and generate answers using existing LLM | ・The LLM itself generates responses through additional training |
Benefits | - Easy updates for the latest information | ・High accuracy of responses for specific domains and tasks |
Disadvantages | ・If incorrect information is included, accuracy will not be achieved ・Data maintenance is necessary |
- Creation of training datasets is necessary |
There are such differences between RAG and fine-tuning. It cannot be said outright which method is superior. While RAG is suitable for handling the latest information, fine-tuning is more appropriate if you seek deeper accuracy in specific fields of expertise. Therefore, which method is suitable depends on the purpose, so it is important to carefully consider and choose the customization method.
4. Summary
We have explained RAG and fine-tuning for customizing LLMs. Regardless of which method you choose, it is essential to properly prepare and create external data for customization in terms of both quantity and quality.
As mentioned earlier, at RAG, it is necessary to select documents from the large volume of internal documents that can be reliably searched by RAG, and to perform tasks such as structuring them if necessary. On the other hand, fine-tuning requires creating a large dataset based on external data that we want to use for additional training.
While there is progress in automating these data preparation and creation processes to improve efficiency, human manual work will still be necessary at some point in the data creation process. If you attempt to carry out these tasks in-house, it will lead to additional work outside of your core business, resulting in extra costs. In such cases, it is also a good option to entrust these tasks to a specialized external vendor.
5. Human Science Annotation, LLM RAG Data Structuring Agency Service
A rich track record of creating 48 million pieces of training data
At Human Science, we are involved in AI model development projects across various industries, starting with natural language processing, including medical support, automotive, IT, manufacturing, and construction. Through direct transactions with many companies, including GAFAM, we have provided over 48 million high-quality training data. We accommodate various types of annotation, data labeling, and data structuring, from small-scale projects to long-term large projects with a team of 150 annotators, regardless of the industry.
Resource management without using crowdsourcing
At Human Science, we do not use crowdsourcing; instead, we advance projects with personnel directly contracted by our company. We form teams that can deliver maximum performance based on a solid understanding of each member's practical experience and their evaluations from previous projects.
Supports not only annotation but also the creation and structuring of generative AI LLM datasets
In addition to labeling and annotation for identification systems for data organization, we also support the structuring of document data for the construction of generative AI and LLM RAG. Since our founding, we have been engaged in manual production as a primary business and service, leveraging our unique know-how gained from a deep understanding of various document structures to provide optimal solutions.
Equipped with a security room in-house
At Human Science, we have a security room that meets ISMS standards within our Shinjuku office. Therefore, we can ensure security even for projects that handle highly confidential data. We consider the protection of confidentiality to be extremely important for all projects. Even for remote projects, our information security management system has received high praise from our clients, as we not only implement hardware measures but also continuously provide security training to our personnel.