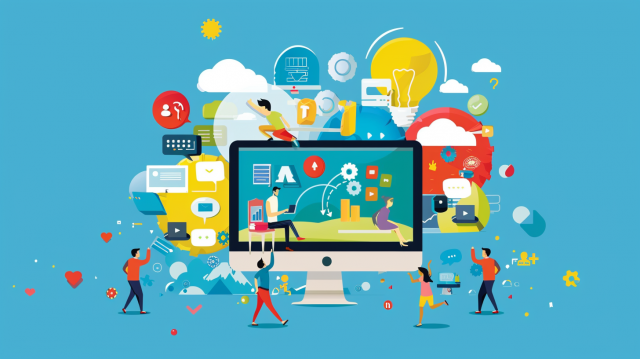
Since the emergence of LLMs (Large Language Models) represented by ChatGPT, many people have been able to easily interact with LLMs, amazed by their ability to perform various tasks such as complex programming, document creation, information retrieval, and analysis, as if they were truly conversing with a human. The movement to leverage this capability for business and promote digital transformation (DX) is also accelerating. By utilizing LLMs, dramatic efficiency improvements are expected in all aspects of business, including content creation, marketing, market analysis, document search, and knowledge management. However, there are challenges where the expected results are not achieved even after implementing LLMs. In this article, we will explain the challenges associated with the utilization of LLMs and discuss ways to address them.
- Table of Contents
1. Challenges in Utilizing LLMs
The overwhelming advantage of LLMs over traditional language models is their ability to understand and output information in a conversational manner, with a very high level of accuracy, comparable to or even exceeding that of humans. Some models possess intelligence that meets the passing standards of the national medical examination. The capabilities of LLMs have been achieved through the enhancement of the three key factors that determine AI accuracy: "computational power," "data volume," and "number of parameters." While LLMs may seem all-powerful, they also have their challenges.
The data that LLM learns from is based on a vast amount of text data collected from the internet. However, it does not continuously learn from the enormous amount of text that is generated in real-time; rather, it has learned from data up to a certain point (for example, for the free version of ChatGPT 3.5, this is up to September 2021). Additionally, while LLM excels at generating general responses across a wide range of fields, it cannot learn about specialized areas or internal company data that is not available on the internet (and even if it could, the amount of data would not be sufficient), which means it cannot provide accurate answers. In some cases, it may produce incorrect information that appears to be genuine, a phenomenon known as "hallucination."
For example, let's consider utilizing LLMs in the manufacturing industry. There is a vast amount of documentation accumulated daily in various operations such as design and development, production management, quality control, and maintenance and inspection for each company. On the other hand, many of the company's unique documents are not publicly available on the internet, so LLMs cannot learn from them. Therefore, using publicly available LLMs as they are may result in not getting answers or experiencing hallucinations, and you may not achieve the desired results.
Various methods have been researched and devised to address the challenges when trying to utilize LLMs for specific purposes. Among them, fine-tuning and RAG are widely known. Therefore, this time we will focus on fine-tuning and explain it.
Reference Blog
>LLM and RAG: An Explanation of the Utilization of Generative AI in Business
2. What is Fine-Tuning?
Fine-tuning is a method of providing additional training data tailored to a specific purpose to a pre-trained LLM model and further training it. Fine-tuning itself is a technique used in deep learning with neural networks even before LLMs, where a new layer is added to the end layer of the network to train on a new dataset. The advantage of fine-tuning is that it can be performed with a very small dataset (though still in the thousands to tens of thousands) compared to pre-training, as it involves additional training on a pre-trained LLM.
LLMs provided by companies like OpenAI offer APIs for fine-tuning, allowing users to perform fine-tuning using these resources. The basic process involves preparing a dataset, adding data for the LLM to learn from, and evaluating the results. The format of the data to be prepared varies by LLM provider, but generally, it consists of data structured as <input, output>. For example, OpenAI's training data format is in JSON, consisting of prompts (questions) and completions (answers).
Example
{"prompt":"What are the features of model HS024?\n##\n", "completion":"As a new feature, it is equipped with a LiDAR sensor that can perceive three-dimensional space.\n###\n"}
The amount of training data varies depending on the complexity and difficulty of the objective, but at least several thousand to tens of thousands of data points are required. While it is possible to tune with a smaller amount, there is a risk of forgetting the pre-trained parts or overfitting, so it is important to prepare a sufficient amount of data when performing fine-tuning.
Additionally, the quality of the dataset is also important. Naturally, the data must not contain incorrect information. It is also necessary to comprehensively cover all the information that you want to train on. For example, in order to enable searching for your company's product information, it is essential to prepare comprehensive information related to the product, such as its specifications, features, design, and release date.
Creating such data often has to be done manually, and considering the amount and quality of the necessary data, it is easy to imagine that it will require a tremendous amount of labor. When conducting fine-tuning in-house, additional work to create training data arises on top of regular operations, which may make it difficult to allocate resources for that purpose.
3. Summary
LLMs possess a versatility that is unmatched by previous AIs. However, that does not mean they can be expected to be utilized effectively in specialized fields or for the latest information as is.
To utilize LLMs in business, it is necessary to customize them according to specific purposes, and one of the methods we have highlighted this time is fine-tuning. As we have seen here, there is a process that requires human effort in collecting and creating the training datasets for fine-tuning. Often, the work of creating training data does not require specialized knowledge, such as that expected from IT engineers. In such cases, outsourcing to vendors specializing in training data creation can be a good option.
Our company has extensive experience and achievements in annotation work for creating "training data," starting with natural language processing annotation, even before the advent of LLMs. We hope to leverage this experience and achievements to assist our clients in their digital transformation by creating training data for fine-tuning LLMs.
4. Human Science Annotation, LLM RAG Data Structuring Agency Service
A rich track record of creating 48 million pieces of training data
At Human Science, we are involved in AI model development projects across various industries, starting with natural language processing, including medical support, automotive, IT, manufacturing, and construction. Through direct transactions with many companies, including GAFAM, we have provided over 48 million high-quality training data. We accommodate various types of annotation, data labeling, and data structuring, from small-scale projects to long-term large projects with a team of 150 annotators, regardless of the industry.
Resource management without using crowdsourcing
At Human Science, we do not use crowdsourcing; instead, we advance projects with personnel directly contracted by our company. We form teams that can deliver maximum performance based on a solid understanding of each member's practical experience and their evaluations from previous projects.
Supports not only annotation but also the creation and structuring of generative AI LLM datasets
In addition to labeling and annotation for identification systems for data organization, we also support the structuring of document data for the construction of generative AI and LLM RAG. Since our founding, we have been engaged in manual production as a primary business and service, leveraging our unique know-how gained from a deep understanding of various document structures to provide optimal solutions.
Equipped with a security room in-house
At Human Science, we have a security room that meets ISMS standards within our Shinjuku office. Therefore, we can ensure security even for projects that handle highly confidential data. We consider the protection of confidentiality to be extremely important for all projects. Even for remote projects, our information security management system has received high praise from our clients, as we not only implement hardware measures but also continuously provide security training to our personnel.