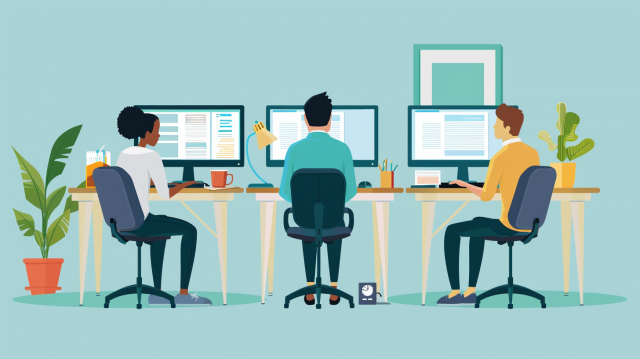
With the emergence of ChatGPT, the trend of AI, which has been primarily focused on "discriminative systems," is making a significant shift towards "generative systems" represented by LLMs. In this article, we will explain LLMs and also discuss RAG, a technology considered challenging to utilize internal data with just LLMs.
- Table of Contents
1. What is LLM?
LLMs (Large Language Models) are AI models built by learning vast amounts of text data through deep learning techniques. Compared to traditional language models, LLMs significantly enhance the three elements of "computational power," "data volume," and "number of parameters." For example, while traditional models have parameter counts ranging from millions to billions, LLMs possess an astonishing number ranging from tens of billions to trillions. High-performance GPUs have enabled the fast processing of these elements, allowing for advanced language understanding and text generation across a wide range of fields.
A representative example of such LLMs is ChatGPT. It has garnered significant attention across various industries since its inception as a model that can respond as if in conversation and generate programming code simply by having users input questions or prompts in spoken language (natural language).
In addition to ChatGPT, various LLMs such as Google LaMDA and GEMINI have been announced and continue to evolve, increasing the potential for their use in business across a wide range of applications.
2. Challenges of LLM
That said, LLMs also have challenges. This is referred to as "hallucination." This phenomenon refers to the generation of text by LLMs that includes incorrect information not based on facts or reality, or completely fictional content. LLM models output words based on the probability of occurrence of text and words. They do not generate text considering whether the information is accurate. They can also generate text about things they have not been trained on.
Causes of 'hallucination' include the LLM not learning the latest information and being unable to access closed data (such as internal data that is isolated from external networks).
To address these challenges, there are techniques such as fine-tuning and additional training of LLMs to enhance their accuracy in specific fields. While these techniques can improve accuracy, they also have the drawback of requiring vast amounts of data and computational resources. A technology that is gaining attention to overcome these drawbacks is RAG.
3. What is RAG?
RAG (Retrieval-Augmented Generation) is a combination of search technology and LLM. While fine-tuning and additional training improve the accuracy of the LLM itself, RAG performs data retrieval and extraction, allowing the LLM to output high-precision answers based on that content. RAG can be operated at a lower cost compared to fine-tuning and additional training, and it also enables access to the latest information and closed data, which was difficult for LLMs. By combining the language understanding capabilities of LLMs with the data retrieval capabilities of RAG for specific areas such as internal information, various business efficiencies can be achieved. The scope of this is extensive. Below are examples of such efficiencies.
Knowledge Management
In knowledge management, it is important to effectively collect, organize, and share knowledge within the organization. With RAG, it is possible to quickly search for relevant information from a large number of documents and databases, allowing for the extraction of necessary knowledge. This enables quick access to the required information, significantly improving operational efficiency. For example, in the manufacturing industry, there may be a vast accumulation of documents such as design standards, processing flow diagrams, business flowcharts, and quality control standards. By utilizing RAG, it becomes possible to quickly search for necessary information within these documents.
Content Generation
It becomes possible to generate content that communicates the company's unique case studies and strengths on websites and blogs. By extracting relevant information through RAG, more accurate information dissemination can be carried out efficiently in a shorter time. For example, when wanting to externally communicate past success stories, utilizing RAG to efficiently collect and integrate various technical reports and staff reports about those cases allows for the generation of content that better highlights the company's strengths.
Customer Support
For manufacturers that release multiple products with manuals spanning hundreds of pages, providing prompt and accurate support in response to customer inquiries is one of the most important tasks. If it takes time to search for the relevant product manual and provide an accurate answer, it can lead to significant losses in customer satisfaction. By utilizing RAG, it becomes possible to analyze past inquiry data and generate optimal responses, which can be expected to improve customer satisfaction. Additionally, it can significantly reduce the burden on the support team and achieve operational efficiency.
4. Challenges of RAG
RAG can search through limited data such as internal data and output results. Conversely, if this data is incorrect, it will produce an incorrect answer, and if the data does not exist, it will not be able to output an answer. Additionally, unstructured data such as scanned blueprints, PDFs, and PowerPoint materials need to be organized to make it easier for RAG to search. For example, for PDFs, methods include applying OCR processing or using Word files that can recognize document layout structures, such as paragraphs before converting to PDF (or creating a Word version).
Therefore, when implementing RAG, it is necessary to maintain and cleanse the data. Additionally, when handling confidential information, security measures such as access restrictions are also essential.
5. Summary
By utilizing LLM and RAG, it is expected to improve efficiency and productivity in various operations. Additionally, it can also be used to support the creation of new value. However, each technology has challenges in terms of accuracy and other aspects. To address these challenges, fine-tuning of LLM or data preparation for improving the accuracy of RAG is necessary. Nevertheless, the task of structuring and organizing the vast amount of documents scattered within the company requires significant labor. Therefore, if it is difficult to allocate internal resources for this, hiring an external vendor is one option.
6. Human Science Annotation, LLM RAG Data Structuring Agency Service
Over 48 million pieces of training data created
At Human Science, we are involved in AI model development projects across various industries, starting with natural language processing and extending to medical support, automotive, IT, manufacturing, and construction, just to name a few. Through direct business with many companies, including GAFAM, we have provided over 48 million pieces of high-quality training data. No matter the industry, our team of 150 annotators is prepared to accommodate various types of annotation, data labeling, and data structuring, from small-scale projects to big long-term projects.
Resource management without crowdsourcing
At Human Science, we do not use crowdsourcing. Instead, projects are handled by personnel who are contracted with us directly. Based on a solid understanding of each member's practical experience and their evaluations from previous projects, we form teams that can deliver maximum performance.
Support for not just annotation, but the creation and structuring of generative AI LLM datasets
In addition to labeling for data organization and annotation for identification-based AI systems, Human Science also supports the structuring of document data for generative AI and LLM RAG construction. Since our founding, our primary business has been in manual production, and we can leverage our deep knowledge of various document structures to provide you with optimal solutions.
Secure room available on-site
Within our Shinjuku office at Human Science, we have secure rooms that meet ISMS standards. Therefore, we can guarantee security, even for projects that include highly confidential data. We consider the preservation of confidentiality to be extremely important for all projects. When working remotely as well, our information security management system has received high praise from clients, because not only do we implement hardware measures, we continuously provide security training to our personnel.