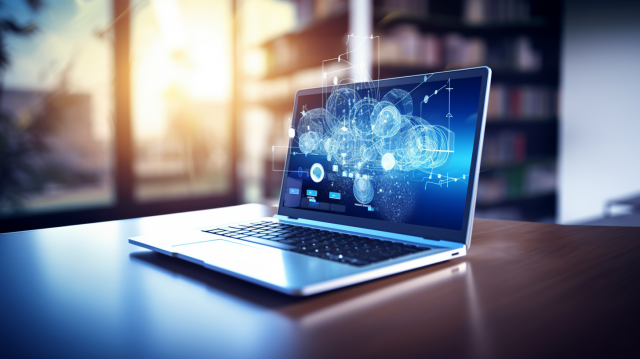
Spinoff Blog Project
――Annotations supporting AI in the DX era. The reality of that analog field
How to deal with edge cases that cannot be fully covered by specifications
~Overcoming edge cases that cause confusion in annotations~
Until now, our company has been publishing various blogs related to annotation and AI. In those, we have focused on conveying general knowledge and know-how. While the task of annotation may seem simple when expressed in words, it is a task that inevitably involves a lot of human interaction due to its inherent "ambiguity" and the fact that it is a "task that cannot be avoided by humans." Therefore, it often becomes quite messy and cannot be resolved with the neat theories that are commonly found. In order to ensure quality and productivity, a variety of experiences and know-how are actually required.
Therefore, understanding the specific problems that occur in the actual annotation field and how to address them can serve as helpful hints for leading to successful annotation.
What actually happens in our field and what specific responses and measures we take. Unlike a regular blog, we would like to share the realities of our field, including our unique features and commitments, under the spin-off blog project titled: "Annotations Supporting AI in the DX Era. The Reality of the Analog Field."
- Table of Contents
1. Edge cases will always occur
If you have experience with annotations, you have likely encountered what is known as an "edge case." An edge case refers to situations in image annotation where it is unclear whether to include a subject or, if included, how to properly enclose it. Such cases are not clearly defined in specifications or manuals as criteria or exceptions, yet they cannot be ignored.
I encountered this regularly when I was working as an annotator. Even when I asked the annotators I was working with, they would respond, "Hmm... I might do it this way, but I'm not sure." or "I feel like it might not need to be enclosed." The answers were never definitive. Before I knew it, I should have been working at a pace of one image per minute, but often ten minutes had already passed.
As annotation is a task that contains a lot of ambiguity, such edge cases are bound to occur. Even with relatively easy annotations, there can be moments of doubt. If we rely on intuition to annotate these edge cases, it can lead to situations where something that was white yesterday is black today, or where Annotator A marks it as black while Annotator B marks it as white, and Annotator C is indecisive. This can result in AI learning in an indecisive manner, which affects accuracy.
While I won't go so far as to say, "Those who master edge cases master annotations," it is undoubtedly true that one of the most labor-intensive tasks in our daily work is handling edge cases.
2. Create a list of questions
Edge cases like these, where annotators and PMs cannot reach a conclusion when compared to the manual, will be discussed and consulted with the customer each time to determine a policy. Once the policy is decided, it will be communicated to the annotators, but there is something to be careful about at this stage. That is to ensure that information does not get buried.
When annotators work remotely, questions are mostly asked using chat tools. As you know, in chat tools, threads such as questions flow away as time passes and become buried as past information. This leads to situations like, "Huh? I think A-san asked something similar the other day, but where was it?" resulting in wasted time spent searching or scrolling through the screen. Additionally, on-site, it is common to have verbal exchanges like, "Kitada-san! Is this XXX?" "Yes! It is XXX!" without any records being kept. (In this case, it would be good if PM Kitada recorded it...)
To avoid such waste and the scattering of information, we will prepare supplementary materials such as a collection of edge cases, or accumulate and share them in a spreadsheet under names like "Question List" so that similar questions can be easily accessed and quickly referenced, ensuring that questions and answers do not become transient information limited to that moment.
3. Differences from the Tool's Comment Function
Some annotation tools come with a commenting feature. This is a very useful function as it allows you to directly communicate specific issues to the annotator on the tool when sending back for QA checks. However, such comments often have difficulties in terms of information aggregation in most tools. This is because information and know-how become dispersed among individual annotators.
We sometimes use tools with comment features in our company. In such cases, we have experienced that the dispersion of information can lead to differences in understanding among individual annotators. There were instances where feedback given to person A regarding a certain annotation method also applied to other annotators. Since the comment feature only allows feedback to be returned to each individual annotator, it became necessary to comment each time similar edge cases arose for multiple annotators, which was cumbersome.
In this way, the tool's comment feature is limited to interactions with individual annotators, so as it stands, important information cannot be shared or communicated to everyone. Ultimately, it is necessary for the PM or the person in charge of checks to pick up the information and include it in documents or question lists.
In terms of delivering necessary information to everyone without any deficiencies, a question list that can aggregate information may seem like an analog and labor-intensive task, but it can be considered a simple and effective tool for information aggregation and operation.
4. Able to share information with customers
By interacting with customers through the list of questions, we often hear them say, "We gained insights that we did not anticipate at the beginning of development." Such insights can help advance AI development in a better direction. Of course, we can provide this list of questions upon delivery if desired.
5. Summary
In annotation, edge cases that are not covered in the manual inevitably arise, which can be a source of frustration for annotators. This leads to spending time on policy considerations and inquiries, resulting in decreased productivity. To minimize these factors, our PM makes it a point to identify and resolve potential edge case patterns as early as possible in the process (sometimes even before the annotation work begins), utilizing a list of questions. It is also essential to thoroughly check the data provided by our clients. Although this can be a time-consuming task, spending a few hours here can prevent waste that could multiply in terms of both labor and quality once the actual work begins. We hope that the completed annotation data will contribute to the success of our clients' AI development.
Author:
Manabu Kitada
Annotation Group Project Manager
Since the establishment of our Annotation Group, we have been broadly responsible for team building and project management for large-scale projects centered on natural language processing, as well as the formulation of annotation specifications for PoC projects and consulting aimed at scaling.
Currently, in addition to being a project manager for image and video annotation and natural language annotation, I am also engaged in promotional activities such as being a seminar instructor for annotation and writing blogs.
Related Blog Posts
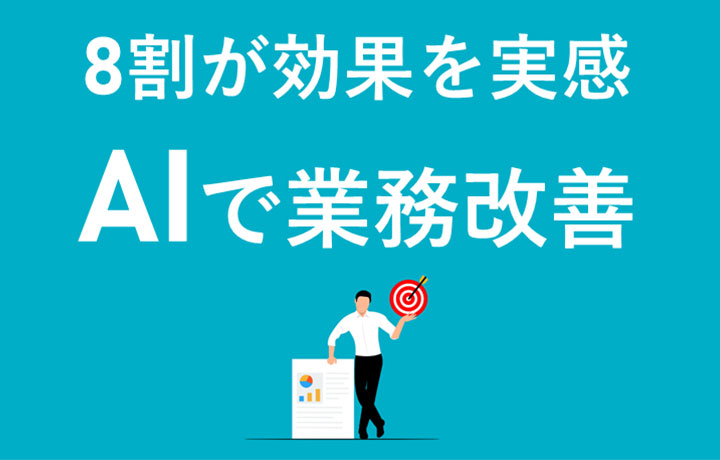
- Most Popular
-
-