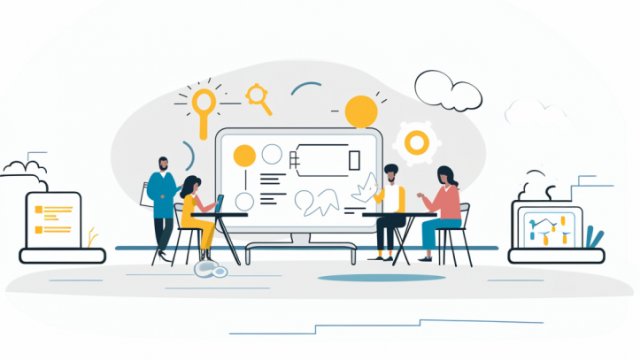
The use of AI is advancing in various fields. With AI, it has become possible to process not only quantitative data but also qualitative data using its learning capabilities, and the areas where AI will be utilized will continue to expand. AI improves recognition accuracy through learning. To achieve this, there are various methods such as "supervised learning," which uses training data known as teacher data, and "unsupervised learning," where AI interprets and learns from data without the need for training data. Here, we will mainly introduce practical methods for creating teacher data within AI development projects, focusing on "supervised learning."
- Table of Contents
-
- 1. What is Teacher Data
- 2. The Importance of Teacher Data Quality
- 2-1. Benefits of High-Quality Training Data
- 2-2. What is High-Quality Training Data?
- 2-3. Disadvantages of Low-Quality Training Data
- 3. Basic Methods for Creating Teacher Data
- 3-1. Setting Objectives
- 3-2. Collecting Data
- 3-3. Perform Annotation
- 4. Specific Practices to Ensure the Quality of Teacher Data
- 4-1. Clarify the Purpose
- 4-2. Ensure the quality and appropriate quantity of collected data
- 4-3. Ensuring the Quality of Annotations
- 4-4. Considerations for Security, Privacy, and Copyright
- 5. Summary
- 6. Human Science Annotation Agency Services
1. What is Teacher Data
In order for AI to recognize the necessary information from data, learning is required. The data used for this learning is called training data. For example, to enable AI to recognize whether a car is present in an image, we prepare images of cars and provide them with the information "This photo contains a car" as training data. The process of adding information to the data is called "annotation."
>> Related Links
2. The Importance of Teacher Data Quality
The quality of training data is a very important factor that determines the accuracy of AI. AI learns based on training data and makes predictions on unknown data. The quality of training data affects the accuracy of AI. To avoid issues where AI makes misrecognitions due to poor quality training data, it is important to ensure quality when creating training data.
2-1. Benefits of High-Quality Training Data
AI trained on high-quality training data can make highly accurate judgments on unknown data. There is no need for AI retraining or modifications to the training data, allowing projects to progress without slowing down the speed of AI development.
2-2. What is High-Quality Training Data?
While it has been stated that high quality has benefits for AI learning, what exactly does high quality entail?
To achieve the objectives in AI development, it is essential to clarify the requirements for the AI to correctly recognize data and define what kind of training data is needed. Based on this definition, it is necessary to prepare work instructions and specifications for annotation and create training data accordingly.
What is important to note here is that the accuracy of annotations is different from high quality. Let's consider the example of an annotation that surrounds a car with a bounding box. When we think of high quality, we might assume that the bounding box must fit perfectly around the car. However, if the specifications do not require such a level of annotation accuracy, it is possible to maintain quality even with some margin. What matters is to work faithfully according to the specifications, rather than blindly pursuing accuracy.
High-quality training data refers to training data that has been correctly annotated based on work instructions and specifications.
2-3. Disadvantages of Low-Quality Training Data
If AI learns from low-quality training data, misrecognition is inevitable. In such cases, not only will there be a need to correct or add to the training data and retrain the model, but there will also be associated management costs. Therefore, when considering outsourcing annotation, it is necessary to evaluate not only the reduction of work costs but also quality and various other aspects.
For example, if a specification states, "Create a bounding box that fits perfectly around the car," but a rough bounding box is created instead, it will include background information other than the car within the bounding box. As a result, the AI learning model may proceed with training that includes noise such as background information. This could lead to misrecognition. Consequently, it may require starting over with the recreation of the training data, incurring costs that were originally unnecessary, and negatively impacting the project with delays in development.
3. Basic Methods for Creating Teacher Data
The creation of training data is divided into three main steps. First, we set the objectives for the AI. Next, we collect the necessary data. Finally, we perform annotation on the collected data.
3-1. Setting Objectives
First, we set the purpose of the AI. For example, developing an AI that can perform image recognition while driving for autonomous driving. Even if we vaguely say that we will perform image recognition, the AI will not learn on its own, so we need to define what kind of objects we want the AI to learn about.
3-2. Collecting Data
We will collect the data necessary for the specified purpose. In the example above, this would include images captured by an onboard camera.
3-3. Perform Annotation
We will create training data by annotating the collected data with bounding boxes and other annotations, so that AI can correctly recognize the data.
*Meaning of Annotation
Annotation originally means "to add notes."
For example, it is like putting sticky notes on a book or adding asterisks to words and writing notes in the margins.
In the creation of training data, it involves specifying certain locations of the data that you want the AI to recognize (such as bounding boxes or segmentation for images, or underlining for text) and linking labels to them (also known as labeling), which allows the AI to learn from the data.
4. Specific Practices to Ensure the Quality of Teacher Data
From here, we will introduce the basic practical methods for creating training data.
4-1. Clarify the Purpose
As mentioned in the section on setting objectives, if the objectives are vague, it will be difficult to train the AI. When creating training data, vague instructions will not allow for proper annotation. As a result, the quality of the training data will be uncertain, and the AI's learning will not proceed effectively. By clarifying the objectives, such issues can be avoided.
4-2. Ensure the quality and appropriate quantity of collected data
In order for AI to learn, a certain amount of training data is necessary. The required amount varies depending on the complexity of the objective, so it cannot be generalized, but in terms of images, it often requires thousands to tens of thousands of images.
: Use our own data
If you have a large amount of data that you have accumulated in-house, you can utilize it. Data that was stored without the premise of AI utilization, such as meeting minutes, call logs, images, and videos, can also create new value through the use of AI. Since there is no need to collect new data, the development period can be shortened. There are tools available that can extract images from videos, so if you can prepare the necessary amount of data, utilizing your own data would be a good choice.
: Use the survey
Collecting data through surveys and emails is also a good method. In the past, it involved tedious street surveys, mailing, and phone calls, which were very time-consuming and costly. However, now by utilizing social media and crowdsourcing, it is relatively low-cost and easy to directly obtain the raw voices of the target audience you want to analyze with AI.
: Utilize the dataset
If you cannot gather such a large amount of data in-house, you may consider utilizing open datasets such as COCO.
: Be cautious of data bias
Let's prepare various types of data. For example, when collecting images from in-vehicle cameras, we should include not only urban images but also various scenes such as highways, mountainous areas, night, and rainy weather. By doing this, we can prevent overfitting of the AI, which would increase accuracy only for specific situational images while decreasing accuracy for others.
: Incorporate Negative Samples
This includes data that does not have any target to be recognized. Such data is called negative samples and is effective in improving the recognition accuracy of AI.
4-3. Ensuring the Quality of Annotations
To ensure the quality of training data, annotations must be performed correctly. To achieve this, it is necessary to determine the specifications for annotations, design standards and rules so that anyone working based on those specifications will produce consistent results, and manage various aspects to create quality training data in accordance with those standards.
: Determine the specifications for annotations
We will determine the specifications for the annotations based on the clearly defined requirements in the objective setting.
: Design work processes and rules
We create work instructions and specifications based on the annotation specifications. When creating them, let's devise the work methods to minimize the chances of errors. It is not guaranteed that annotators understand the work instructions and specifications at the same level, so there may be variations in how labels are applied. It is necessary to design a work process to address potential questions and variations that may arise during the work. For example, incorporating a process not only to check the annotated data but also to provide feedback.
Management for Ensuring Quality
To ensure the quality of annotations, it is important to determine specifications and design processes. However, appropriate management is essential to advance annotations on-site based on these. Always keep an eye on any discrepancies with work instructions and specifications, and in addition to checks and feedback, hold meetings and individual hearings as necessary to ensure quality management.
4-4. Considerations for Security, Privacy, and Copyright
The data handled in annotations may, in some cases, require work in a high-security environment. Additionally, there is a possibility of unknowingly using data that could infringe on privacy or is protected by copyright as part of a dataset. It is important to carefully examine the conditions under which the data can be used in advance.
5. Summary
The accuracy of AI recognition is influenced by the quality of the training data. Even if the objectives are clearly defined and the necessary quantity and types of data are collected, if the training data is of low quality, desirable results cannot be achieved. To avoid this, we have explained practical methods to ensure and improve quality. Among these, the annotation for creating training data requires manual work, making human-centered management essential. While these may seem obvious, let's ensure we do not overlook these fundamentals.
By implementing these practices, the quality of the training data can be ensured, and an improvement in AI accuracy is expected. If the set objectives can be achieved, the project will be successful, allowing for a definite progression to the next steps, such as tackling more challenging goals or product releases.
6. Human Science Annotation Agency Services
Over 48 million pieces of training data created
At Human Science, we participate in AI model development projects across various industries, including natural language processing, medical support, automotive, IT, manufacturing, and construction. To date, we have provided over 48 million high-quality training data through direct transactions with many companies, including GAFAM. We handle a wide range of annotation projects, from small-scale projects to long-term large-scale projects with 150 annotators, regardless of the industry. If your company wants to implement AI models but doesn't know where to start, please feel free to consult with us.
Resource management without crowdsourcing
At Human Science, we do not use crowdsourcing. Instead, projects are handled by personnel who are contracted with us directly. Based on a solid understanding of each member's practical experience and their evaluations from previous projects, we form teams that can deliver maximum performance.
Utilizing the latest data annotation tools
One of the annotation tools introduced by Human Science, AnnoFab, allows customers to receive progress checks and feedback from the cloud even during the project. By ensuring that work data cannot be saved on local machines, we also take security into consideration.
Secure room available on-site
At Human Science, we have a security room that meets ISMS standards within our Shinjuku office. This allows us to handle even highly confidential projects on-site while ensuring security. We consider the protection of confidentiality to be extremely important for all projects. Our staff undergoes continuous security training, and we exercise the utmost caution in handling information and data, even for remote projects.
Related Blog Posts
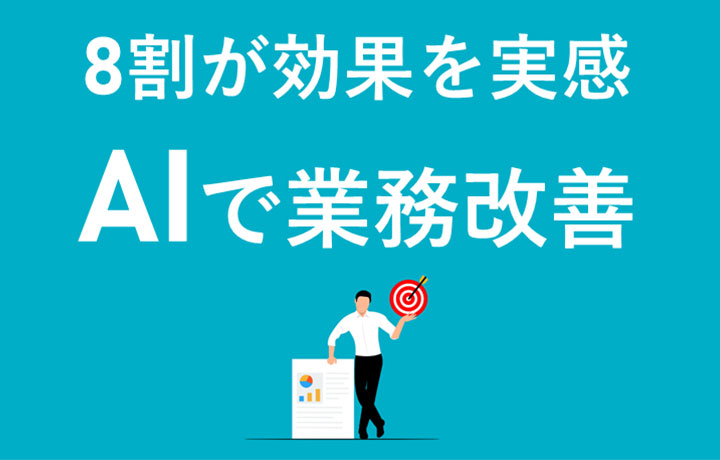