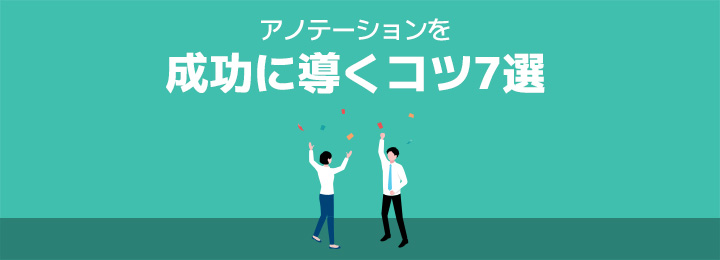
In annotation, we create training data for AI models with various purposes. The data we handle spans a wide range, including images, videos, text, and audio, each requiring different tools and annotation work specifications. Since training data is the foundation for AI model learning, it is essential to produce data that meets specifications and quality requirements. Additionally, in the fast-paced development of AI models, the rapid execution of annotation is crucial. Furthermore, cost cannot be overlooked. Achieving these required elements is the key to successful annotation. Our company is also engaged in daily trial and error, and through this experience, we will discuss and explain seven key tips primarily focused on quality that should be kept in mind.
>>Related Blog
What is Annotation? An Explanation of Its Meaning and Its Relationship with AI Models and Machine Learning
- Table of Contents
-
- 7 Tips for Successfully Leading Annotations
- 1-1. Purpose of Annotations
- 1-2. Collecting Various Types of Data
- 1-3. Create Work Standards and Specifications
- 1-4. Establish efficient procedures for annotation work
- 1-5. Establishing a Check Process
- 1-6. Establish a smooth mutual communication environment
- 1-7. Implement Feedback
- 1-8. Conduct a review
- 2. Points to Note When Making Annotations
- 3. Summary
- 4. Human Science Annotation Agency Services
7 Tips for Successfully Leading Annotations
Annotation primarily involves the task of tagging data. While it may seem like a simple task, in practice, it often proves to be more complex than expected. This is because the annotation work, and by extension the AI model, mimics the functioning of our brains, which we usually perceive intuitively. For example, when annotating the "Tuna Quality Recognition AI Model" that simulates a buyer judging the quality of tuna based on the cut of its tail, it is necessary to translate the tacit knowledge (features based on experience) that buyers intuitively recognize into data. Managing how to capture such intuitive tasks both quantitatively and qualitatively, in order to ensure the quality of the training data, is key to the success of annotation.
1-1. Purpose of Annotations
There are three methods by which AI learns through deep learning based on algorithms modeled after neural networks: "supervised learning," "semi-supervised learning," and "unsupervised learning." Among these, supervised learning requires the creation of training data.
Training data refers to data that has been labeled (tagged) to identify the subjects that we want the AI to recognize. This labeling process is also known as annotation work. In other words, the purpose of annotation can be said to be the creation of training data.
1-2. Collecting Various Types of Data
Just as humans accumulate knowledge through experience and can respond to various challenges, AI models also improve their recognition accuracy by learning from diverse data. To achieve this, we should collect as many different types of data as possible. Taking vehicle detection as an example, it is important to have images not only from busy urban traffic but also from rural areas, where there are fewer vehicles and the background features mountains or winding roads instead of cityscapes. Additionally, we will prepare images of vehicles from various angles, such as front, side, rear, and overhead. By teaching the AI model these various characteristics of vehicles, we can enhance its recognition accuracy.
Even with a small amount of data, data augmentation such as reducing image resolution, flipping images horizontally, or cropping parts of images can help supplement the types of data. Additionally, if the types of data are varied but the amount is small, overfitting can occur in AI models, which may lead to incorrect recognition of newly loaded images. Therefore, it is ideal to prepare as much data as possible. Our company often receives requests for thousands to tens of thousands of files.
1-3. Create Work Standards and Specifications
Human annotation is necessary to create training data for AI models. To ensure that the annotators understand the work requirements and produce accurate data, let's prepare well-organized and easy-to-understand work standards and specifications.
In addition to explaining the annotation rules in writing, let's make it visually clear by using screenshots of the work tools. It would also be good to include flowcharts that cover the process from start to finish. Additionally, if there are any edge cases that may cause confusion in judgment, be sure to document them.
If possible, let's conduct test annotations. This allows us to identify edge cases, improve work standards and specifications, review tool settings, and predict progress. This can help ensure that the actual work proceeds smoothly. However, there may be times when there is not enough time to select and set up the annotation tools. Additionally, it is realistically impossible to identify all edge cases in advance, so it is unavoidable that responses will arise after the annotation work has begun. For handling edge cases, please refer to the section on communication mentioned later.
1-4. Establish efficient procedures for annotation work
When annotating, it is important to consider in advance the steps to take in order to work efficiently. For example, if you are using an annotation tool to assign multiple types of tags, simply setting it up so that frequently occurring tags can be selected quickly can save several seconds of work time. If it is a project where each person creates 10,000 bounding boxes, this alone can reduce the time by 10,000 to 30,000 seconds, which is equivalent to 2.5 to 8 hours.
In projects with many types of tags, switching between tags can take time. Some tools allow you to set keyboard shortcuts for tags. When selecting a tool, it's important to keep these points in mind.
>>Related Blog
Comparison of 5 Recommended Annotation Tools - What are the 3 Points to Consider When Choosing a Tool?
Not only productivity but also quality in image annotation is important, and where to start working on the screen is crucial. The starting position and the method of progress can affect the ease of work and careless mistakes. Additionally, it may impact the checking process mentioned later. Of course, the easiest working methods vary by annotator, so there is no absolute way that must be followed. However, it is an undeniable fact that complicated working methods and tool operations can be significant factors that induce human error, so it is recommended to keep the number of steps and actions minimal and to use simple operations and working methods as much as possible.
In our actual project experience, we often hear comments like, "We continued with the way we were used to because we started that way, but there are actually easier and faster methods out there." Therefore, it is important to listen to the voices of the annotators and share easier methods with the entire team.
1-5. Establishing a Check Process
Needless to say, let's incorporate the check process into the annotations. By doing so, we can ensure the quality of the annotations and lead the project to better results.
Setup of the Check Phase:
By checking the data that has undergone annotation work, you can discover careless mistakes, discrepancies in individual annotators' perceptions, and levels of understanding. By checking and correcting these, you can ensure consistent data and also obtain information that should be fed back into the work. Therefore, postponing the checks will continue to produce errors, so it is recommended to conduct checks from an early stage.
There are methods such as assigning a dedicated reviewer during the check and mutual checks by annotators. The choice between the two depends on the difficulty and scale of the project.
Check Rate:
While it is ideal to conduct a full check, it does incur additional costs. Depending on the accuracy required by the AI model, spot checks may sometimes be sufficient. Additionally, if the difficulty of annotation is low, it is expected that operational errors will also decrease, making low-rate spot checks a reasonable choice. Furthermore, as the project progresses, annotators become more accustomed to the work, so starting with a full check and then switching to spot checks midway can also be an effective approach.
Creation of check procedures and check sheets:
In addition to the work standards and specifications, creating a procedure manual for the checking process is also a good approach. In the checking process, the main tasks involve verifying whether the annotated data meets the required quality and checking for careless mistakes. By clearly stating the key points that should be emphasized during such checks, it helps eliminate unnecessary details that do not impact quality, akin to nitpicking, allowing for more efficient checking work. Furthermore, if there are multiple checkers, sharing the procedure manual and check sheets among them can help reduce variations in check items and perspectives, leading to more stable quality.
1-6. Establish a smooth mutual communication environment
I feel that I am actually doing annotation work, but people often hesitate to ask questions even when they have doubts. The reasons include thoughts like, 'I can't ask such a basic question, it's embarrassing,' 'I want to ask, but I might not be able to explain it well,' 'I would hate to be seen as not fully understanding the work standards or specifications,' and 'I feel sorry for taking up someone's time by asking a question.' People's psychology is quite varied.
Such hesitation itself can already be a factor that lowers the efficiency of the project. Therefore, it is extremely important for the entire team to have the sense that "communication can be done casually." To achieve this, it is very important to implement regular team meetings and encourage the effective use of chat tools. Additionally, an overly formal environment can also create factors of hesitation and reluctance, so it would be ideal to successfully create a positive team atmosphere.
For these reasons, choosing the right annotators is important. Often, when you gather people just because the task seems simple, it does not go well. Suitable candidates for annotation work should not only be able to read and understand the work standards and specifications properly, but also be able to communicate effectively and responsively, possess the necessary PC skills to operate tools, and be capable of consistently performing detailed tasks over long periods. Assembling such personnel can be surprisingly challenging, so it may be a good option to entrust this to an external vendor that has the right talent.
Implementation of the Kick-off Meeting:
Avoid starting by simply handing the work standards and specifications to the annotator and saying, "Please take care of this." Conduct a kickoff meeting to explain the project's objectives as much as possible and demonstrate the actual workflow using tools on screen. This can convey subtle nuances that cannot be fully captured in documents. Additionally, having the team meet in person at the start can serve as a foundation for future communication.
Handling Edge Cases:
In annotation work, there are often cases that were not anticipated in the work standards and specifications beforehand, as well as exceptional cases that are not clearly described in the specifications. In such cases, there can be inconsistencies in judgment among annotators, and edge cases frequently arise where it is unclear how to annotate (or whether to annotate at all). Annotators should not be left to make their own judgments on these edge cases. While it is important to align with the development team, discussions through meetings or chat tools within the team can often lead to answers. Additionally, through such communication, understanding of the annotation work deepens, and team cohesion increases. Establishing a good communication environment brings positive effects to the project.
1-7. Implement Feedback
Let's regularly provide feedback to the annotators. When they continue working without any feedback, people tend to become increasingly anxious. This can lead to a decrease in motivation and poses a risk of deteriorating quality. Instead of only providing feedback on negative factors like annotation mistakes, let's also communicate positive aspects such as reducing errors and improving productivity. Praising is very important.
Whether to provide feedback to individuals or share it with the entire team sometimes requires careful judgment. Especially regarding negative factors, there may be annotators who do not want others to know about them, so it is preferable to provide feedback to individuals. However, there may also be information that the entire team would want to share included in such feedback. When disseminating information, consider measures such as keeping names confidential.
However, in cases where productivity and quality metrics cannot be improved by the team as a whole, it may be necessary to clarify each individual's performance to objectively understand one's own results and share them with the entire team. While this may be a drastic approach, there are times when such measures are necessary. In such cases, it is also important to provide support through follow-ups, such as 1-on-1 meetings.
1-8. Conduct a review
Once the project is completed, let's reflect on the annotation process as well. We will identify various aspects such as whether the quality met the requirements, whether the progress was on schedule, and whether it stayed within the anticipated budget, including both the positive points and the areas that did not go well, as well as any challenges. We should compile and accumulate the know-how gained from the information and experiences. By utilizing the accumulated information and know-how in the next project, we can achieve better project management.
2. Points to Note When Making Annotations
What must be noted in annotation is the balance between the quality of the annotation data and the overall cost of the work. If too much time is spent on each task in an effort to improve annotation quality, the labor required will increase, leading to higher costs. Moreover, this can also affect the overall progress of the AI project. However, if one becomes overly concerned about labor and ends up with sloppy annotation work, it will not ensure quality and may lead to a decrease in AI recognition accuracy. In the annotation work, which involves creating training data, it is important to determine the limits of the quality level that meets the requirements, as well as to find a balance between quality and productivity.
Above all, when developing AI, it is important to clarify in advance the level of AI recognition accuracy and the quality level of annotations you aim for, and to consistently manage the process from data collection to annotation while being mindful of the seven tips mentioned so as not to deviate from the goals you aim to achieve.
3. Summary
Annotation is built on the continuation of diligent work. It often becomes monotonous, yet it is not simply a series of identical tasks. Here, we have introduced some tips to ensure quality amidst these challenges. However, to actually carry out annotation in-house, it is necessary to manage not only the focus on quality but also the work duration that does not hinder the AI model development cycle, as well as appropriate costs. It can be perplexing to know where to start or how to effectively implement these tips. In such cases, one option is to engage an external vendor with extensive annotation experience. By hiring a vendor with expertise in annotation services, you can concentrate more on your own AI model development.
4. Human Science Annotation Agency Services
Over 48 million pieces of training data created
At Human Science, we participate in AI model development projects across various industries, including natural language processing, medical support, automotive, IT, manufacturing, and construction. To date, we have provided over 48 million high-quality training data through direct transactions with many companies, including GAFAM. We handle a wide range of annotation projects, from small-scale projects to long-term large-scale projects with 150 annotators, regardless of the industry. If your company wants to implement AI models but doesn't know where to start, please feel free to consult with us.
Resource management without crowdsourcing
At Human Science, we do not use crowdsourcing. Instead, projects are handled by personnel who are contracted with us directly. Based on a solid understanding of each member's practical experience and their evaluations from previous projects, we form teams that can deliver maximum performance.
Utilizing the latest data annotation tools
One of the annotation tools introduced by Human Science, AnnoFab, allows customers to receive progress checks and feedback from the cloud even during the project. By ensuring that work data cannot be saved on local machines, we also take security into consideration.
Secure room available on-site
At Human Science, we have a security room that meets ISMS standards within our Shinjuku office. This allows us to handle even highly confidential projects on-site while ensuring security. We consider the protection of confidentiality to be extremely important for all projects. Our staff undergoes continuous security training, and we exercise the utmost caution in handling information and data, even for remote projects.