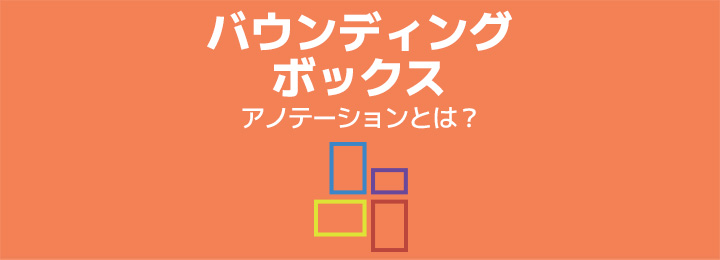
What is Bounding Box Annotation?
Object detection utilizing AI is being applied in various fields such as autonomous driving technology and customer behavior analysis in stores. In order for AI to detect specified objects like people from images, data for training is required in advance. This necessitates tagging information that indicates the objects within the images. The process of creating this information is called annotation. There are many methods of annotation, for example, a method that classifies the image itself by the name (class) of the target to be detected. If the image is like that of a photography studio, where only one object is captured against a simple background, this method may work well for object detection. However, in real images, there are often various objects and backgrounds captured besides the target that needs to be recognized. In such cases, annotation is performed using methods that identify the position and shape of the objects to be recognized within the image. This includes various methods such as bounding boxes that enclose the object in a rectangle and segmentation that colors the object according to its shape. This time, we will explain object detection focusing on bounding boxes, the differentiation of annotation methods, and their usage scenarios.
- Table of Contents
-
- 1. Purpose and Context of Using Bounding Boxes
- 2. What is Object Detection?
- 3. What are the advantages and disadvantages of bounding boxes?
- 4. Differentiation of Annotation Methods Used in Object Detection
- 5. The Necessity of Annotation in Object Detection
- 6. Importance of Data (Annotation Quality and Data Volume)
- 7. Use Cases for Object Detection
- 8. Summary
- 9. Human Science Annotation, LLM RAG Data Structuring Agency Service
1. Purpose and Context of Using Bounding Boxes
Bounding box annotation is one of the methods used for object detection. It allows the information of objects in an image to be represented with simple values such as object attributes, position, and size, making it possible to convert data formats to suit various AI algorithms when creating datasets. Additionally, the task itself is relatively simple as it only involves enclosing the target with a rectangle. Due to the availability of many compatible tools, it is suitable for small-scale projects and object detection tasks that do not require high detection accuracy.
2. What is Object Detection?
By the way, as mentioned in the previous blog "What is Image Recognition? Mechanisms of Image Recognition and Use Cases in AI", object detection refers to the ability of AI to recognize specific objects in images and videos. For example, in the rapidly developing field of autonomous driving, it is necessary to recognize vehicles, pedestrians, signals, and signs ahead using onboard cameras. To enable AI to recognize these elements within images, it is essential to create training data for AI learning, and one of the methods for this is bounding box annotation.
>What is image recognition? Mechanism of image recognition and use cases in AI
3. What are the advantages and disadvantages of bounding boxes?
Benefits
・Cost can be reduced
As mentioned in Chapter 1, creating bounding boxes is a relatively simple task that involves enclosing the target objects in images with rectangles. The time required for one object can be done quickly compared to other annotations mentioned later. Thus, by preparing the necessary training data with less work effort, costs can be reduced, and AI development can proceed with speed.
・Can be used for various object detection
The annotation data with bounding boxes basically contains the following information.
Size of the bounding box (width and height)
Coordinates of the bounding box on the image
Class of the object enclosed by the bounding box (may also include metadata)
AI models for object detection learn from this information. The required data formats vary by model, including JSON formats compliant with YOLO or COCO. Even if the training data created with annotation tools is in a different required format, you can prepare data suitable for the model by using converters to transform the above information into the corresponding format.
Disadvantages
・Not suitable for detecting complex shapes
Bounding boxes do not trace the contours of objects but instead enclose them in rectangles. Therefore, unless the shape of the object being annotated is rectangular, the background behind the object will also be enclosed by the bounding box. This can lead to background noise and contribute to false detections. Additionally, for objects with complex shapes, there is a possibility that the AI model may confuse the contours with the background, resulting in false detections.
To avoid such issues, there are methods such as aligning the bounding box as closely as possible to the object during annotation, and defining and enclosing areas that capture the object's features without including too much background. For example, elongated protrusions like a car's antenna are not considered important elements that capture the characteristics of the car, so they should not be included within the bounding box.
That said, for objects that are almost identical in shape and must be judged by subtle differences in texture (such as facial recognition), or when detection including contours is desired (such as in identifying areas in endoscopic images), bounding box annotation may not be effective. In such cases, it is advisable to adopt other annotation methods, such as keypoint annotation or segmentation, which will be explained in the next chapter.
4. Differentiation of Annotation Methods Used in Object Detection
As explained in the previous section, the advantage of bounding box annotation is its ease of use and high versatility, making it applicable to various object detection tasks. However, there are also disadvantages, so it is important to choose the appropriate annotation method depending on the AI you want to develop. In this chapter, we will introduce annotation methods other than bounding boxes.
Key Point Annotation:
This is a method for specifying specific locations or feature points of an object. For example, joints of the human body and facial landmarks are annotated as key points.
What is Key Point Annotation? Its Features and Annotation Methods
Segmentation Annotation:
This is a method of assigning object areas for each pixel in an image. Each pixel is annotated with a label indicating whether it belongs to the corresponding object class or to the background.
What is segmentation? What can be achieved by utilizing AI segmentation?
In this way, annotation methods can be selected based on requirements such as what kind of object detection you want the AI to perform and the characteristics of the data. Additionally, it is also common to combine multiple annotation methods in the creation of datasets and the training of models.
5. The Necessity of Annotation in Object Detection
There is also a method called "unsupervised learning" that does not use labeled data when advancing AI learning. This method includes techniques such as clustering and principal component analysis. However, "unsupervised learning" in object detection is still in the research stage, and it seems difficult to achieve the same level of accuracy as "supervised learning" at this point. For these reasons, annotation in object detection can still be considered essential in general.
6. Importance of Data (Annotation Quality and Data Volume)
AI learns based on the training data created by annotations. The training data serves as the only clue for object detection. The quality of this data can be equated to the quality of the AI. The quality of the AI is determined by the "quality of the annotations" and the "amount of data."
6-1. Annotation Quality:
If the training data is inaccurate, AI will not be able to achieve high detection accuracy. Annotation work is fundamentally done by hand, so the quality of the data equals the quality of the work done by the person (annotator). To ensure that annotators create correct data, various measures are necessary, including appropriate annotation guidelines, standards, and education and management for the annotators.
When you actually perform annotation work, you realize that there are often troublesome cases (edge cases) that cannot be fully covered by guidelines or standards. In such cases, it is important to establish an environment and system where questions and answers can be easily exchanged, so that work does not proceed while remaining ambiguous. Additionally, since people's perceptions can differ subtly, it is almost impossible for Annotator A and Annotator B to have completely consistent judgment criteria. It is important to accept a certain degree of variation in judgment and manage it to remain within the acceptable range of the required accuracy. Therefore, educating annotators is particularly important.
If you can manage the project to ensure correct annotation, the quality of the training data will inevitably improve, and the detection accuracy of the AI will also increase.
6-2. Data Volume:
The amount of data is also an important factor. No matter how good the quality of the annotated training data is, if the amount of data is small, there will not be enough learning to enable the AI to detect objects. Problems that arise when the amount of data is small include the following:
- 1. Risk of Overfitting:
When the amount of data is small, the model may become overly optimized for the training data and may not generalize well to unseen data. In other words, the AI model may show high performance on the training data but may not make accurate predictions on new data. - 2. Unstable Prediction Results:
When the amount of data is small, the influence of random biases and noise in the dataset used for AI training becomes significant. This can lead to unstable prediction results from the AI model. Even when the same AI model is trained on different datasets, the prediction results may vary. - 3. Limitations of the Model's Generalization Ability:
When the amount of data is small, it becomes difficult for the AI model to appropriately capture and distinguish the diversity and variability of the data. A lack of data diversity can limit the AI model's ability to learn new patterns and features, potentially leading to a decrease in *generalization ability.
*Generalization Ability: Generalization refers to the ability of a learned AI model to generate correct outputs for input data that it has not previously observed.
The amount of data required varies by project, but for example, our company often handles annotations for tens of thousands of image files. Such large-scale annotation projects can take several weeks, and to ensure data volume while maintaining annotation quality, effective management to increase productivity is essential.
7. Use Cases for Object Detection
Here, we will specifically look at the use cases of object detection. In these use cases, bounding box annotations are often used as training data.
7-1. Autonomous Driving:
In autonomous driving technology, object detection is a crucial element. Vehicles need to accurately recognize their surrounding environment and detect obstacles and other vehicles. AI object detection models detect objects in real-time from onboard cameras and sensor data, helping to understand their positions and movements to support appropriate decision-making and evasive actions.
7-2. Video Surveillance:
In a video surveillance system, it is necessary to analyze camera footage in real-time for security and monitoring purposes. By utilizing object detection, suspicious behavior, intruders, and abnormal activities can be detected. For example, by detecting individuals and vehicles and monitoring their positions and movements, it can contribute to enhancing security and the early detection of incidents.
7-3. Image Search:
In image search, AI object detection is used to search for images that contain specific objects or elements. Object detection algorithms analyze large image databases to identify images that contain specific objects or patterns. This allows users to efficiently search for relevant images using keywords or queries.
7-4. Business Analysis:
Commercial analysis utilizes video data from cameras installed in stores and shopping centers to analyze customer behavior and develop effective marketing strategies. AI object detection is used to understand customer movements, behavior patterns, and product popularity. For example, by detecting how much attention people are paying to specific products and which areas are crowded, it can help optimize product displays and store layouts.
8. Summary
As we have seen so far, the application range of object detection is broad, and it will be increasingly utilized in various scenes such as business, research, and healthcare. Furthermore, the necessity for annotations that support object detection is also growing. The annotation process is often time-consuming and requires patience, which can become a hindrance when concentrating resources on research and development. Even if there is a good idea for object detection, it may be difficult for a company to create the necessary annotation data to realize it. In such cases, it can be effective to utilize external vendors that specialize in annotation.
9. Human Science Annotation, LLM RAG Data Structuring Agency Service
Over 48 million pieces of training data created
At Human Science, we are involved in AI model development projects across various industries, starting with natural language processing and extending to medical support, automotive, IT, manufacturing, and construction, just to name a few. Through direct business with many companies, including GAFAM, we have provided over 48 million pieces of high-quality training data. No matter the industry, our team of 150 annotators is prepared to accommodate various types of annotation, data labeling, and data structuring, from small-scale projects to big long-term projects.
Resource management without crowdsourcing
At Human Science, we do not use crowdsourcing. Instead, projects are handled by personnel who are contracted with us directly. Based on a solid understanding of each member's practical experience and their evaluations from previous projects, we form teams that can deliver maximum performance.
Support for not just annotation, but the creation and structuring of generative AI LLM datasets
In addition to labeling for data organization and annotation for identification-based AI systems, Human Science also supports the structuring of document data for generative AI and LLM RAG construction. Since our founding, our primary business has been in manual production, and we can leverage our deep knowledge of various document structures to provide you with optimal solutions.
Secure room available on-site
Within our Shinjuku office at Human Science, we have secure rooms that meet ISMS standards. Therefore, we can guarantee security, even for projects that include highly confidential data. We consider the preservation of confidentiality to be extremely important for all projects. When working remotely as well, our information security management system has received high praise from clients, because not only do we implement hardware measures, we continuously provide security training to our personnel.