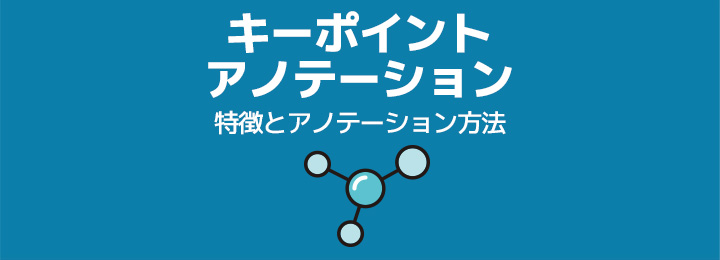
What is Key Point Annotation?
The field of image recognition using AI has evolved significantly in recent years. Along with this, the creation of training data for AI has begun to employ various methods depending on the purpose of image recognition. One of these methods is keypoint annotation. In this method, the characteristic positions (landmarks) of specific objects in images or videos are annotated as points. Since landmarks are annotated, it is sometimes referred to as landmark annotation. While it can be explained separately as keypoint annotation when annotating points such as joints, and as landmark annotation when annotating points on the surface of a face, we will explain it here without distinction as keypoint annotation.
- Table of Contents
-
- 1. Why is key point annotation attracting attention?
- 1-1. Pose Estimation and Motion Capture
- 1-2. Face Recognition
- 2. How to Perform Key Point Annotation
- 2-1. Data Collection
- 2-2. Selection of Annotation Tools
- 2-3. Selection of Points
- 2-4. Implementation of Annotations
- 2-5. Data Verification and Correction
- 3. Summary
- 4. Human Science Annotation Agency Services
- Over 48 million pieces of training data created
- Resource management without crowdsourcing
- Utilizing the latest data annotation tools
- Secure room available on-site
1. Why is key point annotation attracting attention?
Keypoint annotation is mainly used for applications such as posture estimation and facial recognition. In the annotation for posture estimation, points are input by estimating the positions of joints such as shoulders, elbows, wrists, and knees. For AI to recognize changes in posture of individuals, it needs to learn from pixel information of images cut out from videos on a frame-by-frame basis, along with the coordinate information of each point, enabling it to recognize changes in posture and expressions. Therefore, it is important to prepare keypoint annotation data using images of various postures and expressions. By doing so, the accuracy of AI in recognizing postures and expressions improves. Additionally, as the number of input points increases, it becomes possible to recognize more complex changes.
Next, we will introduce the reasons why keypoint annotation is gaining attention in posture estimation and facial recognition.
1-1. Pose Estimation and Motion Capture:
Keypoint annotation is used to accurately capture body poses and movements. In applications such as pose estimation and motion capture, it digitizes poses and body movements by capturing changes in nodal points like joints. Traditionally, to capture these movements, it was common to set up multiple cameras to capture the movements of sensors attached to a person. This method requires a well-equipped environment to install measurement equipment, and it involves measuring movements with multiple sensors attached. Due to these constraints, it has been difficult to measure in confined spaces, capture complex movements, or obtain data for multiple target actions.
With the advent of AI, if we can obtain such data through keypoint annotation, it becomes possible to estimate actions from two-dimensional data such as images and videos, enabling posture estimation and motion capture without relying on location or measurement devices. The ability to achieve high accuracy recognition with just one camera has led to advancements in applications for detecting actions in various scenarios.
1-2. Face Recognition:
By inputting points at specific locations on the face, AI can learn variations in expressions and differences in individual facial shapes. By precisely defining points such as the outer corners of the eyes, mouth, cheeks, and jaw, more accurate recognition becomes possible. Utilizing keypoint annotation allows for capturing detailed and complex features of the subject that are difficult to achieve with other annotation methods. Even if bounding box annotation is performed to enclose the entire face of a person, it becomes challenging for AI to recognize the same individual if they are wearing glasses, a mask, or a hat. However, in such cases, if the positions of keypoints like the outer corners of the eyes, nose, jaw, and ears—unique to that person—are captured through image recognition with keypoint annotation, accurate recognition can still be achieved even with just a part of the face.
2. How to Perform Key Point Annotation
In the Key Point Annotation Project, we will proceed with the work through the following steps.
2-1. Data Collection:
We will collect datasets to be used for keypoint annotation. Let's gather data that contains the subjects or movements we want to detect, such as images and videos. Additionally, in the case of human pose estimation, there are ways to utilize existing datasets like the COCO dataset.
2-2. Selection of Annotation Tools:
Choose the right tools for key point annotation. There are various tools available, including open-source and commercial options, so let's select tools based on various aspects such as usability, according to the purpose and situation. Depending on the scale of the project, it is also recommended to choose tools that come with project management features.
2-3. Setting Points:
Determine the input points for the target you want to train (annotation specifications). Depending on the target, decide how to mark the points to obtain the optimal data. Consider creating definitions and guidelines to ensure consistency during the annotation process. Additionally, if possible, it would be even better to perform annotations on a small amount of data before actually starting the work and test it with AI.
2-4. Implementation of Annotations:
Use the annotation tool to annotate the specified points on the image. It is important to annotate each point accurately. However, pursuing accuracy too much can be time-consuming and make it difficult to prepare large amounts of data. Therefore, it is advisable to set a margin of error in advance. Additionally, as you progress with the work, there may be cases where you are unsure of your judgment or where examples do not fit the guidelines or definitions. For these quality-determining factors, it is important to designate a responsible person and make efforts to ensure quality, such as updating the guidelines.
2-5. Data Verification and Correction:
We will review the annotated data and make corrections as necessary. It is important to establish a system to ensure quality, such as setting the frequency of checks according to data quality requirements and, if necessary, appointing dedicated personnel for QA checks.
3. Summary
As we have seen so far, keypoint annotation is applied in various fields of image recognition, particularly in posture estimation and face recognition. Applications in business include detecting abnormal behavior of drivers in the transportation industry and analyzing human motion in sports, healthcare, and education. By utilizing AI trained on keypoint annotation data, it will be possible to solve various challenges that were difficult to address with conventional technologies.
To ensure the accuracy of AI recognition, it is essential to create precise annotations and high-quality datasets. If it is difficult for your company to carry out keypoint annotation data creation in-house, utilizing external vendors can also be an effective method.
4. Human Science Annotation Agency Services
Track record of creating 48 million pieces of training data
At Human Science, we participate in AI development projects across various industries, including natural language processing, medical support, automotive, IT, manufacturing, and construction. To date, we have provided over 48 million high-quality training data through direct transactions with many companies, including GAFAM. We handle a wide range of annotation projects, from small-scale projects to long-term large-scale projects with 150 annotators, regardless of the industry. If your company wants to implement AI but doesn't know where to start, please feel free to consult with us.
Resource management without crowdsourcing
At Human Science, we do not use crowdsourcing. Instead, projects are handled by personnel who are contracted with us directly. Based on a solid understanding of each member's practical experience and their evaluations from previous projects, we form teams that can deliver maximum performance.
Utilizing the latest data annotation tools
One of the annotation tools introduced by Human Science, AnnoFab, allows customers to receive progress checks and feedback from the cloud even during the project. By ensuring that work data cannot be saved on local machines, we also take security into consideration.
Secure room available on-site
At Human Science, we have a security room that meets ISMS standards within our Shinjuku office. This allows us to handle even highly confidential projects on-site while ensuring security. We consider the protection of confidentiality to be extremely important for all projects. Our staff undergoes continuous security training, and we exercise the utmost caution in handling information and data, even for remote projects.