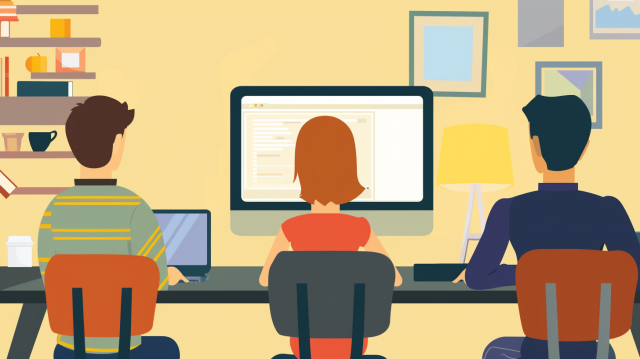
- Table of Contents
-
- 1. What is Curation? Its Basic Meaning
- 2. The Importance of Curation in the AI Era
- 3. Curation Services in Building Generative AI/LLM Models
- 3-1. Curation tasks necessary for building generative AI/LLM models
- 3-2. Differences from Annotation – Data Scrutiny vs. Data Labeling
- 4. Key Points and Challenges of Curation Work in Building Generative AI/LLM Models
- 4-1. Points to Note in Curation Services
- 4-2. Challenges When Conducting Curation Work In-House
- 5. Summary: Request for Curation to Human Science
1. What is Curation? Its Basic Meaning
"Curation" refers to the process of collecting, selecting, and organizing information, and then reassembling it based on specific purposes or perspectives. Originally, it referred to the work of curators who select and arrange exhibits in museums and galleries.
Today, due to the widespread use of the internet, we are in an era overflowing with vast amounts of information. The rise of social media platforms like Instagram and TikTok has accelerated the speed of information dissemination, increasing the effort required to find necessary information. Therefore, "curation" has taken on a new meaning.
To enable users to quickly access valuable information they seek, the demand for "curation media" and "curation sites" that organize information along various themes has been increasing, and they are being utilized in a wide range of fields such as gourmet, fashion, and beauty.
2. The Importance of Curation in the AI Era
In recent years, advancements in generative AI and LLMs (large language models) have led to sophisticated automation utilizing vast amounts of data. However, for AI to produce highly accurate results, it is crucial to consider "what data to train on." This is where the role of "curation" becomes essential.
AI learns from the data provided and generates output. However, if data that has been collected haphazardly is input as is, it may contain misinformation, bias, and noise. For example, if biased information is learned, the risk increases that the AI will make incorrect judgments or generate inappropriate expressions.
By conducting curation, we can select reliable information and ensure data consistency and accuracy, contributing to the improvement of AI quality.
3. Curation Services in Building Generative AI/LLM Models
Curation is a crucial process that affects the quality of data in the development of generative AI and LLMs. The accuracy and reliability of the model heavily depend on the type of data it is trained on, making the process of collecting, organizing, and selecting appropriate data essential. Here, we will explain the specific content of curation tasks in LLM model building and the differences from annotation.
3-1. Curation tasks necessary for building generative AI/LLM models
The development of LLMs involves training on large amounts of text data to achieve natural text generation and advanced reasoning capabilities. However, simply gathering a large amount of data is not enough; noise removal and quality control are essential. Therefore, the curation process includes the following steps.
1. Data Collection
・The task of collecting training data suitable for AI.
・Data is obtained from various sources such as the web, books, papers, corporate data, FAQs, etc.
・It is important to consider rights issues (copyrights and licenses) during data collection.
2. Data Preprocessing (Preprocessing)
・To ensure that AI can learn appropriately, unify the format and remove unnecessary elements.
Example: Removal of HTML tags and special characters, normalization of line breaks and spaces, removal of unnecessary metadata
3. Data Filtering (Selection)
・Select only data suitable for learning, excluding low-quality data and biased data.
Example: Elimination of misinformation and spam data, removal of duplicate data, and removal of short or noisy data that AI cannot learn from properly.
④ Data Cleansing (Quality Improvement)
・Correcting typos and grammatical errors, and revising unnatural sentences.
・Standardizing specific industry terms and technical jargon to maintain consistent data.
Example: Standardizing "AI", "artificial intelligence", and "machine learning"; unifying the notation of "Co., Ltd." and "(株)".
By properly executing a series of processes from data collection to filtering and cleansing, the quality of learning for LLMs can be significantly improved.
3-2. Differences from Annotation – Data Scrutiny vs. Data Labeling
Curation and annotation are often confused, but there are clear differences between the two.
Item | Curation | Data annotation |
Purpose | Data Selection and Organization | Labeling Data |
Work Content | Removal of unnecessary data, formatting, quality improvement | Perform specific tagging and classification |
Example | – Exclude inappropriate text – Remove noise data |
– Add emotional tags to the text – Label images with tags like "dog" and "cat" |
Scope of Application | Quality Control of the Entire Learning Data for LLM | Data creation for specific tasks (classification, translation, for dialogue AI, etc.) |
In particular, in LLM development, the process begins with curation to select and organize appropriate training data, followed by annotation as needed.
In this way, curation is the process of preparing the foundation of data that AI learns from, while annotation is the process of adding additional information to the data to clarify the task.
4. Key Points and Challenges of Curation Work in Building Generative AI/LLM Models
I believe you have found that the output accuracy and reliability of LLM models have greatly improved in curation tasks, but there are various challenges in curation work, and a strategic approach is necessary for efficient implementation. Here, we will explain the points to keep in mind during this process and the challenges when operating in-house.
4-1. Points to Note in Curation Services
When curating, it is important to keep several key points in mind.
①Automation vs. Manual Curation
Advantages of Automation: Capable of processing large amounts of data in a short time
Advantages of Manual Curation: Ability to understand context and judge nuances
By utilizing automated tools, large amounts of data can be filtered quickly, but there is a risk of incorrect data remaining if fully relied upon. On the other hand, while manual curation has high accuracy, it faces challenges in handling large-scale data. The optimal method is a hybrid operation of initial screening by machines + final confirmation by humans.
② Management of Bias
When biased data is learned, the model's output will also be biased.
There is a risk of unintentionally learning discriminatory or inappropriate expressions.
For example, if the model is trained on data biased towards a specific country or culture, it may generate responses lacking diversity. Therefore, it is important to be aware of the balance of data during the curation stage and to ensure the availability of fair information.
③Challenges of Scale (Handling Large-Scale Data)
Curating millions to hundreds of millions of data points is not easy.
It is necessary to build a scalable data processing pipeline.
To manage large volumes of data while maintaining high-quality data, it is essential to utilize automation tools and distributed processing technologies. Additionally, sampling methods for quality checks are also effective.
4-2. Challenges When Conducting Curation Work In-House
It is possible to in-house the curation operations, but there are several significant challenges that come with it.
① Data Volume Issues
– Managing millions to billions of data points requires vast storage and processing power
– The task of extracting useful information from the collected data is enormous
As data increases, storage costs and processing loads rise, necessitating the use of cloud environments and distributed processing frameworks.
②Resource Shortage (Human and Technical Costs)
– Skilled personnel capable of properly processing large amounts of data are needed
– It is difficult to secure resources such as machine learning engineers and data scientists
In particular, since personnel with specialized knowledge to ensure data quality are required, outsourcing may be an option if sufficient resources cannot be secured.
③ Lack of Expertise
– It is necessary to understand the characteristics of data suitable for AI
– Regulatory and ethical considerations (such as GDPR, CCPA) must also be taken into account
If there is a lack of knowledge in appropriate data collection and selection, the quality of the training data may decline, which could result in a decrease in the accuracy of the model.
By leveraging the expertise of data specialists and external professionals, higher quality curation can be achieved.
5. Request for curation to Human Science
A rich track record of creating 48 million pieces of training data
At Human Science, we are involved in AI model development projects across various industries, starting with natural language processing, including medical support, automotive, IT, manufacturing, and construction. Through direct transactions with many companies, including GAFAM, we have provided over 48 million high-quality training data. We handle a wide range of training data creation, data labeling, and data structuring, from small-scale projects to long-term large projects with a team of 150 annotators, regardless of the industry.
Resource management without using crowdsourcing
At Human Science, we do not use crowdsourcing; instead, we advance projects with personnel directly contracted by our company. We form teams that can deliver maximum performance based on a solid understanding of each member's practical experience and their evaluations from previous projects.
Supports not only curation and annotation but also the creation and structuring of generative AI LLM datasets
In addition to labeling and annotation for identification systems for data organization, we also support the structuring of document data for the construction of generative AI and LLM RAG. Since our founding, we have been engaged in manual production as a primary business and service, leveraging our unique know-how gained from a deep understanding of various document structures to provide optimal solutions.
Equipped with a security room in-house
At Human Science, we have a security room that meets ISMS standards within our Shinjuku office. Therefore, we can ensure security even for projects that handle highly confidential data. We consider the protection of confidentiality to be extremely important for all projects. Even for remote projects, our information security management system has received high praise from our clients, as we not only implement hardware measures but also continuously provide security training to our personnel.