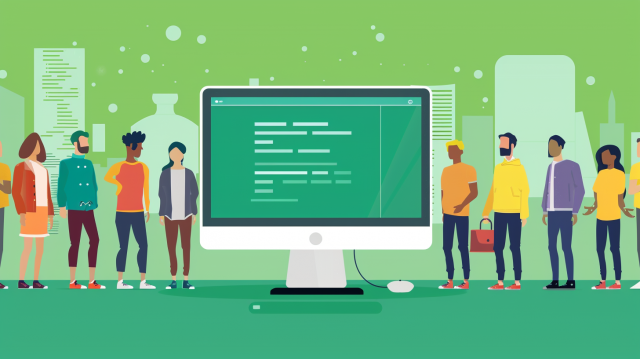
In recent years, the evolution of AI technology has made the use of NLP (Natural Language Processing) common in our lives and businesses. The proliferation of search engines, chatbots, and translation tools has significantly changed the way we access information and communicate.
In particular, the emergence of LLMs (Large Language Models) has been a revolutionary advancement, allowing AI to understand and generate human language more naturally. However, it has also become known that LLMs have challenges such as "knowledge fixation" and "generation of misinformation." RAG (Retrieval-Augmented Generation) is gaining attention as a technology to overcome these issues.
In this article, we will take a look back at the history of the evolution of NLP, explore the potential and challenges of LLM, and provide a detailed explanation of the mechanism of RAG that overcomes these challenges and its future prospects.
- Table of Contents
-
- 1. What is NLP (Natural Language Processing)?
- 2. The Emergence of LLMs (Large Language Models)
- 3. Challenges of LLM
- 4. RAG (Retrieval-Augmented Generation) to Complement the Weaknesses of LLMs
- 5. Limitations of RAG and Future Challenges
- 6. Summary
- 7. Human Science Annotation, LLM RAG Data Structuring Agency Service
1. What is NLP (Natural Language Processing)?
NLP (Natural Language Processing) is a collective term for technologies that enable computers to understand and process human language. Its history dates back to the 1950s, shortly after the invention of computers. Early NLP primarily used rule-based approaches, analyzing sentences with grammar rules and dictionaries.
However, this method was unable to fully address the diversity of languages and exceptions, leading to challenges in practicality. Subsequently, statistical methods and machine learning were introduced, enabling more advanced language understanding. In recent years, with the evolution of deep learning, models utilizing neural networks have emerged, achieving remarkable progress in fields such as translation, text generation, and speech recognition.
2. The Emergence of LLMs (Large Language Models)
Since around 2018, LLMs (Large Language Models) have emerged, further advancing NLP. In particular, the following models have brought about innovation.
●BERT (Bidirectional Encoder Representations from Transformers)
Enables bidirectional contextual understanding, improving accuracy in search engines and question-answering systems. For example, it can appropriately distinguish between words that cannot be differentiated in hiragana, such as "hashi (chopsticks)" and "hashi (bridge)" based on the surrounding context. However, BERT is primarily suited for classification and extraction tasks and is not suitable for text generation.
●GPT (Generative Pre-trained Transformer)
It has the ability to generate high-quality text and programming code by pre-training on vast amounts of text data available on the internet. Particularly, since GPT-3, its ability to engage in natural conversations and handle a variety of tasks has garnered attention.
LLMs are capable of learning from vast amounts of data, possessing knowledge on a wide range of topics, and generating appropriate responses that take context into account. As a result, many applications such as AI-driven automated responses, summarization, translation, and code completion have been realized.
3. Challenges of LLM
While LLM has powerful language generation capabilities, there are several challenges that exist.
①Knowledge Fixation: Difficulty in Learning Latest Information
LLMs operate based on data available at the time of training, so they cannot reflect new information in real-time. Therefore, re-training (fine-tuning) is necessary to update the model's knowledge.
For example, if you ask, "Who are the Nobel Prize winners in 2024?" and that information is not included in the training data, it cannot generate an appropriate response. In fields such as news, law, and medicine, where up-to-date information is always required, this knowledge fixation poses a significant challenge.
②Unclear Sources: Information Reliability Cannot Be Guaranteed
Although LLMs learn from vast amounts of data, they cannot specify which information they are basing their responses on. Therefore, using LLM outputs directly in fields such as healthcare and law, where reliability is crucial, carries risks.
For example, in medical diagnosis, even if it outputs "This treatment is effective for this disease," without the supporting papers or data, experts cannot evaluate the accuracy of the information.
③ Hallucination: The Risk of Generating Incorrect Information
LLMs can generate natural text based on training data, but they may produce information that does not exist as if it were real. This is called "hallucination."
For example, there are cases where non-existent historical events are described as facts, or fictional papers are presented as evidence.
To overcome these challenges, a new approach called RAG (Retrieval-Augmented Generation) is gaining attention.
4. RAG (Retrieval-Augmented Generation) to Complement the Weaknesses of LLMs
RAG (Retrieval-Augmented Generation) is a method designed to compensate for the weaknesses of LLMs. RAG has a mechanism that allows LLMs to search for external information in real-time and generate text based on that information.
Benefits of RAG
①Utilizing the Latest Information
RAG (Retrieval-Augmented Generation) can retrieve information in real-time from external data sources and generate text based on that, allowing for the use of new data beyond the training point. Traditional LLMs face challenges in handling information about the latest events and new technologies, as they rely on pre-trained data for inference. However, by using RAG, it becomes possible to generate responses while referencing the latest information from news articles, corporate internal databases, research papers, websites, and more, enabling the provision of fresher information. This is particularly advantageous in fields such as healthcare, finance, and law, where the freshness of information directly impacts decision-making.
① Citation Disclosure
RAG can explicitly indicate the sources that serve as the basis for its responses, as it searches for information and generates text based on that content. Traditional LLMs generate text based on vast amounts of data but cannot clearly indicate their sources, making it difficult to assess the accuracy of the information. With RAG, it is possible to include the URLs or titles of the original data along with the search results, allowing users to verify the reliability of the information.
③ Suppression of Hallucinations
RAG prevents the model from relying solely on internal knowledge by referencing external information sources in real-time. As a result, the likelihood of providing accurate, fact-based answers increases.
④ No Need for Model Re-Training
Generally, LLMs require re-training (fine-tuning) to update their knowledge, which incurs significant computational costs and time. By utilizing RAG, external data can be dynamically searched to supplement knowledge, allowing for the reflection of the latest information without re-training the model itself. This enables the provision of up-to-date knowledge while reducing operational costs.
In this way, RAG is gaining attention as a technology that enables more flexible and reliable information provision while complementing the challenges of traditional LLMs.
5. Limitations of RAG and Future Challenges
RAG is a powerful technology that addresses the challenges of LLM, but it cannot be considered a complete solution. There are also limitations as follows.
Limits of RAG
① Dependence on the Quality of Data Being Searched
The effectiveness of RAG greatly depends on the quality of external data sources. For example, if the target data is from noisy websites or unreliable databases, there is a risk of generating responses based on inaccurate information. Additionally, if the search engine's index is not updated or if there is bias in the retrieved data, it may not reflect the latest accurate information. Particularly in fields where accuracy is critical, such as healthcare and law, the selection and management of data sources become crucial elements directly impacting the performance of RAG.
② Processing Load of Search and Generation
RAG has the challenge of increased computational costs due to the addition of a search process compared to traditional LLMs. While traditional LLMs can generate responses instantly based on pre-trained data, RAG involves a dual process where relevant information is first searched from external sources, and then text is generated based on that information.
③ Issues with Search Accuracy
There are challenges related to search accuracy, such as missing relevant information and extracting irrelevant information due to an inability to accurately understand the context.
④Quality of Summaries is Not Guaranteed
RAG generates summaries based on the information searched, but the quality is not always consistent. If the summary is inappropriate, it may change the original meaning of the information. For example, the summarization algorithm may omit important details or misinterpret the information. Problems can particularly arise when summarizing texts that contain complex technical terms or highly context-dependent information.
RAG is a powerful technology that addresses the challenges of LLM, but there are issues regarding the quality of the data being searched, computational costs, search accuracy, and the quality of summaries. To overcome these challenges, it is essential to select data sources, optimize search systems, and develop high-quality summarization algorithms. Companies and research institutions are expected to enhance the practicality of RAG by improving search engines and implementing hallucination suppression technologies.
6. Summary
The evolution of NLP has been greatly accelerated by the emergence of LLMs. However, LLMs have challenges such as "knowledge fixation," "generation of misinformation," and "lack of clarity in sources," making RAG an important technology that is gaining attention as a complement.
RAG overcomes the challenges of LLM by retrieving information in real-time and generating more reliable answers. Its effectiveness has been proven particularly in customer support and providing information in specialized fields.
However, RAG is not a complete solution, and there are challenges regarding the quality of the data being searched and the accuracy of the search, which significantly impact the final generated results. In the future, improvements to search engines, data refinement, and optimization of AI models will be required.
By appropriately combining LLM and RAG, more advanced and reliable natural language processing can be achieved. Focusing on the development of future NLP technologies and flexibly utilizing the latest technologies will be the key to better AI utilization.
7. Human Science Annotation, LLM RAG Data Structuring Agency Service
Over 48 million pieces of training data created
At Human Science, we are involved in AI model development projects across various industries, starting with natural language processing, including medical support, automotive, IT, manufacturing, and construction. Through direct transactions with many companies, including GAFAM, we have provided over 48 million high-quality training data. We handle a wide range of training data creation, data labeling, and data structuring, from small-scale projects to long-term large projects with a team of 150 annotators, regardless of the industry.
Resource management without crowdsourcing
At Human Science, we do not use crowdsourcing. Instead, projects are handled by personnel who are contracted with us directly. Based on a solid understanding of each member's practical experience and their evaluations from previous projects, we form teams that can deliver maximum performance.
Not only for creating training data but also supports the creation and structuring of generative AI LLM datasets
In addition to creating labeled and identified training data for data organization, we also support the structuring of document data for generative AI and LLM RAG construction. Since our founding, we have been engaged in manual production as a primary business and service, leveraging our unique know-how gained from extensive knowledge of various document structures to provide optimal solutions.
Secure room available on-site
Within our Shinjuku office at Human Science, we have secure rooms that meet ISMS standards. Therefore, we can guarantee security, even for projects that include highly confidential data. We consider the preservation of confidentiality to be extremely important for all projects. When working remotely as well, our information security management system has received high praise from clients, because not only do we implement hardware measures, we continuously provide security training to our personnel.