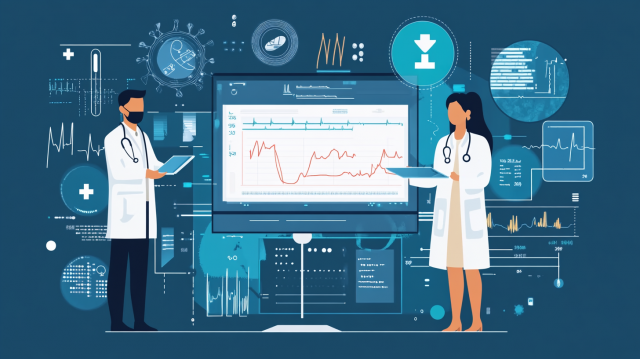
Healthcare is a field that requires extremely high reliability, as it directly affects the lives and health of patients through diagnosis and treatment. In recent years, AI technology in this field has evolved, achieving results in areas such as diagnostic support and pathology image analysis. However, there is also a cautious attitude towards AI in the field. For example, the "black box problem," where it is unclear how AI makes decisions, is a significant concern for healthcare professionals. As the Ministry of Health, Labour and Welfare positions AI as a "support tool that enhances efficiency and presents information in sub-steps of physician-led decision-making within the clinical process" *1, it is a reality that there is resistance to trusting AI's judgments without understanding the reasons behind them in the medical field.
Approaches to solving these challenges include the development of explainable AI (XAI), which focuses on explaining output results in a way that humans can understand, as well as methods that analyze the internal structure of AI models to understand their operating principles. Among these, one of the first steps we can take is to improve the reliability by maximizing the quality of the training data used for AI learning. In this discussion, we will focus on the quality of training data, which is key to enhancing the reliability of medical AI, and the security management that supports it.
*1: Regarding the use of programs that support diagnosis, treatment, etc. using artificial intelligence (AI) and the provisions of Article 17 of the Medical Practitioners Act (Ministry of Health, Labour and Welfare)
Reference link: https://www.pmda.go.jp/files/000227450.pdf
- Table of Contents
-
- 1. Reliable Medical AI Development: Balancing Quality and Safety
- 2. The Importance of Training Data Quality in Medical AI
- 3. The Characteristics of Medical Data and the Importance of Confidentiality
- 4. Points for Effectively Utilizing Appropriate External Vendors
- 5. Summary
- 6. Medical Annotation Services in Human Sciences
1. Reliable Medical AI Development: Balancing Quality and Safety
The performance of AI greatly depends on the quality of the training data used for learning. Therefore, the accuracy of the data and the absence of bias in the cases directly impact the overall performance of the AI. Especially in the medical field, to avoid variability in judgment criteria and the risk of inappropriate assessments, meticulous quality control is required in the creation of training data, focusing on standardization of labeling criteria, consistent judgment, and ensuring clinical validity.
On the other hand, the medical images used as training data contain a lot of personal information and sensitive medical information, making strict security management essential. Specifically, this includes compliance with the Personal Information Protection Act through anonymization, the establishment of data storage and management methods to reduce the risk of unauthorized access and information leakage, and the setting of appropriate access permissions. Strict security measures are required at every stage, from the collection and processing of training data to the implementation of data labeling (annotation), storage, and learning utilization. Thus, to ensure the reliability of medical AI, it is essential to balance high-quality training data with thorough data security management.
2. The Importance of Training Data Quality in Medical AI
AI learns based on the provided training data, so the quantity and quality of the training data are essential. Additionally, securing workers to achieve both within the necessary timeframe is crucial.
However, it is not easy for a professional doctor to dedicate a lot of time to the task of creating training data. Even if we manage to secure about 2 to 3 hours of work time per day for the doctors, it will take a longer period compared to general workers to create the amount of training data necessary for AI to learn. If we increase the number of doctors performing the work, the workload will further increase, including management, as adjustments will be needed in case of scheduling conflicts or differences in opinions among doctors regarding labeling decisions. Additionally, it will naturally incur higher costs compared to general workers.
On the other hand, if only general workers are responsible for the tasks, the issues of cost and work duration are likely to be resolved, but there is a risk that the crucial quality may be neglected, leading to a loss of reliability.
Reference Blog: The Key to Enhancing the Reliability of Medical AI – Training Data and Doctor Review –
It is also important to ensure that there is no discrepancy in quality perception between the development team and the doctors. If there is no role to mediate and adjust opinions between the two parties, there is a possibility that the quality standards aligned with the AI development policy may deviate.
To achieve quality that is validated by doctors, it is necessary to have a project manager who can clearly organize the labeling criteria while appropriately aligning the opinions of both the development team and the doctors.
3. The Characteristics of Medical Data and the Importance of Confidentiality
Medical data requires careful handling as it includes personal health status and diagnostic information. As the application of AI technology progresses in such highly confidential data, privacy protection and security measures are becoming increasingly important.
Handling medical data requires strict compliance with personal information protection laws and related medical regulations. Especially when utilizing data for AI development, thorough anonymization and encryption are essential. Anonymization is a method of processing data in a way that individuals cannot be identified, allowing for the use of data in AI training while protecting privacy. Additionally, when sharing data externally, it is necessary to clarify contracts and strictly manage the purpose of use. By adhering to legal rules, medical institutions and AI developers can reduce risks and enhance reliability.
Vendors involved in the development and operation of medical AI are required to have a high level of security awareness. One of the indicators of this is the ISMS (Information Security Management System) certification, which is an international standard for properly managing information assets and mitigating external threats and internal risks. Vendors that have obtained this certification can be judged to have appropriate rules regarding data handling and are continuously working on improvements.
Furthermore, when outsourcing tasks related to medical data, it is important to properly exchange NDAs (Non-Disclosure Agreements) to prevent the leakage of confidential information. The contract should clearly specify the "scope of confidential information," "data management methods," and "responsibilities in case of violations," and it is necessary to create a system where all parties involved can understand the importance of information management.
4. Points for Effectively Utilizing Appropriate External Vendors
High-quality training data is essential for the development of medical AI. To maintain a balance between cost and delivery time, utilizing the right vendors is effective.
Reference Blog: Challenges and Solutions in Medical AI Annotation - Utilizing Outsourcing Vendors with Expertise
By establishing a system where experienced general workers in medical annotation tasks are primarily involved and checked by several doctors, we can optimize the balance of QCD. This system allows development companies to proceed with development while securing high-quality training data at realistic costs and deadlines.
When utilizing external vendors, it is important to ensure that they have obtained Information Security Management System (ISMS) certification and have signed appropriate Non-Disclosure Agreements (NDAs) as key selection criteria. Additionally, it is essential to verify whether they can meet high-security requirements. In a cloud worker-centric system, remote work is fundamental, and there may be cases where they cannot accommodate data storage via networks or in cloud environments. By confirming their ability to meet security requirements, such as the presence of dedicated security rooms and the feasibility of on-site work at designated locations, you can determine whether they can safely handle valuable medical data.
Utilizing vendors who are well-versed in improving the quality of training data and security management in medical data not only supports the efficient creation of high-quality training data but also provides significant advantages in ensuring strict security measures. Choosing experienced vendors and establishing an appropriate collaboration system can be considered crucial factors that influence the success of medical AI development.
5. Summary
In the field of medical AI, where reliability is highly demanded, high-quality training data and robust security measures are essential. The accuracy of AI learning is greatly influenced by precise and balanced annotations, making it effective to establish a checking system involving general workers with experience in medical annotations and physicians. This allows for balancing costs and timelines while maintaining quality. At the same time, when handling medical data that includes patient information, it is necessary to select vendors that can manage data appropriately, such as those with ISMS certification, and to ensure a dedicated security environment, thus requiring a strict data protection system.
Collaboration with specialized vendors familiar with the quality of training data and security management will enable the development of safe AI systems trusted by the medical field.
6. Human Science Medical Annotation Services
●Extensive annotation experience in medical imaging
Our company has extensive experience in medical image annotation that requires skill transfer, such as surgical images and MRI images, which are of high difficulty and specialization. In addition to experienced project managers in medical image annotation projects, we also have many skilled workers, ensuring high-quality annotation even for projects that are complex, specialized, and require skill transfer.
●Support for physician supervision and annotations by physicians
It can be concerning to have only general workers perform all tasks. In such cases, we receive requests for supervision by a physician for certain check tasks. To meet these requests, we have further strengthened our physician supervision system, allowing us to handle more complex annotations. Additionally, even if you request annotations by physicians instead of general workers, our project managers will provide comprehensive management services, ensuring resource allocation, quality, and progress management.
●Resource management without using crowdsourcing
At Human Science, we do not use crowdsourcing. Instead, projects are handled by personnel who are contracted with us directly. Based on a solid understanding of each member's practical experience and their evaluations from previous projects, we form teams that can deliver maximum performance.
●Equipped with a security room in-house
Within our Shinjuku office at Human Science, we have secure rooms that meet ISMS standards. Therefore, we can guarantee security, even for projects that include highly confidential data. We consider the preservation of confidentiality to be extremely important for all projects. When working remotely as well, our information security management system has received high praise from clients, because not only do we implement hardware measures, we continuously provide security training to our personnel.
Supports not only annotation but also the creation and structuring of generative AI LLM datasets.
In addition to labeling for data organization and annotation for identification-based AI systems, Human Science also supports the structuring of document data for generative AI and LLM RAG construction. Since our founding, our primary business has been in manual production, and we can leverage our deep knowledge of various document structures to provide you with optimal solutions.