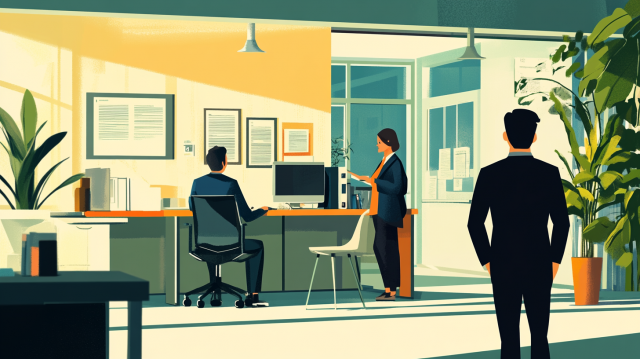
The world of AI is a realm that holds the potential to mimic human intelligence and, at times, surpass it. Among these, discriminative AI and generative AI are attracting attention as the two main pillars of modern AI technology.
Discriminative AI excels in the ability to classify given data and recognize patterns. For example, this includes image recognition and speech recognition. On the other hand, generative AI has the power to create new content, achieving innovative results in fields such as text generation and image generation.
These two AI technologies, while possessing different characteristics, complement each other. The structure in which discriminative AI understands the world and generative AI creates a new world based on that understanding can be said to mimic the process of human intellectual activity. In this blog, we will explain the history and mechanisms of these AI technologies.
- Table of Contents
-
- 1. The History and Evolution of AI
- 2. What is Identification AI: The Power to Distinguish
- 3. What is Generative AI: The Power to Create
- 4. Overview of Supervised Learning, Unsupervised Learning, and Reinforcement Learning
- 5. The quality and quantity of teacher data determine the future
- 6. Summary
- 7. Human Science Annotation, LLM RAG Data Structuring Agency Service
1. The History and Evolution of AI
The history of artificial intelligence (AI) dates back to the 1950s. At that time, with the development of computers, an ambitious goal was set to create machines that could think like humans. The pioneer of this was the proposal of the "Turing Test" by mathematician Alan Turing. He stated that if a machine could converse like a human, it could be considered "intelligent."
In 1956, the term "artificial intelligence" was first used at the Dartmouth Conference, marking the official beginning of AI research. This early AI was built on programs based on logic and rules, designed to solve specific problems such as chess and theorem proving. However, due to limited computational resources and data, it was difficult to handle more complex tasks.
The Emergence of Identification AI
In the 1980s, rule-based AI known as expert systems gained attention, but the application range of AI was still limited. A turning point came between the 1990s and 2000s. During this period, research on machine learning, which forms the basis of identification AI, advanced, and algorithms for classifying images and sounds were developed. For example, in the 2000s, support vector machines (SVM) and decision trees were used, leading to practical applications such as facial recognition and spam email filtering.
The Birth and Evolution of Generative AI
On the other hand, the origins of generative AI can be seen in the fields of natural language processing (NLP) and image generation. Particularly in the 2010s, the evolution of neural networks led to rapid advancements in the development of generative AI. Among these, the introduction of Generative Adversarial Networks (GAN) in 2014 significantly improved the ability to generate images and audio. Additionally, with the emergence of the Transformer model, text generation and translation became much more natural, accelerating the development of conversational AI like ChatGPT, leading us to the present day.
The Role of Deep Learning
The emergence of deep learning has been revolutionary in the development of identification AI and generative AI. In particular, in 2012, the use of deep convolutional neural networks (CNN) in the image recognition competition "ImageNet" achieved results that overwhelmed others, widely recognizing the potential of AI. The combination of large amounts of data and high-performance GPUs has dramatically improved AI's ability to recognize complex patterns.
In generative AI, deep learning has played an important role. Especially in natural language processing, the GPT series based on the Transformer model has achieved the ability to understand context and generate creative text.
2. What is Identification AI: The Power to Distinguish
Identification AI refers to an AI that finds specific patterns within data and utilizes them for classification and recognition. A representative example of this is a task that identifies whether an object in an image is a "cat" or a "dog." In this way, identification AI possesses the ability to make judgments like humans by training its "discriminative power" based on large amounts of data.
Identification AI often uses a method called "supervised learning." This involves training a model on labeled data (for example, data labeled as "dog" for images of dogs and "cat" for images of cats) so that it can predict the correct labels for unknown data.
●Examples of AI Utilization
Identification AI is widely applied in our daily lives and industries. Below are some representative examples.
Face Recognition
It is used for unlocking smartphones and in facial recognition systems for security cameras. AI compares the features of a human face (such as the positions of the eyes, nose, and mouth) with a database to determine if there is a match.
Medical Imaging Diagnosis
In the medical field, AI that analyzes X-ray images and MRI data to identify lesions is being utilized in some medical institutions. For example, systems that support early detection of cancer and diagnosis of cerebral infarction have been put into practical use.
Autonomous Driving
AI identifies signals and pedestrians from images and real-time videos captured by in-vehicle cameras, supporting safe driving.
3. What is Generative AI: The Power to Create
Generative AI refers to AI that has the ability to generate new data and content. While traditional discriminative AI is focused on "recognizing something," generative AI aims to "create something" in response to human requests. Generative AI produces unique outputs while mimicking tasks that humans perform creatively, such as language, images, music, and videos.
The basic mechanism utilizes neural networks and deep learning, with particularly important roles played by Generative Adversarial Networks (GANs), autoregressive models, and Transformer models.
Examples of Utilizing Generative AI
Text Generation
ChatGPT is a prime example of generative AI that applies natural language processing technology. It can generate appropriate responses to text input by users. It is used for a wide range of applications, from casual conversations to creating business documents and suggesting programming code.
Image Generation
Generative AIs like DALL·E and Stable Diffusion can create images based on prompts input by users. For example, they can respond to instructions such as "Draw a picture of a cat wearing a spacesuit," allowing for the rapid creation of creative visual content.
Music and Video Generation
With music generation AI, you can automatically create songs tailored to specific genres or moods. Additionally, video generation AI can produce animations and short videos from simple scripts.
4. Learning Methods Supporting Identification AI and Generative AI
The key that supports the performance of identification AI and generative AI is the "learning method." For AI to be able to perform tasks, it needs to learn based on vast amounts of data. Here, we will explain the common AI learning methods: "supervised learning," "unsupervised learning," and "reinforcement learning."
Supervised Learning
Supervised learning is a method of training AI using data that has been assigned "correct labels." For example, labels such as "dog" and "cat" are attached to image data, and based on that information, the AI learns patterns. After training, it will be able to predict the correct labels for unknown data.
Unsupervised Learning
Unsupervised learning is a method that autonomously learns the structure and patterns of data using unlabeled data. It is used in tasks such as cluster analysis and dimensionality reduction.
Reinforcement Learning
Reinforcement learning is a method by which AI learns through interactions with the "environment." AI learns optimal strategies while receiving rewards and penalties for its actions. This approach is suitable for tasks that aim to maximize long-term benefits.
5. The quality and quantity of training data determine performance
One of the biggest factors that influence AI performance is the "quality" and "quantity" of the training data. For AI to perform tasks accurately and efficiently, appropriate training data is essential. Here, we will explain the impact of training data on AI performance and the importance of labeling.
●The Impact of Training Data on AI Performance
AI models learn based on the data provided. Therefore, the higher the quality of the training data, the better the performance of the AI. Specifically, the following elements are important:
Diversity of Data:
It is essential for the training data to have diversity. For example, in the case of facial recognition AI, data that covers a wide range of factors such as gender, age, and race is necessary.
Amount of Data:
For AI to learn accurately, a large amount of data is required. If the amount of data is insufficient, the model's generalization performance will decrease, and it will not be able to operate accurately on unknown data.
Data Accuracy:
In supervised learning, it is essential that the data is accurately labeled. For example, in medical AI, the more accurate the labels are, the higher the reliability of the diagnosis. Inaccurate labels can lead to incorrect learning by the model and may cause misjudgments.
6. Summary
Up to this point, we have explained the differences between identification AI and generative AI, as well as the importance of the training data required for these AIs. When developing AI, it may not be practical in terms of time and cost to prepare high-quality training data in-house. In such cases, how about considering outsourcing the creation of training data?
7. Human Science Annotation, LLM RAG Data Structuring Agency Service
Over 48 million pieces of training data created
At Human Science, we are involved in AI model development projects across various industries, starting with natural language processing, including medical support, automotive, IT, manufacturing, and construction. Through direct transactions with many companies, including GAFAM, we have provided over 48 million high-quality training data. We handle a wide range of training data creation, data labeling, and data structuring, from small-scale projects to long-term large projects with a team of 150 annotators, regardless of the industry.
Resource management without crowdsourcing
At Human Science, we do not use crowdsourcing. Instead, projects are handled by personnel who are contracted with us directly. Based on a solid understanding of each member's practical experience and their evaluations from previous projects, we form teams that can deliver maximum performance.
Not only for creating training data but also supports the creation and structuring of generative AI LLM datasets
In addition to creating labeled and identified training data for data organization, we also support the structuring of document data for generative AI and LLM RAG construction. Since our founding, we have been engaged in manual production as a primary business and service, leveraging our unique know-how gained from extensive knowledge of various document structures to provide optimal solutions.
Secure room available on-site
Within our Shinjuku office at Human Science, we have secure rooms that meet ISMS standards. Therefore, we can guarantee security, even for projects that include highly confidential data. We consider the preservation of confidentiality to be extremely important for all projects. When working remotely as well, our information security management system has received high praise from clients, because not only do we implement hardware measures, we continuously provide security training to our personnel.