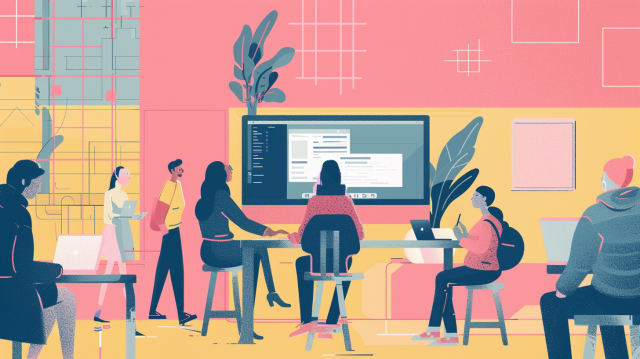
In recent years, the technologies of AI and machine learning have rapidly advanced, and the importance of datasets is increasing. In particular, annotated open datasets play a crucial role in many machine learning projects. However, there are both advantages and disadvantages to their use. Here, we will introduce three representative annotated open datasets and discuss their merits and demerits.
- Table of Contents
1. What is an Open Dataset?
One of the main methods of AI learning, known as "supervised learning," requires annotated training data. However, a large amount of data, ranging from thousands to tens of thousands, is necessary for learning. Preparing this data in-house can be extremely time-consuming and labor-intensive. While it is a crucial element of AI research and development, data collection and annotation work can become a bottleneck in terms of time and cost as research and development projects progress. To alleviate the burden of data collection and annotation work and contribute to the promotion and advancement of AI development, there are institutions and organizations that publicly release annotated datasets for free. These publicly available datasets are known as open datasets.
3 Selected Open Datasets
Open datasets are available in various forms, and here we will introduce three representative datasets from institutions and sites that publish them.
UC Irvine Machine Learning Repository
This is an online repository of datasets provided by the University of California, Irvine, that can be used for research and experiments in machine learning. This repository contains various datasets that can be used for evaluating machine learning algorithms and developing new methods.
The COCO (Common Objects in Context) dataset is a large-scale dataset for object detection, segmentation, and captioning. It is designed to encourage research across various categories and is commonly used for benchmarking computer vision models.
This is a platform for hosting competitions and projects in data science and machine learning. Various open datasets are available. Additionally, there are many open-source AI models that serve as valuable resources to support the data science and machine learning community.
3. Advantages and Disadvantages
These datasets are available for free, but it is important to be aware of their advantages and disadvantages when using them.
Benefits
Reduce development costs
As mentioned earlier, preparing your own training data is very difficult. Open datasets do not require this step, and you can start learning as soon as you obtain the data, which allows for significant cost reduction.
◯Securing Diverse Data
It is important to prepare various types of data for AI training. If training is done only with similar types of data, the recognition accuracy will improve for those similar types, but it will lead to a state called "overfitting," where the accuracy does not improve for other types of data. By utilizing open datasets that contain diverse data, we can expect improvements in AI recognition accuracy.
Disadvantages
◯Variation in Quality
When it comes to the quality of annotations, open datasets cannot necessarily be considered high quality. Caution is required as they may contain labeling errors and low-accuracy annotations.
Unable to find data suitable for the purpose
Open datasets are often published for the purpose of competing in AI performance in competitions or sharing within the development community, and most of them are general-purpose datasets. In terms of image classification annotations, they can be considered datasets where "broad classification" is performed. For example, if the goal of AI development is to recognize tomato varieties, there may be open datasets that label types such as eggplants and tomatoes (broad classification), but there may not be datasets that specifically label tomato varieties (narrow classification).
4. Summary
Open datasets can help reduce costs and shorten development periods during the validation phase, such as Proof of Concept (PoC), if appropriate data can be obtained, allowing for an effective development cycle. However, to further advance development and improve AI recognition accuracy, it is necessary to have training data that aligns with those objectives. This may require data collection and annotation work as needed. For organizations or companies whose main business is development, conducting such annotations in-house can not only be a significant burden in terms of labor shortages and costs but can also lead to issues with AI recognition accuracy due to a lack of know-how and experience in annotation. In such cases, opting to outsource to a crowdsourcing platform or specialized vendors can be a good choice. Our company has extensive experience and a proven track record in both image annotation and natural language annotation. If you are considering outsourcing, please feel free to consult with us.
5. Human Science Annotation and Data Labeling Services
Over 48 million pieces of training data created
At Human Science, we participate in AI model development projects across various industries, including natural language processing, medical support, automotive, IT, manufacturing, and construction. To date, we have provided over 48 million high-quality training data through direct transactions with many companies, including GAFAM. We handle a wide range of annotation and data labeling, from small-scale projects to long-term large projects with 150 annotators, regardless of the industry.
Resource management without crowdsourcing
At Human Science, we do not use crowdsourcing. Instead, projects are handled by personnel who are contracted with us directly. Based on a solid understanding of each member's practical experience and their evaluations from previous projects, we form teams that can deliver maximum performance.
Support for various data according to your needs
We handle a variety of input and output data, from labeling attributes of large amounts of unorganized and uncategorized data such as videos and compiling them into Excel or CSV, to adding label information to images and text data and describing them.
Secure room available on-site
At Human Science, we have a security room that meets ISMS standards within our Shinjuku office. This allows us to handle even highly confidential projects on-site while ensuring security. We consider the protection of confidentiality to be extremely important for all projects. Our staff undergoes continuous security training, and we exercise the utmost caution in handling information and data, even for remote projects.