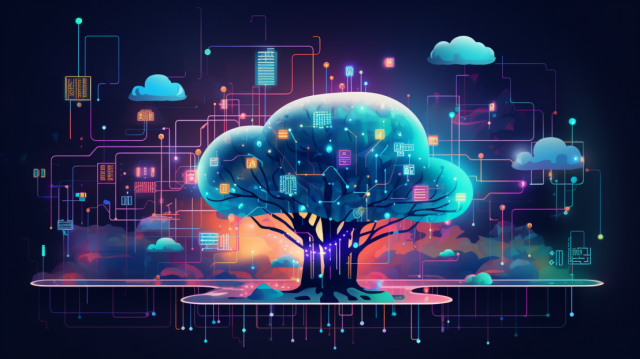
There are various methods for AI development. Among them, the emergence of a method called deep learning has significantly advanced the recognition accuracy of AI. The application range of AI has also expanded to all fields, including manufacturing, services, healthcare, and education, making it an indispensable presence in society.
In deep learning, it is necessary to provide a large amount of data to the AI. By doing so, the AI learns to find patterns that match the objectives from the vast amount of data. While supervised learning is often used for AI training, in this case, data known as training data is required. Training data is created through a process called data labeling, and this time, we will explain data labeling, its mechanism, and the market size worldwide.
- Table of Contents
-
- 1. What is Data Labeling?
- 1-1. Definition and Characteristics of Data Labeling
- 1-2. What is the difference from annotation?
- 1-3. Differences in the Use of Terms Between Japan and Overseas
- 2. Market Size of Data Labeling
- 2-1. Current Market Growth Rate
- 2-2. Forecast of Future Trends in the Data Labeling Market
- 2-3. Relevance to Next-Generation Technologies and Industry Development
- 2-4. The Impact of Increased Demand for Data Labeling
- 3. What are the benefits of outsourcing data labeling services?
- 3-1. Possibilities for Cost Reduction and Efficiency Improvement
- 3-2. Utilization of Specialized Knowledge and Skills
- 3-3. Points to Consider When Outsourcing Data Labeling Services
- 4. Achievements in Human Science
- 5. Data Labeling Outsourcing Services for Human Sciences
1. What is Data Labeling?
Data labeling, which is essential for "supervised learning," refers to the process of assigning labels to data, as the term suggests. But what does it mean to label data? Here, we will explain the mechanism of data labeling.
1-1. Definition and Characteristics of Data Labeling
Data labeling is the process of marking (labeling) the subjects that you want AI to recognize within the data. There are various types of labeling methods. For example, in image labeling, there are methods such as "bounding boxes" that enclose the subject in a rectangle, "key points" that mark specific positions of the subject, and "segmentation" that colors the outline of the subject. In text labeling, there are methods to select the relevant parts of the text (words, sentences, paragraphs, etc.) using underlining or highlighting, as well as methods to label the entire text as a whole. Although the purposes differ, the comment function in Word documents can also be considered a form of labeling. The assigned labels include types and information (referred to as classes) that indicate what the subject is, such as "car," "person," or "signal." The labeled data then becomes the training data used for AI learning.
1-2. What is the difference from annotation?
Many of you may have seen the explanation that involves performing "annotation" to create training data. Our company also has a blog that explains "annotation."
>>What is Annotation? An explanation of its meaning and its relationship with AI and machine learning.
Although "data labeling" and "annotation" may seem to have different meanings at first glance, they can be considered the same in terms of creating training data. However, as English words, they also have distinct meanings. Since methods of AI learning, such as deep learning, have primarily developed in English-speaking regions (especially the United States), let's take a look at how these two terms are treated in English.
1-3. Differences in the Use of Terms Between Japan and Overseas
In English, "annotation" originally means "to add notes or comments to a text." For example, it refers to marking relevant parts of a text with symbols like "*" or underlining, and adding annotations. When creating training data, similar tasks are performed by marking the data and assigning classes, which is why it is called "annotation." On the other hand, "data labeling" also involves similar tasks, such as attaching price tags or labels to products to provide information like product names and prices, and is used when creating training data as well.
When you search for the word "annotation" in English on the web, content related to writing and proofreading, such as "methods for adding annotations to text," tends to rank highly. In contrast, "data labeling" brings up AI-related content, so it seems that "data labeling" is more commonly used to refer to the creation of training data. For example, in the United States, there are tool names for creating training data, such as "labelimg" and "labelme," which evoke the concept of labeling. Additionally, the blog of a company called Superannotate, which provides online tools, frequently uses the expression "be labeled."
That said, both "data labeling" and "annotation" can be considered the same term referring to the task of creating training data. However, since "data labeling" evokes a more specific action, it seems to be used more frequently at the level of tool operation instructions and work procedures for training data. Additionally, the workers who create training data are referred to as "data labelers".
On the other hand, in Japan, the process of creating training data is often referred to as "annotation." The workers who create the training data are commonly called "annotators" in Japan.
2. Market Size of Data Labeling
Various reports have been released regarding the market size of data labeling, and the figures for market size differ among them. However, all reports indicate that the data labeling market is on an upward trend. Here, we will refer to a report released by Markets and Markets in February 2023 and an analyst note released by UBS in July 2023 to explain the market size of data labeling.
2-1. Current Market Growth Rate
As of 2022, the global market size for data labeling is 800 million USD. According to the report, it is expected to expand to 3.6 billion USD by 2027, which represents an average annual growth rate of 33.3%.
2-2. Future Trends and Forecasts of the Data Labeling Market
The market size for data labeling continues to show an expanding trend, which has been reported in other reports besides Markets and Markets. Factors contributing to this expansion include the broadening scope of AI applications due to advancements in AI technology. Among these, a significant factor for growth is the increasing demand in medical imaging. It is anticipated that there will be applications in image diagnostics that do not require medical professionals, the introduction of medical robots, and document searches for various medical records, papers, and documents issued during new drug development (where natural language processing technology enhanced with medical terminology, in addition to AI OCR, will be necessary).
2-3. Relevance to Next-Generation Technologies and Industry Development
UBS, a major Swiss bank, in an analyst note released on July 25, 2023, raised its long-term AI demand forecast from an average annual growth rate of 20% over the previous five years from 2020 to an average annual growth rate of 61% over the next five years starting from 2022. This is believed to be a forecast considering the rapid adoption of generative AI, represented by ChatGPT. Such developments in AI are recognized as a long-term trend rather than a transient growth similar to an AI bubble.
>>UBS predicts an average annual growth rate of 61% for AI demand from 2022 to 2027
Data labeling is expected to play an important role in the learning of AI, even in the field of generative AI, just as it has in the past. Considering the expansion of its use in industries such as healthcare mentioned earlier, it can be said that the scope of AI requiring data labeling will continue to expand in the future.
>>What AI and Machine Learning Can Do: 12 Use Cases by Industry.
2-4. The Impact of Increased Demand for Data Labeling
The increasing demand for data labeling will create new job opportunities. Data labeling can be done remotely depending on the security level, allowing for a wide range of talent to be secured without being limited by region or time zone.
On the other hand, if the data contains information related to privacy or security, there are concerns about leaks or breaches if the data is not handled in an appropriate environment. In particular, in the field of medical data labeling, which is expected to see increased demand in the future, it is believed that handling such data will be common. Especially for data labeling vendor companies, it is increasingly required not only to focus on building a secure remote work environment to ensure robust security management but also to prepare security rooms for on-site responses and to be able to accommodate client on-site presence.
3. What are the benefits of outsourcing data labeling services?
Data labeling currently requires human intervention. Moreover, since labels must be applied to a vast quantity of data ranging from thousands to hundreds of thousands, it often takes weeks to months to complete. In companies engaged in AI development, there are cases where development engineers handle this labeling, but this can encroach on the time needed for their primary development tasks. Here, we will explain the benefits of outsourcing data labeling tasks.
3-1. Possibilities for Cost Reduction and Efficiency Improvement
Data labeling, unlike AI development tasks, does not require programming skills or specialized knowledge in AI engineering. Furthermore, it is a task that can take up a significant amount of time in the AI development process. If engineers perform this task, it incurs costs unrelated to the core development work. Additionally, even if a company secures personnel for labeling, it can become a waste if labeling tasks do not arise. Therefore, considering the effort involved in securing personnel and managing data labeling, outsourcing to specialized vendors instead of performing labeling in-house can be said to be the greatest advantage in terms of cost reduction and operational efficiency.
3-2. Utilization of Specialized Knowledge and Skills
Data labeling does not require the expertise of engineering and other skills necessary for AI development, but to maintain data quality and meet deadlines with high productivity, appropriate management skills, as well as expertise and know-how related to labeling, are essential. By outsourcing to external vendors, you can leverage the unique expertise and know-how specific to data labeling.
3-3. Points to Consider When Outsourcing Data Labeling Services
When outsourcing, we recommend keeping the above benefits in mind and discussing with multiple vendors, including their track record and quality assurance in data labeling that aligns with your company's AI development goals, as well as security measures. For reference, please also check out the following blog from our company.
4. Achievements in Human Science
We would like to introduce interview articles featuring feedback from our clients based on our past achievements. Please take a look.
>>Achieving fast and accurate annotation work through outsourcing - Ensuring the accuracy and reliability of machine learning systems - (Sumitomo Heavy Industries)
5. Data Labeling Outsourcing Services for Human Sciences
A rich track record of creating 48 million pieces of training data
At Human Science, we participate in AI model development projects across various industries, including natural language processing, medical support, automotive, IT, manufacturing, and construction. To date, we have provided over 48 million high-quality training data through direct transactions with many companies, including GAFAM. We handle a wide range of annotation projects, from small-scale projects to long-term large-scale projects with 150 annotators, regardless of the industry. If your company wants to implement AI models but doesn't know where to start, please feel free to consult with us.
Resource management without using crowdsourcing
At Human Science, we do not use crowdsourcing; instead, we advance projects with personnel directly contracted by our company. We form teams that can deliver maximum performance based on a solid understanding of each member's practical experience and their evaluations from previous projects.
Utilizing the latest data annotation tools
One of the annotation tools introduced by Human Science, AnnoFab, allows customers to receive progress checks and feedback from the cloud even during the project. By ensuring that work data cannot be saved on local machines, we also take security into consideration.
Equipped with a security room in-house
At Human Science, we have a security room that meets ISMS standards within our Shinjuku office. This allows us to handle even highly confidential projects on-site while ensuring security. We consider the protection of confidentiality to be extremely important for all projects. Our staff undergoes continuous security training, and we exercise the utmost caution in handling information and data, even for remote projects.
Related Blog Posts
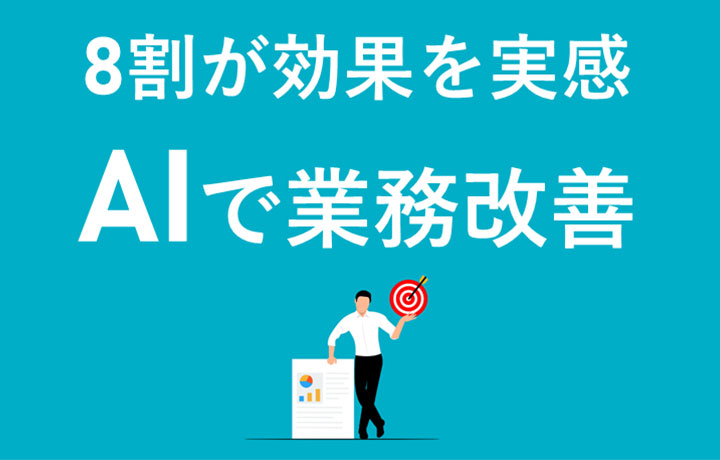