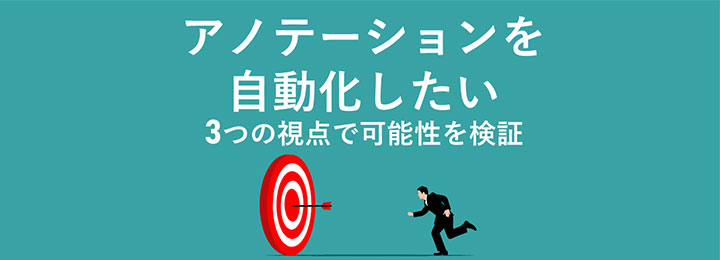
Annotation is essential for AI development, but it still requires a tremendous amount of time and resources. As the demand for annotation automation increases, is its practical implementation realistic? We will consider the challenges faced by current annotation practices and the perspectives needed when contemplating the introduction of automation.
- Table of Contents
-
- 1. The Role of Annotation in AI Development and Its Types
- 1-1. What is Annotation
- 1-2. What kind of data can be annotated?
- 1-3. Image Annotation
- 1-4. Video Annotation
- 1-5. Audio Annotation
- 1-6. Text Annotation
- 2. Challenges of Annotations
- 2-1. It takes a lot of time anyway
- 2-2. The Challenges of Project Management
- 3. Is it possible to automate work using annotation tools?
- 3-1. The Current Situation Where Automation is Difficult
- 3-2. Three Perspectives for Annotation Automation
- 3-3. Reasons to Choose Annotation Proxy Services
- 4. Human Science Annotation Agency Services
- 4-1. Utilizing the latest data annotation tools
- 4-2. Resource Management Without Using Crowdsourcing
- 4-3. Complete Security Room in Our Company
- 4-4. Achievements in Creating 48 Million Teacher Data Entries
1. The Role of Annotation in AI Development and Its Types
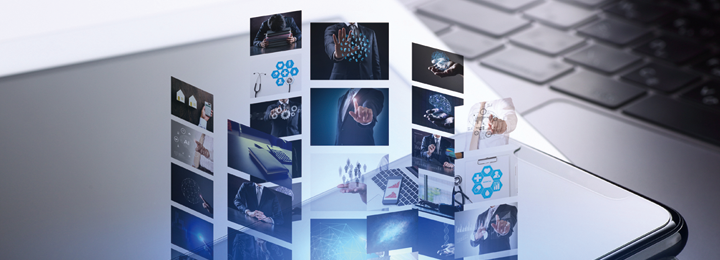
1-1. What is Annotation
To improve the accuracy of AI, machine learning using training data is necessary. The process of creating that training data is called annotation. Information such as tags, labels, and metadata is added as annotations to each piece of data that serves as the material. The accuracy of AI improves as it ingests the training data and recognizes patterns.
This is the positioning of annotation in the AI development process.
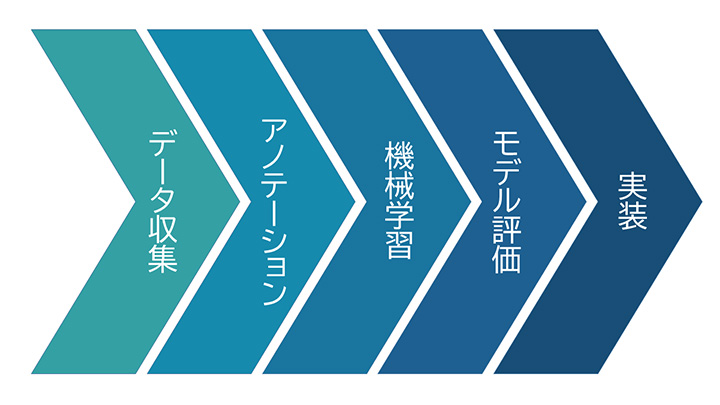
Please refer to the following article for the meaning of annotations.
>>What is an annotation? An explanation from its meaning to its relationship with AI and machine learning.
1-2. What kind of data can be annotated?
Annotations can be divided into several types. Here, we will introduce the annotations that are currently commonly used.
1-3. Image Annotation
Image annotation can be broadly classified into three categories.
・Object Detection
We find objects in images and tag them with meaningful labels such as "human" or "car" according to the subject.
・Region Extraction (Semantic Segmentation)
Select areas within the image and tag them. Specify the meaning of the selected areas, such as "This area is clothing" or "This area is a door."
・Image Classification
Attributes are tagged to the images. Information such as "Is it polka dot or stripe?" and "Is it open or closed?" is added.
1-4. Video Annotation
This is an annotation that shares many similarities with images. Since a video can be viewed frame by frame as images, it can also be said to be a difference in data format. The differences from image annotation include tagging and labeling at the segment level, such as scenes (from what second to what second) in the video.
1-5. Audio Annotation
There are cases where tagging is done for volume and type of sound, as well as cases where tagging is done for the meaning of words spoken by humans. In the latter case, it is common practice to transcribe the speech into text and tag each word. This is utilized in various fields such as voice recognition for smartphones and smart speakers represented by "Hey Siri" and "OK, Google", intent extraction from users, and voice calls in customer service.
1-6. Text Annotation
Annotations allow you to extract specific text from large volumes of documents and aggregate necessary text or phrases from scattered data. Tagging can be performed according to pre-set rules, enabling document identification and content analysis. It is also used to remove inappropriate content. This is utilized in various scenarios such as business documents, manuals, invoices, and contracts.
2. Challenges of Annotations
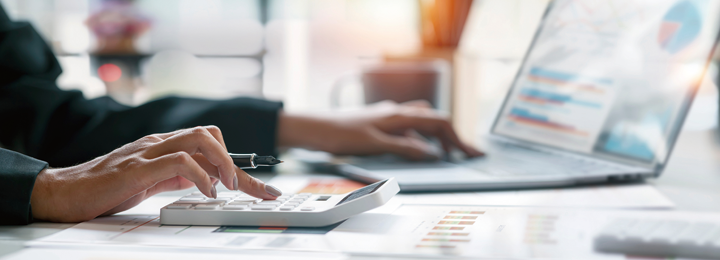
2-1. It takes a lot of time anyway
For any type of annotation, it is necessary for the personnel to manually add information to each piece of data. This work requires considerable care, patience, and a deep understanding and insight into the rules and the target data. Depending on the project, there may be a training period for skill acquisition before the actual work begins. In such cases, it is also necessary to assign personnel to act as trainers. This is a project that requires both a significant amount of work time and resources that are suited to the task.
2-2. The Challenges of Project Management
In annotation projects, the number of workers can exceed several dozen to over 100. The role of the project manager is extremely important in ensuring the quality of the training data. In addition to formulating work guidelines, responding to ongoing questions and specification changes, and managing productivity, the project manager themselves must be proficient in the work.
3. Is it possible to automate work using annotation tools?
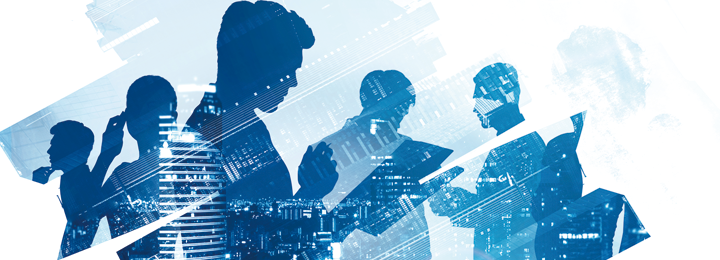
3-1. The Current Situation Where Automation is Difficult
The automation of annotation has significantly improved in accuracy compared to before. However, since most automation is performed by AI that learns from training data, the practical application is inevitably limited to general-purpose and relatively simple tasks with a wide range of applications.
Even with automation, human checks are still necessary, and corrections will occur. Therefore, it is wiser to view it as a means of increasing efficiency rather than true automation.
There is also an approach to train the results of manual annotations to automate future annotations. While this can be an effective means of streamlining the process when a large volume of annotations is required, it still inevitably involves checks and manual corrections. As a result, there are many cases where it ends up taking more time than doing everything manually, depending on the workload.
Recent trends in the development of identification AI have shifted from general-purpose and relatively simple systems to more complex and specialized fields. It must be said that automation in specialized areas is still quite challenging, and realistically, the scope of automation is still quite limited.
3-2. Three Perspectives for Annotation Automation
When considering the automation of future annotations, it is necessary to fully consider the following perspectives.
・Can automated annotation in that field achieve practical accuracy?
・What level of efficiency can be expected from automation?
・Is the risk of rework or corrections within an acceptable range?
Currently, we have attempted to automate annotations, but often require human intervention for corrections, and in some cases, it has been more efficient to perform the work manually from the beginning. To avoid this, it is important to consider various factors, such as the accuracy of automation and the labor required for corrections, from multiple perspectives to determine which method to use.
3-3. Reasons to Choose Annotation Proxy Services
As mentioned so far, achieving high-quality annotations currently requires both time and manpower. It is not only the annotators responsible for the work, but also the roles of trainers, checkers, and project managers who manage the cases. It may be realistically difficult to achieve the objectives while relying solely on in-house resources. Therefore, it is common for many companies to outsource annotation projects to external service providers. However, the project management systems can vary significantly depending on the outsourcing partner. The differences in systems directly affect the quality of the training data, so careful selection of the outsourcing partner is necessary.
4. Human Science Annotation Agency Services
4-1. Utilizing the latest data annotation tools
At Human Science, we are constantly introducing the latest annotation tools to further enhance quality and work efficiency. One of the tools we have implemented, AnnoFab, allows for progress checks and reviews of deliverables during the project. It is also possible to mechanically detect work omissions and common mistakes using the check function. Real-time communication with annotators is possible, enabling immediate notification of changes or additions to standards and rules. We also flexibly accommodate new methods such as 3D annotation. By ensuring that work data cannot be saved on local machines, we also take security into consideration.
4-2. Resource Management Without Using Crowdsourcing
The initiatives for efficiency considered by Human Science go beyond just reviewing work processes and selecting annotation tools. It also involves thinking from the essence of the project and assigning tasks to resources that match its characteristics. Human Science does not utilize crowdsourcing; instead, we advance projects with resources contracted directly. We organize teams that can demonstrate maximum performance based on a solid understanding of each member's practical experience and evaluations from previous projects.
4-3. Complete Security Room in Our Company
Human Science has a security room that meets ISMS standards within our Shinjuku office. We can handle even highly confidential projects on-site. We consider the assurance of confidentiality to be extremely important for any project. Our staff undergoes continuous security training, and we exercise the utmost care in handling information and data, even for remote projects.
4-4. Achievements in Creating 48 Million Teacher Data Entries
If your company wants to implement AI but doesn't know where to start, please consult Human Science. At Human Science, we participate in AI development projects across various industries, including natural language processing, medical support, automotive, IT, manufacturing, and construction. Through direct transactions with many companies, including GAFAM, we have provided over 48 million high-quality training data. We handle a variety of annotation projects, from small-scale projects to long-term large-scale projects with 150 annotators, regardless of the industry.