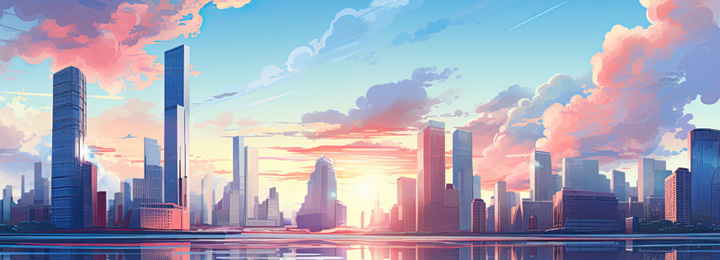
The technology of AI has developed remarkably in recent years, and its application is advancing even in areas that were previously considered difficult. AI is widely permeated in society, but the machine learning technology that supports this development requires a vast amount of data. Moreover, in order for AI to accurately achieve its objectives through learning, this data must be prepared through human manual work (annotation). These are referred to as "training data" and "labeled data." In this article, we will explain the differences between these "training data" and "labeled data."
- Table of Contents
-
- 1. What is AI machine learning?
- 1-1. What is AI?
- 1-2. What is Machine Learning?
- 2. What is training data?
- 3. The Difference Between Training Data and Learning Data
- 3-1. What is training data?
- 3-2. Differences Between Learning Data and Training Data
- 4. Flow of AI Development
- 5. Points to Consider When Collecting Training Data
- 6. Summary
- 7. Human Science Annotation Agency Services
1. What is AI machine learning?
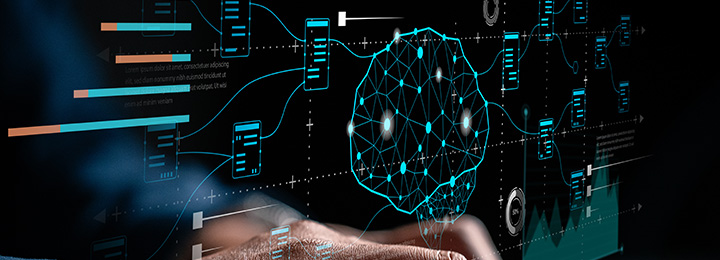
Here, to understand the difference between training data and learning data in AI machine learning, we will first explain AI and machine learning.
1-1. What is AI?
AI stands for Artificial Intelligence. It is a technology aimed at reproducing human recognition, thinking, and creative abilities in machines, allowing them to operate autonomously, with its origins dating back to the 1950s.
1-2. What is Machine Learning?
Machine learning is one of the technologies of AI. By allowing machines to learn (train) the characteristics of objects within data, they can automatically detect those objects. In recent years, the field of machine learning has seen the emergence of a technology called deep learning, which uses algorithms modeled after human neurons. Due to its high recognition accuracy and wide range of applications, the third AI boom has arrived. On the other hand, there are also technologies outside of machine learning, such as knowledge processing techniques, planning techniques, and matching techniques, which have been utilized since before the boom.
2. What is teacher data?
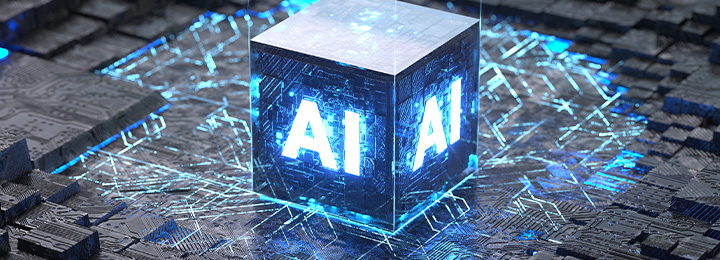
There are three learning methods in machine learning: "supervised learning," "unsupervised learning," and "reinforcement learning." All learning methods require a vast amount of data, but the data prepared for each method differs. In "supervised learning," data known as training data is required.
In supervised learning, for AI to recognize specific objects from data through learning, it is necessary to indicate the objects within the data. For example, to recognize Mount Fuji from an image, it means marking the data with "This image is Mount Fuji." The process of marking data in this way is called annotation. By providing the AI with these annotated images, it learns for the first time that "This image is Mount Fuji." The annotated data is referred to as training data.
3. Difference Between Teacher Data and Learning Data
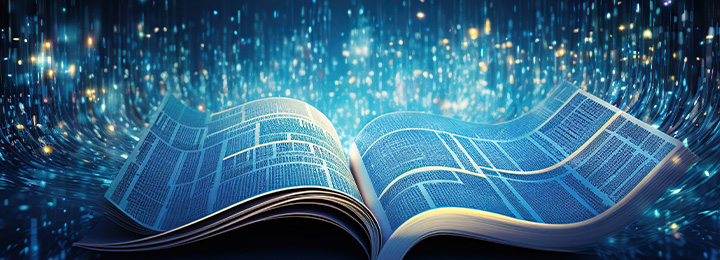
In machine learning, data referred to as training data is used in addition to labeled data. While the two may seem similar at first glance, there are differences.
3-1. What is training data?
Training data is a dataset used for AI to learn recognition targets. In "supervised learning," the training data includes labeled data. In "unsupervised learning" and "reinforcement learning," it does not include labeled data. In other words, any data used for learning in machine learning is referred to as training data.
3-2. Differences Between Learning Data and Training Data
As explained earlier, the training data is the data that has been annotated. It is essential in "supervised learning," but is not used in other methods. This is the difference between training data and labeled data.
4. Flow of AI Development
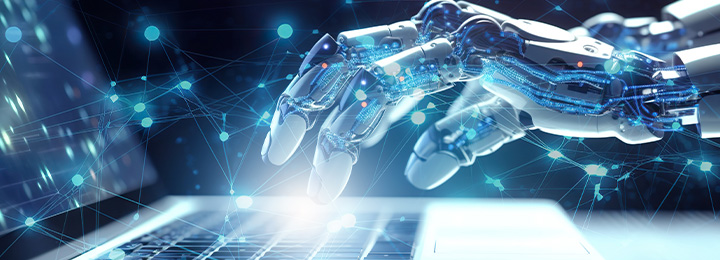
Here, we will focus on the part of the AI development process that involves learning from data.
: Data Collection
We collect data for learning purposes. We gather a large amount of data that includes the subjects we want to recognize. By preparing data under as many different conditions as possible, we prevent the occurrence of "overfitting," where recognition accuracy improves only under specific conditions. Various conditions, in the case of Mount Fuji mentioned earlier, refer to images with various variations such as different seasons, different weather, different angles, and different sizes.
: Annotation
Once the data collected is selected for creating the training data, a specification document will be created, and annotation work will be carried out based on it. Since it is necessary to create a large amount of data, we will assign the necessary number of annotators to manage quality and productivity, ensuring that there are no delays in the development schedule or impacts on AI learning due to quality issues.
: Learning
The completed training data is used to train the AI. The AI that has learned the correct patterns is called an inference model or a trained model.
: Model Evaluation
We evaluate whether the results output by the inference model, given learning data other than the training data, reach the desired accuracy.
: Implementation
Once it is confirmed that the AI can achieve sufficient accuracy, it will be implemented and operated on various devices and software. We will monitor to ensure that recognition accuracy does not decline, and perform additional training and maintenance as necessary.
5. Points to Consider When Collecting Training Data
When collecting data, it is necessary to be mindful of copyright and personal information. According to the document "About the Relationship Between AI and Copyright" published by the Ministry of Internal Affairs and Communications, under Article 30-4 of the current Copyright Act, it is stated that "in the case of information analysis such as AI development, acts of use that do not aim to enjoy the thoughts or feelings expressed in the work can generally be used without the permission of the copyright holder."
Citation:Regarding the relationship between AI and copyright
However, with the recent advancements in generative AI, there is a possibility that the output may closely resemble the original copyrighted work, which could unjustly infringe on the rights of the author. Even when using commercially available datasets, it is necessary to comply with the terms of use. Additionally, data that includes personal information, such as facial photographs obtained from street cameras, may infringe on privacy. Even if data is collected with the consent or permission of the individual, there have been cases where objections to its use arise later, leading to the discontinuation of development. Therefore, it is essential to exercise utmost caution in the collection and use of data.
6. Summary
We explained the difference between training data and learning data in machine learning. Among various machine learning methods, supervised learning is the most common approach, utilized with various types of data such as images, videos, text, and audio. For the success of AI development, it is crucial to have good learning data, especially high-quality training data. Although there are automated tools for data creation annotation, it is mostly done manually. Many companies may face challenges in progressing with development as they may have their own annotation processes but lack resources or management experience. In such cases, outsourcing can be a good option.
7. Human Science Annotation Agency Services
Rich track record of creating 48 million training data
Human Science participates in AI model development projects across various industries, including natural language processing, medical support, automotive, IT, manufacturing, and construction. Through direct transactions with many companies, including GAFAM, we have provided over 48 million high-quality training data. We handle a variety of annotation projects, from small-scale projects to long-term large-scale projects with 150 annotators, regardless of the industry. Companies that want to implement AI models but are unsure where to start are welcome to consult with us.
Resource Management Without Using Crowdsourcing
At Human Science, we do not utilize crowdsourcing; instead, we advance projects with personnel directly contracted by our company. We carefully assess each member's practical experience and their evaluations from previous projects to form a team that can deliver maximum performance.
Utilize the Latest Annotation Tools
One of the annotation tools introduced by Human Science, AnnoFab, allows customers to receive progress checks and feedback in the cloud even during the project's progress. By ensuring that work data cannot be saved on local machines, we also take security into consideration.
Fully Equipped Security Room On-Site
At Human Science, we have a security room that meets ISMS standards within our Shinjuku office. This allows us to handle even highly confidential projects on-site while ensuring security. We consider the protection of confidentiality to be extremely important for all projects. Our staff undergoes continuous security training, and we exercise the utmost care in handling information and data, even for remote projects.