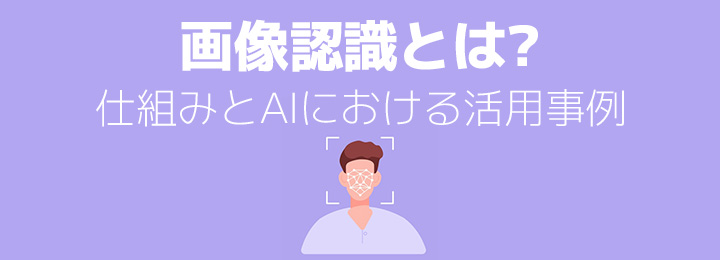
In recent years, AI image recognition technology has been active in various fields. While image recognition technology itself has been researched and put into practical use for a long time, the development of AI technology using deep learning has been remarkable in recent years, and products and services utilizing this technology are rapidly spreading in our daily lives. I often encounter situations where I am surprised to realize, 'Is this also using AI image recognition technology!' This time, I would like to explain what image recognition is, how it works, and how it is being utilized, using examples.
- Table of Contents
-
- 1. What is Image Recognition? The Mechanism of Image Recognition
- 1-1. What is image recognition?
- 1-2. Mechanism of Image Recognition (Machine Learning, Emergence of Deep Learning)
- 2. Types of Image Recognition
- 2-1. Image Classification
- 2-2. Object Detection
- 2-3. Segmentation (Region Detection)
- 2-4. Character Recognition
- 3. Use Cases of Image Recognition
- 4. Summary - Image Recognition AI Involved with Our Company
- 5. Human Science Annotation, LLM RAG Data Structuring Agency Service
- 5-1. Utilizing the latest data annotation tools
- 5-2. Achievements in Creating 48 Million Training Data
- 5-3. Resource Management Without Using Crowdsourcing
- 5-4. Complete Security Room within the Company
1. What is Image Recognition? The Mechanism of Image Recognition
1-1. What is Image Recognition?
Image recognition is, in simple terms, a technology that recognizes people and objects in images. Image recognition is a type of pattern recognition, and as mentioned at the beginning, in recent years, applications in various fields have advanced due to the technology of deep learning (deep learning).
The history of image recognition is quite old, with research progressing for 40 to 50 years, and it is said that barcode recognition is the first instance of image recognition as a familiar technology.
In recognizing objects within an image and determining what they are, humans rely on experience (for example, distinguishing between dogs and cats) to unconsciously identify various features of the objects. However, this is not the case for computers. Computers can only recognize images as a collection of pixels or at the pixel level. As a result, various research and initiatives have been undertaken. Template matching is one such example, where the image of the object to be recognized or detected is used as a template, allowing for the extraction of information such as "where the object is located in the image" and "how many of them are present" by comparing the similarity of the target image to the template.
However, even with this method, it was a technology that was difficult to put into practical use unless various conditions, such as the shooting conditions of the images, were managed rigorously, as the recognition rate would decrease significantly if there were large differences from the template images.
1-2. The Mechanism of Image Recognition (Machine Learning, The Emergence of Deep Learning)
Image recognition was a technology that was difficult to put into practical use, but the advent of machine learning and deep learning has changed the situation dramatically. Although machine learning has been an established technology for a long time, innovations such as improvements in computer processing speed have made it a realistically applicable technology that is now more accessible.
(We will omit a detailed explanation of how deep learning works here, but for more information, please see our blog below.)
https://www.science.co.jp/annotation_blog/30343/
Deep learning, as you may know, is an algorithm that uses neural networks modeled after human neurons, and it has increasingly been discussed as a representative technology supporting AI. This falls under the category of pattern recognition, where AI learns from labeled data known as training data (for example, data labeled as dog or cat for images of dogs and cats, respectively), allowing it to recognize the features of dogs and cats and identify them in images.
The more experience a person gains, the better they can identify confusing things; similarly, the more training data there is, the higher the accuracy of AI. In other words, having more data yields the same effect as a person gaining more experience.
The bottleneck here is the preparation of a large amount of training data. Naturally, preparing a large amount of training data requires a significant amount of labeling. (This labeling work is referred to as annotation.) Although automation has advanced considerably in recent years, the fact remains that training data is created to identify ambiguous items that computers cannot mechanically identify according to rules, so we still rely heavily on human effort. As a result, it inevitably becomes a manpower-intensive task, which incurs a considerable cost.
Needless to say, the quality of training data = the quality of annotations greatly affects the identification accuracy of AI. Additionally, since neural networks are structured to mimic the human brain, areas where humans are prone to make mistakes are similarly prone to errors by AI. However, when humans recognize things, their judgment can be dulled by the situation, physical condition, or emotions they are in, which can significantly impact identification accuracy; AI does not experience such issues. Moreover, the speed of identification is incomparable to that of humans, as it can identify and process information instantaneously. Therefore, for simple tasks that have ambiguity or lack discernible patterns, which have been difficult for machines to identify in the past, the automation brought by AI implementation is expected to have a significant impact, leading to an accelerated application in products and services in recent years.
2. Types of Image Recognition
So far, we have discussed image recognition and its mechanisms, but from here, we will introduce the representative types of image recognition: image classification, object detection, segmentation, and character recognition.
2-1. Image Classification
Image classification is a technology that classifies objects within images. It recognizes whether predefined objects are present in the image. For example, if dogs and cats are defined as the objects to be recognized, the task of image classification is to classify whether the objects in the image are either a dog or a cat. Unlike object detection, which will be discussed later, it does not detect the position of the objects.
Example 1: Scene Recognition
In scene recognition, the focus is not on recognizing specific objects within an image, but rather on recognizing the overall characteristics of the image. If image classification is the task of determining whether a specific tree is present in an image of a forest, then scene recognition is the task of determining whether the image depicts a forest.
Application Example 2: Anomaly Detection
In industries such as manufacturing and construction, there is a method for detecting anomalies in objects from images as an alternative to visual inspection. Since anomalies often occur infrequently, the method typically involves processing a large number of images to learn the normal values, and then detecting images that contain values that deviate from these (anomalous values).
Application Example 3: Face Recognition
Face recognition is a technology that extracts and recognizes prominent features from human facial images, as can be easily imagined from the words. It can be used for face identification and grouping faces. By using this technology, it is now possible to manage security with facial authentication and even identify the age groups of passengers in public transport or customers in stores.
2-2. Object Detection
Object detection is a technology for detecting the location of specific objects within an image. It is often confused with object recognition, but they are strictly different. Object recognition is a technology that verifies whether the same object as the target exists within the image, without specifically detecting its location. When these AI image recognition technologies are used in products and services, they are often used in conjunction.
Object detection and recognition technologies are used in an astonishingly wide range of fields, with alternative examples including their use in autonomous driving for identifying signs, pedestrians, and vehicles ahead.
Application Example: Image Caption Generation
Image caption generation is a technology that adds captions to the situations within images. While it shares similarities with scene recognition mentioned in the image classification section, it also requires the detection of individual objects in the image and recognition of their positions, necessitating object detection technology. Furthermore, it is essential to summarize the spatial relationships and situations of the objects and output them in natural language, which involves the use of natural language processing technology. Its application is expected to assist visually impaired individuals in spatial awareness.
2-3. Segmentation (Region Detection)
In object detection, it is possible to detect the position of objects within an image, but the shape and outline cannot be identified. In segmentation, learning is conducted to detect the outlines of specific objects, which is expected to be utilized in industries such as healthcare that require higher precision object detection, such as shape recognition.
What is segmentation? What can be achieved by utilizing AI segmentation?
2-4. Optical Character Recognition (OCR)
Optical Character Recognition (OCR) is a technology that identifies characters and symbols written on paper or in images. Since characters and symbols have a certain degree of regularity, this technology has been put to practical use for a long time, and recently, the accuracy of handwritten character recognition has also improved. Combined with machine translation technology, applications can now translate restaurant menus scanned with smartphone cameras, or automatically log receipts into household accounts by scanning them with a smartphone camera. Products and services utilizing this technology have permeated not only the business scene but also our everyday lives.
What is AI OCR? - 3 Examples of Its Differences from Traditional OCR and Use Cases
3. Use Cases of Image Recognition
Streamlining Construction Photo Management
In construction work, a vast number of construction photos are taken to understand the construction conditions and progress, and various management tasks using images and drawings arise, such as attaching these images to documents and blueprints. In response to the 2024 issue, it has become urgent to improve operational efficiency and productivity through construction DX, and by utilizing AI image recognition technology for the shooting and management of these construction photos, efficiency in operations is expected.
Sorting of recyclable waste
Industrial waste resource recycling requires accurate sorting of various types of recyclable materials such as PET bottles, steel cans, aluminum cans, and glass bottles. Traditionally, this was done manually, but due to the heavy labor and complexity involved, automation has become an urgent issue. While the sorting of PET bottles, steel cans, and aluminum cans has progressed in automation, glass bottles have not been automated due to the need for sorting by color. With the advancement of AI image recognition technology, color identification has become possible, which can also address the labor shortage.
Expansion of One-Man Operation Railway Lines
Railway companies are being required to implement one-man operation while promoting labor-saving and operational efficiency. So far, JR Tokai has adopted one-man operation with a maximum of two-car trains on some routes. By introducing a safety confirmation device incorporating image recognition AI, safety in one-man operation has been confirmed even on routes operating with four-car trains, making it possible to expand the routes for one-man operation.
JR Tokai to Expand One-Man Operation from FY 2025 Utilizing AI Image Recognition
4. Summary - Image Recognition AI Involved with Our Company
This time, we mainly discussed the mechanisms of image recognition and the types of image recognition. These AI technologies for image recognition are currently used in a wide variety of fields, and in the future, the areas of application will further expand, becoming more ingrained in people's lives. As if to prove this, the AI development requests we receive for our annotation services come from a truly diverse range of companies.
This is just one example, but below we introduce the AI image recognition technology for which Human Science has provided annotation services.
Case Studies
https://www.science.co.jp/annotation/experience/index.html
Industry-Specific Examples
● Medical Industry: Surgical Assistance, Diagnostic Support (Object Detection)
https://www.science.co.jp/annotation/industry/medical.html
● Automotive Industry: Autonomous Driving Project 2D/3D (Object Detection)
https://www.science.co.jp/annotation/industry/automobile.html
● IT Industry: Automatic Invoice Recognition (Optical Character Recognition)
https://www.science.co.jp/annotation/industry/it.html
As mentioned earlier, not only image recognition but also AI machine learning requires a large amount of training data, and therefore, annotation incurs a certain cost. If you want to reduce the cost of annotation, considering outsourcing or delegating the annotation work is one effective option. Our company offers a wide range of services from consultation on annotation, support for formulating annotation specifications, creating specification documents, to proposing annotation tools, so please feel free to reach out to us.
5. Human Science Annotation, LLM RAG Data Structuring Agency Service
5-1. Human Science Annotation, LLM RAG Data Structuring Agency Service
At Human Science, we are involved in AI model development projects across various industries, starting with natural language processing and extending to medical support, automotive, IT, manufacturing, and construction, just to name a few. Through direct business with many companies, including GAFAM, we have provided over 48 million pieces of high-quality training data. No matter the industry, our team of 150 annotators is prepared to accommodate various types of annotation, data labeling, and data structuring, from small-scale projects to big long-term projects.
5-2. Resource Management Without Using Crowdsourcing
At Human Science, we do not use crowdsourcing. Instead, projects are handled by personnel who are contracted with us directly. Based on a solid understanding of each member's practical experience and their evaluations from previous projects, we form teams that can deliver maximum performance.
5-3. Supports not only annotation but also the creation and structuring of generative AI LLM datasets.
In addition to labeling for data organization and annotation for identification-based AI systems, Human Science also supports the structuring of document data for generative AI and LLM RAG construction. Since our founding, our primary business has been in manual production, and we can leverage our deep knowledge of various document structures to provide you with optimal solutions.
5-4. Complete Security Room in Our Company
Within our Shinjuku office at Human Science, we have secure rooms that meet ISMS standards. Therefore, we can guarantee security, even for projects that include highly confidential data. We consider the preservation of confidentiality to be extremely important for all projects. When working remotely as well, our information security management system has received high praise from clients, because not only do we implement hardware measures, we continuously provide security training to our personnel.